mirror of
https://github.com/The-Art-of-Hacking/h4cker.git
synced 2025-02-22 14:43:41 +00:00
Delete ai_research/ML_Fundamentals/ai_generated directory
This commit is contained in:
parent
cef7e37769
commit
7bb7e64023
@ -1,2 +0,0 @@
|
||||
# AI Generated Content
|
||||
This section includes a series of articles that were generated with AI using several scripts that I will add here with time.
|
@ -1,50 +0,0 @@
|
||||
'''
|
||||
A simple test to use AI (OpenAI API) to generate an article based on a list of ideas.
|
||||
You can do this a lot better using LangChain. However, this is a simple example to demonstrate how to use the OpenAI API.
|
||||
Author: Omar Santos, os@cisco.com, @santosomar
|
||||
'''
|
||||
|
||||
# Import the required libraries
|
||||
# Install all the required libraries using pip install openai python-dotenv
|
||||
from dotenv import load_dotenv
|
||||
import openai
|
||||
import os
|
||||
import sys
|
||||
|
||||
# Load the .env file
|
||||
load_dotenv()
|
||||
|
||||
# Get the API key from the environment variable
|
||||
openai.api_key = os.getenv('OPENAI_API_KEY')
|
||||
|
||||
# Read the ideas from a file (ideas.txt)
|
||||
with open('ideas.txt', 'r') as file:
|
||||
lines = file.readlines()
|
||||
|
||||
# Read lines one by one
|
||||
for line in lines:
|
||||
# Create a filename
|
||||
filename = line.strip().replace(' ', '_') + '.md'
|
||||
idea = line.strip()
|
||||
|
||||
# Create a path to save the files in a specific directory
|
||||
filepath = os.path.join('data', filename)
|
||||
|
||||
# Prepare the prompt
|
||||
prompt = [{"role": "user", "content": f"Create an article about {idea}."}]
|
||||
# Generate the AI response using the GPT-3.5 model (16k)
|
||||
# https://beta.openai.com/docs/api-reference/create-completion
|
||||
response = openai.ChatCompletion.create(
|
||||
model="gpt-4o-mini",
|
||||
messages=prompt,
|
||||
max_tokens=16000
|
||||
)
|
||||
|
||||
# print the AI response
|
||||
final_response = response.choices[0].message.content
|
||||
|
||||
print(final_response)
|
||||
|
||||
# Create a new markdown file and write the article
|
||||
with open(filepath, 'w') as md_file:
|
||||
md_file.write(final_response)
|
@ -1,37 +0,0 @@
|
||||
# Actor-Critic Methods
|
||||
|
||||
**Actor-Critic methods** are a popular class of reinforcement learning algorithms that combine value-based methods (like Q-learning) with policy-based methods to solve sequential decision-making problems. They employ both an *actor* network to select actions and a *critic* network to evaluate the selected actions' quality.
|
||||
|
||||
## How Actor-Critic Methods Work
|
||||
|
||||
At a high level, actor-critic methods work by learning two different functions: the *actor* function, which maps states to actions, and the *critic* function, which estimates the value function or the action-value function.
|
||||
|
||||
The actor network is typically a deep neural network with the input as the current state and output as the action probabilities. It is responsible for selecting actions based on the current policy. In contrast, the critic network approximates the value function or action-value function and is used to evaluate the quality of the selected actions.
|
||||
|
||||
The actor network is updated based on the feedback received from the critic network. The critic network, in turn, is updated using the temporal-difference error signals obtained from the environment or using bootstrapping techniques like in TD-learning or Monte Carlo methods.
|
||||
|
||||
## Advantages of Actor-Critic Methods
|
||||
|
||||
1. **Improved Sample Efficiency:** By combining the strengths of value-based and policy-based methods, actor-critic algorithms often achieve improved sample efficiency compared to other reinforcement learning algorithms. They effectively leverage the information from both the value function and the policy to make more informed decisions.
|
||||
|
||||
2. **Addressing Exploration-Exploitation Tradeoff:** The actor-critic framework allows for a tradeoff between exploration and exploitation. The critic network guides the actor by providing valuable feedback on the quality of the current policy, helping to balance exploration and exploitation effectively.
|
||||
|
||||
3. **Suitable for Continuous Action Spaces:** Actor-critic methods are well-suited for environments with continuous action spaces. The actor network outputs probabilities for each possible action, enabling easy adaptation to different action requirements.
|
||||
|
||||
4. **Flexibility in Policy Representation:** Actor-critic methods allow for flexible policy representations, as the actor network can be easily designed using various policy structures such as deep neural networks or Gaussian processes.
|
||||
|
||||
## Popular Actor-Critic Algorithms
|
||||
|
||||
Several popular actor-critic algorithms have been developed, each with its own variations and improvements. Some of the well-known algorithms include:
|
||||
|
||||
1. **Advantage Actor-Critic (A2C):** A2C is a synchronous variant of the actor-critic algorithm that updates the actor and critic networks simultaneously based on the experiences collected from multiple agents.
|
||||
|
||||
2. **Asynchronous Advantage Actor-Critic (A3C):** A3C is an extension of A2C that handles multiple agents in an asynchronous manner. This architecture allows for parallelization during the learning process, resulting in faster convergence.
|
||||
|
||||
3. **Proximal Policy Optimization (PPO):** PPO is an actor-critic algorithm that uses a surrogate objective function to update the policy network. It ensures that policy updates maintain a similar policy distribution, preventing large policy changes during training.
|
||||
|
||||
4. **Deep Deterministic Policy Gradient (DDPG):** DDPG is an actor-critic algorithm specifically designed for continuous action spaces. It employs an actor network to approximate the optimal deterministic policy and a critic network to estimate the corresponding action-value function.
|
||||
|
||||
## Conclusion
|
||||
|
||||
Actor-critic methods offer a powerful framework for reinforcement learning, combining the strengths of value-based and policy-based methods. They have proven to be effective in various complex environments and have been widely used for solving challenging decision-making problems. With continuous improvements and variations of actor-critic algorithms, they continue to play a significant role in advancing the field of reinforcement learning.
|
@ -1,33 +0,0 @@
|
||||
# Association Rules: Apriori and FP-Growth
|
||||
|
||||
Association rule mining is a widely used technique in data mining to discover interesting relationships hidden in large datasets. It aims to find associations or correlations among items or events, often expressed in the form of "if X, then Y", where X and Y are known as itemsets. Two popular algorithms used for association rule mining are Apriori and FP-Growth.
|
||||
|
||||
## Apriori Algorithm
|
||||
|
||||
Apriori is an algorithm that identifies frequent itemsets in a dataset and uses them to generate association rules. It follows the "bottom-up" approach, where frequent itemsets of size k are used to explore frequent itemsets of size k+1. The basic idea behind the Apriori principle is that if an itemset is infrequent, then its supersets must also be infrequent.
|
||||
|
||||
The Apriori algorithm consists of two main steps:
|
||||
|
||||
1. **Generating frequent itemsets:** In this step, the algorithm scans the dataset to identify the frequent itemsets that satisfy the minimum support threshold specified by the user. Initially, it starts with individual items as the frequent itemsets, and then iteratively generates larger itemsets.
|
||||
|
||||
2. **Generating association rules:** Once the frequent itemsets are identified, the algorithm generates association rules from these itemsets. It calculates the confidence measure for each association rule and filters out the ones that do not meet the minimum confidence threshold set by the user.
|
||||
|
||||
Apriori has the advantage of being simple and easy to implement. However, it suffers from inefficient execution, especially when dealing with large datasets, due to the large number of candidate itemsets generated.
|
||||
|
||||
## FP-Growth Algorithm
|
||||
|
||||
FP-Growth (Frequent Pattern-Growth) is another popular algorithm used for mining association rules. It addresses the limitations of the Apriori algorithm by using a different approach. FP-Growth avoids generating the candidate itemsets and instead builds a compact data structure called an FP-tree.
|
||||
|
||||
The FP-Growth algorithm consists of two main steps:
|
||||
|
||||
1. **Building the FP-tree:** In this step, the algorithm scans the dataset to construct an FP-tree, which represents the frequent itemsets and their support information. The FP-tree is built incrementally using a series of transactions from the dataset.
|
||||
|
||||
2. **Mining the FP-tree for association rules:** Once the FP-tree is constructed, the algorithm performs a recursive mining process on the tree to find the frequent itemsets and generate the association rules. The mining process utilizes a technique called recursive projection, which efficiently explores the patterns in the FP-tree.
|
||||
|
||||
FP-Growth has several advantages over the Apriori algorithm. It does not require multiple scans of the dataset, as it constructs the FP-tree in a single pass. Additionally, it avoids the generation of candidate itemsets, leading to improved performance on large datasets.
|
||||
|
||||
## Conclusion
|
||||
|
||||
Association rule mining using algorithms like Apriori and FP-Growth is a powerful technique for discovering meaningful relationships and patterns in large datasets. While both algorithms have their strengths and weaknesses, they provide valuable insights that can be used for various applications, such as market basket analysis, recommendation systems, and fraud detection.
|
||||
|
||||
Whether you choose the simplicity of the Apriori algorithm or the efficiency of the FP-Growth algorithm depends on the specific requirements of your dataset and the desired performance trade-offs. Understanding these algorithms and their differences can help you make informed decisions and extract valuable knowledge from your data.
|
@ -1,42 +0,0 @@
|
||||
# What is DBSCAN?
|
||||
|
||||
DBSCAN (Density-Based Spatial Clustering of Applications with Noise) is a popular clustering algorithm used in data mining and machine learning. It was proposed by Martin Ester, Hans-Peter Kriegel, Jörg Sander, and Xiaowei Xu in 1996. DBSCAN is particularly useful for discovering clusters in large spatial databases with noise and irregularly shaped clusters.
|
||||
|
||||
## How does DBSCAN work?
|
||||
|
||||
DBSCAN groups data points that are close to each other based on two parameters: ε (Epsilon) and MinPts.
|
||||
|
||||
- Epsilon (ε) defines the radius within which the algorithm looks for neighboring data points. If the distance between two points is less than ε, they are considered neighbors.
|
||||
- MinPts specifies the minimum number of neighbors a data point should have within a distance ε to be considered a core point.
|
||||
|
||||
The algorithm proceeds as follows:
|
||||
1. Randomly choose an unvisited data point.
|
||||
2. Check if the point has at least MinPts neighbors within a distance ε. If yes, mark the point as a core point and create a new cluster.
|
||||
3. Expand the cluster by adding all directly reachable neighbors to the cluster. To achieve this, the algorithm recursively checks the neighbors of each core point to determine if they also have MinPts neighbors within ε. If a point is reachable, it is added to the cluster.
|
||||
4. Repeat steps 2 and 3 until no more points can be added to the current cluster.
|
||||
5. Find the next unvisited data point and repeat the process until all data points have been visited.
|
||||
|
||||
DBSCAN classifies data points into three categories:
|
||||
- Core points: Points that have at least MinPts neighbors within ε.
|
||||
- Border points: Points that have fewer than MinPts neighbors within ε but are within the ε radius of a core point.
|
||||
- Noise points: Points that are neither core nor border points.
|
||||
|
||||
## Advantages
|
||||
|
||||
DBSCAN has several advantages over traditional clustering algorithms like k-means:
|
||||
- DBSCAN can discover clusters of various shapes and sizes because it does not assume any specific cluster shape.
|
||||
- It can handle noisy data points effectively by identifying them as noise.
|
||||
- The algorithm does not require the number of clusters to be pre-specified, making it suitable for exploratory data analysis.
|
||||
- Once the clusters are identified, DBSCAN does not require iterative optimization steps, making it computationally efficient for large datasets.
|
||||
|
||||
## Limitations
|
||||
|
||||
While DBSCAN is a powerful clustering algorithm, it also has some limitations:
|
||||
- Choosing appropriate values for ε and MinPts can be challenging. Setting them too low may result in multiple small clusters, while setting them too high may merge distinct clusters.
|
||||
- DBSCAN struggles with high-dimensional data due to the curse of dimensionality. As the number of dimensions increases, the density becomes more scattered, making it difficult for the algorithm to distinguish between noise and clusters.
|
||||
- The algorithm may still struggle with datasets where clusters have varying densities.
|
||||
- DBSCAN cannot determine the optimal number of clusters automatically.
|
||||
|
||||
## Conclusion
|
||||
|
||||
DBSCAN is a density-based clustering algorithm that offers flexibility in identifying clusters of different shapes and sizes without requiring the number of clusters to be predefined. It is particularly useful for large spatial databases with irregularly shaped clusters and noisy data points. However, choosing appropriate parameter values and handling high-dimensional data remain challenges. Nonetheless, DBSCAN is a valuable tool in the realm of exploratory data analysis and pattern recognition.
|
@ -1,69 +0,0 @@
|
||||
# Decision Trees: Understanding the Basics
|
||||
|
||||

|
||||
|
||||
Decision Trees are powerful yet intuitive machine learning models that have gained popularity for their ability to solve both classification and regression problems. They play a crucial role in predictive analytics and have a wide range of applications in various industries, such as finance, healthcare, and marketing.
|
||||
|
||||
## Introduction to Decision Trees
|
||||
|
||||
At its core, a Decision Tree is a flowchart-like structure that breaks down a dataset into smaller and smaller subsets based on various attributes or features. It is a tree-like model where each internal node represents a feature, each branch represents a decision rule, and each leaf node represents an outcome.
|
||||
|
||||
Decision Trees are built using a series of splitting rules based on statistical metrics to maximize information gain or minimize impurity in the resulting subsets. These splitting rules divide the dataset based on feature values, creating branches or sub-trees, ultimately leading to the prediction or classification of a target variable.
|
||||
|
||||
## Key Components of a Decision Tree
|
||||
|
||||
### Root Node
|
||||
|
||||
The root node is the starting point of a decision tree, representing the entire dataset. It usually contains the most significant feature that best splits the data based on the specified criterion.
|
||||
|
||||
### Internal Nodes
|
||||
|
||||
Internal nodes represent test conditions or features used for splitting the data. Each internal node has branches corresponding to the possible outcomes of that feature.
|
||||
|
||||
### Leaf Nodes
|
||||
|
||||
Leaf nodes are the end-points of a decision tree, representing the final prediction or classification. They contain the target variable or the class label associated with the subset of data in that leaf.
|
||||
|
||||
### Splitting Criteria
|
||||
|
||||
Splitting criteria are statistical metrics used to measure the quality of a split or the homogeneity of the resulting subsets. Some popular splitting criteria include Gini Impurity and Information Gain.
|
||||
|
||||
### Pruning
|
||||
|
||||
Pruning is a technique used to simplify a decision tree by removing unnecessary branches or sub-trees. It helps prevent overfitting and improves the model's generalization ability.
|
||||
|
||||
## Advantages of Decision Trees
|
||||
|
||||
### Interpretability
|
||||
|
||||
Decision Trees are highly interpretable compared to other machine learning models. The flowchart-like structure allows us to trace the decision-making process for each observation.
|
||||
|
||||
### Handling Non-linear Relationships
|
||||
|
||||
Decision Trees can handle both linear and non-linear relationships between features and target variables. They can capture complex patterns that may be missed by other models.
|
||||
|
||||
### Feature Importance
|
||||
|
||||
Decision Trees provide insights into the importance of different features in predicting the target variable. This information can be used for feature selection and feature engineering.
|
||||
|
||||
### Robustness to Outliers and Missing Values
|
||||
|
||||
Decision Trees are relatively robust to outliers and missing values in the dataset. They can handle these situations effectively by splitting the data based on available feature values.
|
||||
|
||||
## Limitations of Decision Trees
|
||||
|
||||
### Overfitting
|
||||
|
||||
Decision Trees tend to create complex and deep trees that may overfit the training data. Pruning techniques can be applied to overcome this problem.
|
||||
|
||||
### Lack of Continuity
|
||||
|
||||
Decision Trees are not suitable for datasets with continuous features as they only support discrete or categorical features. Preprocessing techniques like binning can be used to convert continuous features into discrete ones.
|
||||
|
||||
### Instability
|
||||
|
||||
Decision Trees are sensitive to small changes in the data. A slight modification in the dataset can lead to a completely different tree structure, which might affect the model's performance.
|
||||
|
||||
## Conclusion
|
||||
|
||||
Decision Trees are valuable tools in machine learning, allowing us to make informed decisions and predictions based on data. They offer simplicity, interpretability, and flexibility while handling various types of problems. Understanding their components, advantages, and limitations is crucial for effectively utilizing Decision Trees in real-world applications.
|
@ -1,37 +0,0 @@
|
||||
# Gaussian Mixture Models (GMM)
|
||||
|
||||
## Introduction
|
||||
|
||||
Gaussian Mixture Models (GMM) is a powerful and widely used technique for modeling complex data distributions. It is a probabilistic model that represents the data as a mixture of Gaussian distributions. GMMs are particularly useful when dealing with data that does not fit into a single normal distribution.
|
||||
|
||||
## Basics of Gaussian Mixture Models
|
||||
|
||||
A Gaussian Mixture Model represents the distribution of the data as a weighted sum of multiple Gaussian distributions. Each Gaussian distribution, also known as a component, represents a distinct cluster in the data. GMM assumes that the data points are generated from one of these Gaussian components, and the task is to estimate the parameters of the mixture model to best fit the observed data.
|
||||
|
||||
The parameters of a GMM include the mean, covariance, and weight of each Gaussian component. The mean represents the center of each cluster, the covariance describes the shape of the distribution, and the weight determines the relative importance of each component in the mixture. GMM is commonly used for clustering, density estimation, and outlier detection.
|
||||
|
||||
## Estimating GMM Parameters
|
||||
|
||||
There are several methods for estimating the parameters of a GMM:
|
||||
|
||||
1. **Expectation-Maximization (EM) Algorithm:** The EM algorithm is the most commonly used method for fitting GMMs. It is an iterative algorithm that alternates between the expectation step (E-step), where the expected value of the latent variables (cluster assignments) is computed given the current parameters, and the maximization step (M-step), where the parameters are updated using the newly computed expectations.
|
||||
|
||||
2. **Maximum Likelihood Estimation (MLE):** MLE is another popular method for estimating GMM parameters. It involves finding the parameters that maximize the likelihood of observing the given data. The MLE estimator can be obtained by solving a set of nonlinear equations.
|
||||
|
||||
3. **Bayesian Inference:** Bayesian methods can also be used to estimate the parameters of a GMM. By incorporating prior knowledge about the parameters, Bayesian inference provides a way to update the prior beliefs based on the observed data, resulting in a posterior distribution over the parameters.
|
||||
|
||||
## Applications of Gaussian Mixture Models
|
||||
|
||||
GMMs have a wide range of applications in various domains:
|
||||
|
||||
1. **Image Segmentation:** GMMs can be applied to segment images into different regions based on color or texture information. Each region can be modeled by a separate Gaussian component, allowing for accurate segmentation of complex scenes.
|
||||
|
||||
2. **Speech Recognition:** GMMs are commonly used in speech recognition systems to model the distribution of phonemes or speech units. GMMs can capture the statistical variations in speech, enabling accurate recognition and transcription.
|
||||
|
||||
3. **Anomaly Detection:** GMMs can be used to detect anomalies or outliers in data. By modeling the normal data distribution, any data point that deviates significantly from the GMM is considered an anomaly, making GMMs useful for fraud detection or anomaly detection in various domains.
|
||||
|
||||
4. **Data Clustering:** GMMs are widely used for clustering tasks. Each Gaussian component represents a cluster, and the mixture model can assign data points to their most likely cluster based on the model's parameters. GMMs can handle non-linear and overlapping clusters, making them suitable for complex clustering problems.
|
||||
|
||||
## Conclusion
|
||||
|
||||
Gaussian Mixture Models provide a flexible and powerful framework for modeling complex data distributions. With their ability to capture multi-modal and non-linear patterns, GMMs have applications in various domains including image segmentation, speech recognition, anomaly detection, and data clustering. Understanding and utilizing GMMs can greatly enhance our ability to analyze and understand complex datasets.
|
@ -1,45 +0,0 @@
|
||||
# Gradient Boosting Machines (GBM)
|
||||
|
||||
Gradient Boosting Machines (GBM) are a powerful machine learning algorithm used for both regression and classification tasks. It is an ensemble method that combines multiple weak predictive models to create a strong model.
|
||||
|
||||
## How GBM Works
|
||||
|
||||
GBM builds the predictive model in a stage-wise manner, where each stage improves the model's performance by minimizing the loss function. The algorithm uses a gradient descent approach to optimize the loss function.
|
||||
|
||||
1. **Initialization:** GBM starts with an initial model, typically a constant value prediction for regression or the log odds for classification.
|
||||
2. **Stage-wise Learning:** At each stage, GBM fits the model to the negative gradient of the loss function, which is the residual error from the previous stage.
|
||||
3. **Adding New Model:** GBM adds a new model to the ensemble by adjusting the model's parameters to minimize the loss function. The new model is chosen based on the negative gradient direction that reduces the loss.
|
||||
4. **Weight Update:** GBM calculates the weights of the new model ensemble by finding the optimal step size produced by line search or grid search.
|
||||
5. **Repeat:** Steps 3 and 4 are repeated until a stopping criterion is met, such as reaching a specific number of models or achieving a certain improvement in the loss function.
|
||||
|
||||
## Advantages of GBM
|
||||
|
||||
GBM offers several advantages, making it popular among data scientists and machine learning practitioners:
|
||||
|
||||
1. **Flexibility:** GBM can handle a variety of data types, including both numerical and categorical features.
|
||||
2. **Feature Importance:** GBM provides a measure of feature importance, allowing analysts to identify which variables are most influential in making predictions.
|
||||
3. **Robustness to Outliers:** GBM can handle outliers effectively by using robust loss functions or robust optimization algorithms.
|
||||
4. **Handles Missing Values:** GBM can handle missing values in the dataset and still produce accurate predictions.
|
||||
5. **Higher Accuracy:** GBM often achieves better predictive accuracy compared to other machine learning algorithms due to its ensemble nature.
|
||||
|
||||
## Limitations of GBM
|
||||
|
||||
While GBM is a powerful algorithm, it also has some limitations:
|
||||
|
||||
1. **Computational Complexity:** GBM can be computationally expensive since it builds models sequentially, requiring more computational resources and time.
|
||||
2. **Overfitting:** If not carefully regularized, GBM models can overfit the training data and perform poorly on unseen data.
|
||||
3. **Hyperparameter Tuning:** GBM involves tuning multiple hyperparameters, which can be a manual and tedious process.
|
||||
4. **Lack of Interpretability:** The ensemble nature of GBM makes it difficult to interpret and understand the individual contributions of each feature.
|
||||
|
||||
## Applications of GBM
|
||||
|
||||
GBM has been successfully applied in various domains, including:
|
||||
|
||||
1. **Finance:** GBM is widely used in predicting stock prices, credit risk modeling, and fraud detection.
|
||||
2. **Healthcare:** GBM has been applied to predict diseases, identify patterns in genomic data, and predict patient outcomes.
|
||||
3. **Marketing:** GBM is used for customer segmentation, churn prediction, and targeted marketing campaigns.
|
||||
4. **Recommendation Systems:** GBM can be utilized to develop personalized recommendation systems based on user preferences and behavior.
|
||||
|
||||
## Conclusion
|
||||
|
||||
Gradient Boosting Machines (GBM) provide a powerful and flexible approach for predictive modeling. By combining weak models in an ensemble using a stage-wise learning approach, GBM achieves high accuracy and handles complex datasets. While it has some limitations, GBM remains a popular choice among data scientists for various machine learning tasks.
|
@ -1,45 +0,0 @@
|
||||
# Independent Component Analysis (ICA)
|
||||
|
||||
Independent Component Analysis (ICA) is a statistical technique used to reveal hidden factors or independent components in multivariate data. It aims to decompose a set of mixed signals into their respective sources, assuming that the observed signals are linear mixtures of non-Gaussian source signals. ICA has applications in various fields including signal processing, blind source separation, image processing, and machine learning.
|
||||
|
||||
## How does ICA work?
|
||||
|
||||
ICA is based on the assumption that the observed signals are linear combinations of statistically independent source signals. The goal is to recover the original independent components by separating the mixed observed signals.
|
||||
|
||||
The process of ICA involves the following steps:
|
||||
|
||||
1. **Preprocessing:** Before applying ICA, it is essential to preprocess the data by centering it to have zero mean and decorrelating the signals to remove any linear dependencies.
|
||||
|
||||
2. **Statistical independence estimation:** ICA aims to estimate the statistical independence between the observed signals. It achieves this by maximizing the non-Gaussianity of the estimated components.
|
||||
|
||||
3. **Signal separation:** Once the independence estimation is obtained, ICA decomposes the mixed signals into their respective independent components. This separation is achieved through a matrix transformation that maximizes the statistical independence of the estimated sources.
|
||||
|
||||
4. **Component reconstruction:** After the signal separation, the independent components can be reconstructed by multiplying the estimated sources with the mixing matrix.
|
||||
|
||||
## Advantages of ICA
|
||||
|
||||
ICA offers several advantages in different fields:
|
||||
|
||||
1. **Signal separation:** ICA has been widely used for blind source separation, which involves the separation of mixed signals without any prior knowledge about the mixing process. This makes ICA a powerful tool in separating audio signals, EEG (electroencephalography) signals, and other types of mixed data.
|
||||
|
||||
2. **Feature extraction:** ICA can be used to extract meaningful features from complex data. By decomposing the mixed signals into their independent components, it becomes easier to identify and analyze the essential underlying factors in the data.
|
||||
|
||||
3. **Noise reduction:** In image processing, ICA can effectively remove noise and artifacts from images. By separating the signal sources, it becomes possible to distinguish between the signal of interest and the noise or background interference.
|
||||
|
||||
4. **Dimensionality reduction:** ICA can also be applied as a dimensionality reduction technique. By extracting the most important independent components, it helps reduce the dimensionality of the data while retaining the essential information.
|
||||
|
||||
## Limitations of ICA
|
||||
|
||||
While ICA is a powerful technique, it also has some limitations:
|
||||
|
||||
1. **Assumption of linearity:** ICA assumes that the observed signals are a linear mixture of the independent sources. In some cases, this linearity assumption may not hold, leading to inaccurate results.
|
||||
|
||||
2. **Number of sources estimation:** Estimating the correct number of independent sources can be challenging. Choosing an incorrect number of sources may lead to incomplete or incorrect separation.
|
||||
|
||||
3. **Sensitive to signal scaling:** ICA is sensitive to the scaling of the signals. If the scaling is not consistent, the estimated independent components may be distorted.
|
||||
|
||||
4. **Computationally intensive:** Performing ICA on large datasets can be computationally intensive, requiring significant computational resources and time.
|
||||
|
||||
## Conclusion
|
||||
|
||||
Independent Component Analysis (ICA) is a powerful statistical technique used to extract hidden factors or independent components from mixed signals. It has applications in various fields and offers advantages such as signal separation, feature extraction, noise reduction, and dimensionality reduction. However, it is important to consider its limitations and potential constraints when applying ICA for specific tasks. Overall, ICA provides valuable insights into the underlying structure of multidimensional data, enabling a better understanding and analysis of complex information.
|
@ -1,51 +0,0 @@
|
||||
## Latent Dirichlet Allocation (LDA)
|
||||
|
||||
Latent Dirichlet Allocation (LDA) is a probabilistic model used to group documents based on the topics they contain. It is widely used in the field of natural language processing and has applications in information retrieval, text mining, and recommendation systems.
|
||||
|
||||
LDA assumes that each document in a corpus is a mixture of several topics, and each topic is a distribution of words. It aims to discover these latent topics and their corresponding word distributions by analyzing the words in the documents.
|
||||
|
||||
### How LDA works
|
||||
|
||||
LDA follows a generative process to allocate topics to documents and words to topics. Here are the primary steps involved:
|
||||
|
||||
1. **Initialization**: Initialize the number of topics, the number of words per topic, and the document-topic and topic-word probability distributions.
|
||||
|
||||
2. **Document-topic allocation**: Iterate through each document and randomly assign a topic to each word in the document according to the document-topic distribution.
|
||||
|
||||
3. **Word-topic allocation**: Iterate through each word and assign a topic to it according to the word-topic distribution and the topic assigned to its document.
|
||||
|
||||
4. **Updating probabilities**: Repeat steps 2 and 3 multiple times, updating the document-topic and topic-word probability distributions based on the assigned topics.
|
||||
|
||||
5. **Inference**: After a sufficient number of iterations, the final probability distributions represent the latent topics and word distributions. These can be used to assign topics to new documents or extract keywords from existing documents.
|
||||
|
||||
### Benefits of LDA
|
||||
|
||||
LDA provides several benefits and applications in various fields:
|
||||
|
||||
* **Topic modeling**: LDA allows researchers to uncover hidden topics in a corpus of documents, helping in organizing and understanding large volumes of textual data.
|
||||
|
||||
* **Information retrieval**: LDA helps improve search engine performance by identifying the most relevant documents based on user queries.
|
||||
|
||||
* **Text summarization**: LDA can be used for automatic text summarization, generating concise summaries of lengthy documents.
|
||||
|
||||
* **Recommendation systems**: LDA can be used to recommend relevant content to users based on their interests, by identifying the topics they are likely to be interested in.
|
||||
|
||||
* **Market research**: LDA enables analysis of customer feedback, social media posts, and online reviews, helping businesses understand customer preferences, sentiments, and trends.
|
||||
|
||||
### Limitations and Challenges
|
||||
|
||||
While LDA is a powerful technique, it is not without limitations:
|
||||
|
||||
* **Choice of topics**: Determining the optimal number of topics is challenging and subjective. An incorrect number of topics may result in less meaningful or overlapping topic distributions.
|
||||
|
||||
* **Sparsity**: Documents with very few words may produce unreliable topic allocations due to insufficient evidence.
|
||||
|
||||
* **Order sensitivity**: LDA is order sensitive, meaning that the order of words within a document may affect the inferred topics. Preprocessing and careful consideration of input order are necessary.
|
||||
|
||||
* **Domain-specific training**: Training an LDA model on one domain may not generalize well to another domain due to varying terminologies and word distributions.
|
||||
|
||||
* **Efficiency**: LDA can be computationally expensive, especially with large corpora. Advanced techniques such as parallelization and approximate inference can help alleviate this issue.
|
||||
|
||||
### Conclusion
|
||||
|
||||
Latent Dirichlet Allocation (LDA) is a valuable tool for discovering latent topics in a collection of documents. It has paved the way for various applications, including information retrieval, text summarization, and recommendation systems. However, careful consideration of model parameters, input order, and computational efficiency is required to obtain accurate and meaningful results. With continued research and advancements, LDA is expected to enhance our understanding of textual data and improve related applications.
|
@ -1,64 +0,0 @@
|
||||
# Monte Carlo Tree Search (MCTS)
|
||||
|
||||
Monte Carlo Tree Search (MCTS) is a popular algorithm used in decision processes within the domain of artificial intelligence and game theory. It is widely employed in scenarios where there is uncertainty and a need for efficient decision-making in large search spaces. MCTS combines randomized simulations with a tree-based search to gradually build an optimal decision tree, making it particularly effective for complex problems with vast solution spaces.
|
||||
|
||||
|
||||
## Background
|
||||
|
||||
MCTS was first introduced in 2006 by Rémi Coulom and made considerable advancements in the field of game-playing algorithms. Unlike conventional search algorithms, MCTS does not require a complete knowledge of the search space or any heuristics, while still yielding strong results.
|
||||
|
||||
The algorithm has been successfully applied to various problems, ranging from classic board games such as chess and Go, to real-world applications like robot motion planning, logistics optimization, and resource allocation problems.
|
||||
|
||||
|
||||
## Key Components
|
||||
|
||||
MCTS consists of four key components:
|
||||
|
||||
### 1. Selection
|
||||
|
||||
Starting at the root node, the algorithm traverses the decision tree based on certain criteria, typically the selection of the node that maximizes the UCT (Upper Confidence Bound applied to Trees) formula. This formula balances exploration and exploitation, favoring exploration of less visited areas initially, then shifting towards exploitation of promising paths as the search progresses.
|
||||
|
||||
### 2. Expansion
|
||||
|
||||
Once a leaf node is reached, the algorithm expands it by adding child nodes according to the available actions. Each child node represents a possible move or state transition from the current node.
|
||||
|
||||
### 3. Simulation (Rollout)
|
||||
|
||||
To evaluate the potential of a particular child node, MCTS performs a random playout from that node until reaching a terminal state. This simulation step accounts for the uncertainty in the decision-making process and aids in estimating the value of the node.
|
||||
|
||||
### 4. Backpropagation
|
||||
|
||||
After the simulation, the results are backpropagated up the tree, updating the statistics of each visited node. This information propagation step helps refine the UCT values of nodes, enabling the algorithm to make more informed decisions in subsequent iterations.
|
||||
|
||||
|
||||
## Advantages of MCTS
|
||||
|
||||
MCTS offers several advantages over traditional approaches to decision-making:
|
||||
|
||||
1. **Simplicity**: MCTS is relatively easy to understand and implement, as it does not require any domain-specific knowledge or heuristics.
|
||||
|
||||
2. **Ability to handle large search spaces**: MCTS is particularly effective in domains with enormous search spaces, where it outperforms traditional search algorithms by focusing its efforts on promising regions of the search tree.
|
||||
|
||||
3. **Flexibility**: MCTS is versatile and can be adapted to different problem domains and situations.
|
||||
|
||||
4. **Progressive refinement**: Unlike traditional algorithms that require complete evaluation of the entire search space, MCTS progressively improves its decision-making capabilities with each iteration, incorporating new knowledge into its search tree.
|
||||
|
||||
5. **Uncertainty handling**: By incorporating random simulations, MCTS is able to handle problems with uncertainty, making it suitable for domains with incomplete or imperfect information.
|
||||
|
||||
|
||||
## Limitations and Challenges
|
||||
|
||||
While MCTS has proven to be a powerful algorithm, it also has some limitations:
|
||||
|
||||
1. **Computationally expensive**: MCTS can require a significant amount of computational resources, especially in large and complex search spaces. The trade-off is often between exploration and efficiency.
|
||||
|
||||
2. **Parameter tuning**: Fine-tuning the MCTS algorithm to different problem domains is a non-trivial task, requiring experimentation and domain-specific knowledge.
|
||||
|
||||
3. **Knowledge representation**: MCTS may face challenges in domains where explicit representation of states and actions is complex or not well-defined.
|
||||
|
||||
4. **Incomplete knowledge**: MCTS assumes that all possible actions are known, which may not always be the case in some domains.
|
||||
|
||||
|
||||
## Conclusion
|
||||
|
||||
Monte Carlo Tree Search (MCTS) has emerged as a powerful algorithm for decision-making under uncertainty in a wide range of complex domains. It combines elements of random sampling with a tree-based search to gradually build an optimal decision tree. MCTS offers simplicity, flexibility, and the ability to handle large search spaces, making it well-suited for various real-world applications. However, it also has limitations, including computational expense and the need for parameter tuning. Overall, MCTS continues to be an integral part of the modern AI toolkit, paving the way for advancements in areas where uncertainty and complex decision processes exist.
|
@ -1,56 +0,0 @@
|
||||
# Naïve Bayes
|
||||
|
||||
Naïve Bayes is a probabilistic machine learning algorithm commonly used for classification tasks. It is based on Bayes' theorem, which provides a way to calculate the probability of a hypothesis given evidence.
|
||||
|
||||
## Introduction to Naïve Bayes
|
||||
|
||||
Naïve Bayes is a simple and effective classification algorithm, particularly well-suited for text classification problems such as spam filtering, sentiment analysis, and document categorization. It makes a strong assumption of independence between the features in the dataset, hence the term "naïve." Although this assumption might not hold true in all scenarios, Naïve Bayes still performs impressively well in many cases.
|
||||
|
||||
## How Does Naïve Bayes Work?
|
||||
|
||||
Naïve Bayes works by calculating the probability of each class given the input features and selecting the class with the highest probability as the final prediction. The algorithm assumes that each input feature is independent of the others, simplifying the calculations significantly.
|
||||
|
||||
This algorithm is based on Bayes' theorem:
|
||||
|
||||
```
|
||||
P(class | features) = (P(features | class) * P(class)) / P(features)
|
||||
```
|
||||
|
||||
where:
|
||||
- `P(class | features)` is the posterior probability of the class given the input features.
|
||||
- `P(features | class)` is the likelihood of the features given the class.
|
||||
- `P(class)` is the prior probability of the class.
|
||||
- `P(features)` is the probability of the input features.
|
||||
|
||||
To classify a new instance, Naïve Bayes calculates the posterior probability for each class, considering the product of the likelihoods of each feature given that class. It then selects the class with the highest probability as the predicted class for the input.
|
||||
|
||||
## Types of Naïve Bayes
|
||||
|
||||
There are different variations of Naïve Bayes classifiers, depending on the distribution assumptions made for the features. The most common types include:
|
||||
|
||||
1. **Gaussian Naïve Bayes**: Assumes that the continuous features follow a Gaussian distribution.
|
||||
2. **Multinomial Naïve Bayes**: Suitable for discrete features that represent counts or frequencies.
|
||||
3. **Bernoulli Naïve Bayes**: Designed for binary features, where each feature is either present or absent.
|
||||
|
||||
The choice of the type of Naïve Bayes depends on the nature of the dataset and the specific problem at hand.
|
||||
|
||||
## Advantages of Naïve Bayes
|
||||
|
||||
Naïve Bayes offers several advantages that make it a popular choice in many classification tasks:
|
||||
|
||||
1. **Simplicity**: It is a simple and easy-to-understand algorithm with relatively few parameters to tune.
|
||||
2. **Efficiency**: Naïve Bayes has fast training and prediction times, making it suitable for large datasets.
|
||||
3. **Good performance**: Despite the "naïve" assumption, Naïve Bayes often achieves competitive performance compared to more complex algorithms.
|
||||
4. **Robustness to irrelevant features**: Naïve Bayes performs well even in the presence of irrelevant features, as it assumes independence between the features.
|
||||
|
||||
## Limitations of Naïve Bayes
|
||||
|
||||
Although Naïve Bayes has many advantages, it also has some limitations, including:
|
||||
|
||||
1. **Assumption of feature independence**: The assumption of independence may not hold in many real-world scenarios, leading to potential inaccuracies.
|
||||
2. **Sensitive to feature distributions**: Naïve Bayes can struggle with features that have strong dependencies or non-linear relationships, as it assumes all features are equally important.
|
||||
3. **Lack of proper probability estimation**: The predicted probabilities from Naïve Bayes are not reliable measurements of true probabilities.
|
||||
|
||||
Despite these limitations, Naïve Bayes remains a popular and useful algorithm due to its simplicity and efficiency, especially in text classification problems.
|
||||
|
||||
In conclusion, Naïve Bayes is a powerful algorithm that provides a simple yet effective solution for classification tasks. Its assumptions of feature independence enable fast computation and often yield satisfactory results. By understanding the strengths and limitations of Naïve Bayes, data scientists can leverage its potential and apply it to various practical problems.
|
@ -1,43 +0,0 @@
|
||||
# Neural Networks: Unlocking the Power of Artificial Intelligence
|
||||
|
||||

|
||||
|
||||
Artificial Intelligence (AI) has emerged as one of the most transformative technologies of the 21st century. Within AI, neural networks have played a pivotal role in shaping the advancements we witness today. From image recognition to natural language processing, neural networks have revolutionized the way machines can learn, reason, and solve complex problems. In this article, we will dive deep into the world of neural networks, exploring their architecture, training process, and applications.
|
||||
|
||||
## Understanding Neural Networks
|
||||
|
||||
At its core, a neural network is a computer system designed to mimic the structure and functionality of a biological brain. It is composed of multiple interconnected nodes, called artificial neurons or simply "neurons." These artificial neurons are organized into layers: an input layer, one or more hidden layers, and an output layer.
|
||||
|
||||
The neurons within each layer are connected to the neurons in the subsequent layer via weighted connections. These connections can be thought of as synapses in a biological brain, through which information flows. Each connection is associated with a weight, which determines the strength or importance of the information it carries.
|
||||
|
||||
The basic working principle of a neural network involves receiving an input, processing it through the interconnected neurons, and producing an output. This process, known as forward propagation, allows the network to make predictions or classifications based on the input it receives.
|
||||
|
||||
## Training a Neural Network
|
||||
|
||||
To perform its designated task effectively, a neural network needs to be trained on large datasets. The training process involves presenting the network with input data along with corresponding correct output values, known as labels or targets. The network then adjusts the weights of its connections to minimize the difference between its predicted output and the correct output. This iterative optimization process is known as backpropagation.
|
||||
|
||||
During the training phase, the neural network learns to recognize patterns and derive complex representations from the input data. As the training progresses, the network gradually improves its ability to make accurate predictions or classifications. The more data the network is exposed to, the more it refines its internal parameters, enhancing its performance.
|
||||
|
||||
## Applications of Neural Networks
|
||||
|
||||
Neural networks have found applications in various domains, transforming industries and enabling new possibilities. Here are a few notable areas where neural networks have shown remarkable impact:
|
||||
|
||||
### Image and Object Recognition
|
||||
|
||||
Neural networks have revolutionized image recognition tasks. Deep Convolutional Neural Networks (CNNs) have achieved remarkable accuracy in tasks like image classification, object detection, and face recognition. Applications powered by these networks include autonomous vehicles, medical imaging, and surveillance systems.
|
||||
|
||||
### Natural Language Processing (NLP)
|
||||
|
||||
NLP focuses on enabling computers to understand, interpret, and generate human language. Neural networks, particularly Recurrent Neural Networks (RNNs) and Transformer models, have greatly contributed to advancements in machine translation, chatbots, voice recognition, sentiment analysis, and more.
|
||||
|
||||
### Forecasting and Predictive Analytics
|
||||
|
||||
Neural networks have demonstrated their efficacy in forecasting and predictive analytics. By training on historical data, these networks can uncover complex patterns and relationships, facilitating accurate predictions in fields like finance, weather forecasting, stock market analysis, and demand forecasting.
|
||||
|
||||
### Healthcare and Drug Discovery
|
||||
|
||||
In healthcare, neural networks are being leveraged for disease diagnosis, patient monitoring, and drug discovery. They aid in analyzing medical images, predicting disease progression, and designing new drugs through virtual screening, significantly accelerating the research and development process.
|
||||
|
||||
## Conclusion
|
||||
|
||||
Neural networks have become the backbone of modern artificial intelligence. Their ability to learn from data, mimic the human brain, and solve complex problems has made them indispensable in a variety of applications. As computational power continues to grow, and datasets become more expansive, we can expect neural networks to make further breakthroughs, driving the advancement of AI and unlocking its limitless potential.
|
@ -1,39 +0,0 @@
|
||||
# Policy Gradients
|
||||
|
||||
Policy gradients are a popular and powerful technique used in the field of reinforcement learning. They offer a way to optimize the policy of an agent by directly estimating and updating the policy parameters based on the observed rewards.
|
||||
|
||||
## Reinforcement Learning
|
||||
|
||||
To understand policy gradients, it's essential to have a basic understanding of reinforcement learning (RL). In RL, an agent interacts with an environment by taking actions, and the environment provides feedback in the form of rewards or penalties. The goal of the agent is to learn a policy, which is a mapping from states to actions, that maximizes the cumulative reward over time.
|
||||
|
||||
## Direct Policy Optimization
|
||||
|
||||
Policy gradients take a direct optimization approach to finding an optimal policy. Rather than estimating the value function or action-value function, they aim to optimize the policy without intermediate steps. This makes them well-suited for continuous action spaces and tasks with high dimensionality.
|
||||
|
||||
## The Policy Gradient Theorem
|
||||
|
||||
The policy gradient theorem provides the theoretical foundation for policy gradients. It states that the gradient of the expected discounted return with respect to the policy parameters is proportional to the expected sum of the gradients of the log-probabilities of each action multiplied by the corresponding reward.
|
||||
|
||||
In other words, the gradient of the expected return is a sum of gradients of log-probabilities times rewards. This gradient can be used to update the policy parameters in a way that maximizes the expected return.
|
||||
|
||||
## Vanilla Policy Gradient
|
||||
|
||||
The Vanilla Policy Gradient (VPG) algorithm is a simple implementation of policy gradients. It involves estimating gradients using Monte Carlo sampling of trajectories and updating the policy parameters based on these gradients. VPG has shown promising results in various domains, including games and robotics.
|
||||
|
||||
## Advantage Actor-Critic (A2C)
|
||||
|
||||
The Advantage Actor-Critic (A2C) algorithm is an extension of policy gradients that combines the benefits of both value-based and policy-based methods. A2C uses a separate value function to estimate the advantage of each action, which helps in reducing the variance of the gradient estimates.
|
||||
|
||||
By using a value function, A2C provides a baseline and makes the learning process less noisy, resulting in faster and more stable convergence.
|
||||
|
||||
## Proximal Policy Optimization (PPO)
|
||||
|
||||
Proximal Policy Optimization (PPO) is another popular algorithm that uses policy gradients. PPO addresses the issue of overly aggressive policy updates by introducing a surrogate objective function that puts a constraint on the policy divergence.
|
||||
|
||||
PPO iteratively samples multiple trajectories, computes the policy gradient, and performs multiple epochs of optimization updates. This approach results in significantly improved robustness and stability compared to previous methods.
|
||||
|
||||
## Conclusion
|
||||
|
||||
Policy gradients have become a prominent technique in reinforcement learning, enabling direct optimization of policies for a wide range of problems. Algorithms like Vanilla Policy Gradient, Advantage Actor-Critic, and Proximal Policy Optimization provide different approaches to policy optimization, each with their strengths and applications.
|
||||
|
||||
As research progresses, policy gradients are expected to continue evolving and contributing to the advancement of reinforcement learning, opening up new possibilities for autonomous agents in various domains.
|
@ -1,39 +0,0 @@
|
||||
# Principal Component Analysis (PCA)
|
||||
|
||||
Principal Component Analysis (PCA) is a statistical technique used for dimensionality reduction. It helps in transforming a large set of variables into a smaller set of new variables, known as principal components. These principal components retain most of the important information present in the original data.
|
||||
|
||||
PCA seeks to find the directions, or axes, along which the data varies the most. These axes are known as the principal components. The first principal component captures the maximum amount of variation in the data, and each subsequent component captures the remaining variation while being orthogonal (unrelated) to the previous components.
|
||||
|
||||
## How PCA works
|
||||
|
||||
1. Standardize the data: PCA is sensitive to the scale of variables, so it is important to standardize the data by subtracting the mean and dividing by the standard deviation.
|
||||
|
||||
2. Compute the covariance matrix: The covariance matrix measures the relationships and variances between the variables in the dataset.
|
||||
|
||||
3. Calculate the eigenvectors and eigenvalues: The eigenvectors represent the directions or principal components, and the eigenvalues represent the amount of variation explained by each component. The eigenvectors are derived from the covariance matrix.
|
||||
|
||||
4. Sort eigenvalues and select principal components: Sort the eigenvalues in descending order and select the top-k eigenvectors corresponding to the largest eigenvalues. These eigenvectors are the principal components.
|
||||
|
||||
5. Generate new dataset: Multiply the standardized dataset by the selected eigenvectors to obtain the transformed dataset with reduced dimensions. Each observation in the new dataset is a linear combination of the original variables.
|
||||
|
||||
## Benefits of PCA
|
||||
|
||||
1. Dimensionality reduction: PCA reduces the number of features or variables in a dataset while retaining most of the information. It helps remove noisy or less important components and focuses on the most informative ones.
|
||||
|
||||
2. Enhanced interpretability: With fewer variables, it becomes easier to understand and visualize the data. The principal components are new variables that are a combination of the original variables, allowing for a more straightforward interpretation.
|
||||
|
||||
3. Improved efficiency: The reduced dataset after PCA requires less computational time and memory, making it more efficient for subsequent analysis.
|
||||
|
||||
4. Data visualization: PCA can be used to create 2D or 3D scatter plots that show the data points in reduced dimensions. It helps visualize the patterns, clusters, and relationships between observations.
|
||||
|
||||
## Limitations of PCA
|
||||
|
||||
1. Linearity assumption: PCA assumes a linear relationship between variables. If the dataset exhibits non-linear relationships, PCA may not be the most suitable technique.
|
||||
|
||||
2. Information loss: Although PCA retains most of the variation, there is still some information loss, especially when reducing dimensions significantly. It is important to consider the retained variance and carefully select the number of components to avoid losing critical information.
|
||||
|
||||
3. Difficulty in interpretation: While PCA enhances interpretability, the transformed variables (principal components) may not always directly relate to the original variables. Understanding the relationship between the principal components and the original variables can be challenging.
|
||||
|
||||
4. Sensitivity to outliers: PCA is sensitive to outliers; extreme values in the dataset can have a significant impact on the derived principal components.
|
||||
|
||||
In conclusion, PCA is a valuable technique for dimensionality reduction in data analysis. It helps simplify complex datasets, discover patterns, and improve computational efficiency. However, careful consideration of its assumptions, information loss, and proper selection of the number of components is crucial for effective application and interpretation of PCA.
|
@ -1,33 +0,0 @@
|
||||
# Proximal Policy Optimization (PPO)
|
||||
|
||||
Proximal Policy Optimization (PPO) is a reinforcement learning algorithm developed by OpenAI. It is designed to address the challenges of optimizing policies for reinforcement learning tasks. PPO is considered one of the most effective and popular algorithms for training agents in various domains, including robotics, games, and control systems.
|
||||
|
||||
## Background
|
||||
|
||||
Reinforcement learning (RL) is a branch of machine learning that involves training an agent to take actions in an environment to maximize some notion of cumulative reward. RL algorithms typically try to optimize the agent's policy, which determines the actions it takes based on the current state.
|
||||
|
||||
PPO is an approach that falls under the category of "on-policy" methods in RL. On-policy methods update the agent's policy using data collected from the most recent policy. The key challenge in on-policy methods is to balance the trade-off between exploration and exploitation. Exploration refers to the agent exploring the environment to gather new information, while exploitation involves exploiting the current knowledge to maximize the rewards obtained.
|
||||
|
||||
## The PPO Algorithm
|
||||
|
||||
PPO tackles the exploration-exploitation trade-off by introducing a parameter known as the "clip parameter." The clip parameter restricts the change that can be made to the policy during each update. By limiting the change, PPO ensures that an update does not deviate the policy too far from the previous version, preventing catastrophic performance deterioration.
|
||||
|
||||
The PPO algorithm consists of the following steps:
|
||||
|
||||
1. Collect data by running the current policy in the environment.
|
||||
2. Compute the advantages, which quantify how much better or worse each action is compared to the average.
|
||||
3. Update the policy by maximizing the objective function subject to the clip parameter. PPO performs multiple iterations of this step to gradually improve the policy.
|
||||
4. Repeat steps 1-3 until the desired performance is achieved.
|
||||
|
||||
PPO is known for its simplicity and effectiveness. It has achieved state-of-the-art results in various tasks, including complex environments with high-dimensional observations and continuous action spaces.
|
||||
|
||||
## Benefits of PPO
|
||||
|
||||
1. **Sample Efficiency**: PPO is known for its sample efficiency, meaning it requires relatively few interactions with the environment to achieve good performance.
|
||||
2. **Stability**: By constraining the policy updates, PPO provides stability to the learning process and prevents drastic policy changes that can harm performance.
|
||||
3. **Generalization**: PPO performs well across a wide range of tasks and environments, making it a versatile algorithm for reinforcement learning problems.
|
||||
4. **Easy to Implement**: PPO's simplicity makes it easy to understand and implement, making it accessible even to beginners in the field of RL.
|
||||
|
||||
## Conclusion
|
||||
|
||||
Proximal Policy Optimization (PPO) is a powerful algorithm for training agents in reinforcement learning tasks. Its ability to strike a balance between exploration and exploitation using the clip parameter has made it a popular choice among researchers and practitioners. PPO's simplicity, stability, and sample efficiency make it an excellent choice for a wide range of RL applications, and it continues to drive advancements in the field.
|
@ -1,23 +0,0 @@
|
||||
# AI Generated Articles
|
||||
|
||||
- [Actor-Critic Methods](Actor-Critic_Methods.md)
|
||||
- [Association Rules (Apriori, FP-Growth)](Association_Rules_(Apriori,_FP-Growth).md)
|
||||
- [DBSCAN](DBSCAN.md)
|
||||
- [Decision Trees](Decision_Trees.md)
|
||||
- [Gaussian Mixture Models (GMM)](Gaussian_Mixture_Models_(GMM).md)
|
||||
- [Gradient Boosting Machines (GBM)](Gradient_Boosting_Machines_(GBM).md)
|
||||
- [Independent Component Analysis (ICA)](Independent_Component_Analysis_(ICA).md)
|
||||
- [Latent Dirichlet Allocation (LDA)](Latent_Dirichlet_Allocation_(LDA).md)
|
||||
- [Monte Carlo Tree Search (MCTS)](Monte_Carlo_Tree_Search_(MCTS).md)
|
||||
- [Naïve Bayes](Naïve_Bayes.md)
|
||||
- [Neural Networks](Neural_Networks.md)
|
||||
- [Policy Gradients](Policy_Gradients.md)
|
||||
- [Principal Component Analysis (PCA)](Principal_Component_Analysis_(PCA).md)
|
||||
- [Proximal Policy Optimization (PPO)](Proximal_Policy_Optimization_(PPO).md)
|
||||
- [Random Forests](Random_Forests.md)
|
||||
- [SARSA](SARSA.md)
|
||||
- [Support Vector Machines (SVM)](Support_Vector_Machines_(SVM).md)
|
||||
- [Temporal Difference Learning (TD Learning)](Temporal_Difference_Learning_(TD_Learning).md)
|
||||
- [Trust Region Policy Optimization (TRPO)](Trust_Region_Policy_Optimization_(TRPO).md)
|
||||
- [k-Nearest Neighbors (k-NN)](k-Nearest_Neighbors_(k-NN).md)
|
||||
- [t-SNE](t-SNE.md)
|
@ -1,39 +0,0 @@
|
||||
# Random Forests
|
||||
|
||||
Random Forests is a machine learning algorithm that is widely used for classification and regression tasks. It is an ensemble learning method that combines multiple decision trees to make accurate predictions. The algorithm was introduced by Leo Breiman and Adele Cutler in 2001.
|
||||
|
||||
## How does it work?
|
||||
|
||||
Random Forests is based on the concept of decision trees. A decision tree is a flowchart-like structure where each node represents a feature, each branch represents a decision rule, and each leaf node represents the outcome or prediction. However, a single decision tree may suffer from overfitting or bias, which can lead to poor generalization.
|
||||
|
||||
To address this issue, Random Forests builds an ensemble of decision trees and combines their predictions using averaging or voting. The ensemble approach helps to reduce overfitting and improves the accuracy of the model. Each decision tree is trained on a random subset of the training data and a random subset of the features, hence the name "Random Forests."
|
||||
|
||||
## Key features
|
||||
|
||||
1. **Random Sampling**: Random Forests randomly selects a subset of the training data for each decision tree. This technique, called bootstrap aggregating or "bagging," introduces randomness and reduces the variance of the model.
|
||||
|
||||
2. **Random Feature Selection**: In addition to sampling the data, Random Forests also randomly selects a subset of features for each decision tree. By considering different combinations of features, the algorithm increases diversity among trees and improves the overall performance.
|
||||
|
||||
3. **Voting or Averaging**: Once the ensemble of decision trees is built, Random Forests combines their predictions through voting (for classification tasks) or averaging (for regression tasks). This aggregation helps to improve the model's accuracy and reduce overfitting.
|
||||
|
||||
## Advantages of Random Forests
|
||||
|
||||
- Random Forests can handle large data sets with high dimensionality without overfitting. It is robust to noise and outliers that might exist in the training set.
|
||||
|
||||
- The algorithm can provide a feature importance ranking, indicating which features are most relevant for the task.
|
||||
|
||||
- Random Forests are less prone to overfitting compared to a single decision tree. By combining multiple decision trees, the model achieves a balance between bias and variance.
|
||||
|
||||
- The algorithm's versatility allows it to be used for both classification and regression tasks.
|
||||
|
||||
## Limitations of Random Forests
|
||||
|
||||
- Random Forests can be computationally expensive, especially when dealing with large datasets. The training time increases as the number of decision trees or features grows.
|
||||
|
||||
- Interpretability of Random Forests can be challenging, especially compared to single decision trees. It can be difficult to understand the underlying logic of the ensemble model.
|
||||
|
||||
- Random Forests may not perform well if there are strong, complex relationships between features. In such cases, other algorithms like gradient boosting or deep learning models might yield better results.
|
||||
|
||||
## Conclusion
|
||||
|
||||
Random Forests is a powerful machine learning algorithm that combines the strengths of decision trees with ensemble methods. Its ability to handle large datasets, reduce overfitting, and generate feature importance rankings makes it a popular choice in many practical applications. However, it is important to consider its limitations and choose the appropriate algorithm for specific task requirements.
|
@ -1,46 +0,0 @@
|
||||
# SARSA: An Introduction to Reinforcement Learning
|
||||
|
||||
Reinforcement Learning (RL) is a subfield of machine learning concerned with training agents to make decisions in an environment, maximizing a notion of cumulative reward. One popular RL method is **SARSA**, which stands for State-Action-Reward-State-Action. SARSA is an on-policy, model-free control algorithm with applications ranging from robotics to game playing.
|
||||
|
||||
## The Basic Idea
|
||||
|
||||
SARSA utilizes a table, often called a Q-table, to estimate the value of each state-action pair. The Q-table maps the state-action pairs to a numeric value representing the expected cumulative reward. The algorithm aims to learn the optimal policy, which is the sequence of actions that yields the highest cumulative reward over time.
|
||||
|
||||
## The SARSA Algorithm
|
||||
|
||||
The SARSA algorithm is relatively simple to understand, making it a popular choice for introductory RL tutorials. Here is a step-by-step breakdown of the algorithm:
|
||||
|
||||
1. Initialize the Q-table with small random values.
|
||||
2. Observe the current state **s**.
|
||||
3. Choose an action **a** using an exploration-exploitation trade-off strategy (such as ε-greedy).
|
||||
4. Perform the chosen action **a** in the environment.
|
||||
5. Observe the reward **r** and the new state **s'**.
|
||||
6. Choose a new action **a'** for the new state **s'** using the same exploration-exploitation strategy.
|
||||
7. Update the Q-table value for the state-action pair **(s, a)** using the update rule:
|
||||
|
||||
```
|
||||
Q(s,a) = Q(s,a) + α⋅[R + γ⋅Q(s',a') - Q(s,a)]
|
||||
```
|
||||
|
||||
where:
|
||||
- **α** is the learning rate, controlling the weight given to the new information.
|
||||
- **R** is the observed reward for the state-action pair.
|
||||
- **γ** is the discount factor, determining the importance of future rewards.
|
||||
|
||||
8. Set the current state and action to the new state and action determined above (i.e., **s = s'** and **a = a'**).
|
||||
9. Repeat steps 2 to 8 until the agent reaches a terminal state or a predefined number of iterations.
|
||||
|
||||
## Advantages and Limitations
|
||||
|
||||
SARSA has several advantages that contribute to its popularity:
|
||||
- Simplicity: SARSA is relatively easy to understand and implement, making it a great starting point for beginners.
|
||||
- On-policy: It learns and improves the policy it follows while interacting with the environment, making it robust to changes in policy during training.
|
||||
- Works with continuous state and action spaces: Unlike some other RL algorithms, SARSA can handle continuous state and action spaces effectively.
|
||||
|
||||
However, SARSA also has a few limitations:
|
||||
- Less efficient for large state spaces: SARSA's reliance on a Q-table becomes impractical when the state space is exceptionally large, as it would require significant memory resources.
|
||||
- Struggles with high-dimensional or continuous action spaces: SARSA struggles in situations where the number of possible actions is large or continuous, as the action-state value function becomes difficult to approximate accurately.
|
||||
|
||||
## Conclusion
|
||||
|
||||
SARSA is a fundamental reinforcement learning algorithm that provides an introduction to the field. Although it may have limitations in certain scenarios, SARSA is a valuable tool with various applications. As machine learning research continues to evolve, SARSA's simplicity and intuition make it an essential algorithm for studying reinforcement learning.
|
@ -1,40 +0,0 @@
|
||||
# Support Vector Machines (SVM)
|
||||
|
||||
Support Vector Machines (SVM) is a powerful machine learning algorithm that is widely used for classification and regression tasks. It has gained popularity due to its ability to handle high-dimensional datasets and provide accurate results. In this article, we will explore the workings of SVM and its various applications.
|
||||
|
||||
## Introduction to SVM
|
||||
|
||||
Support Vector Machines are supervised learning models that analyze data and classify it into different categories. The algorithm uses a technique called **maximum margin classification** to find the best possible decision boundary that separates the data points of one class from another. The decision boundary is known as a **hyperplane**.
|
||||
|
||||
## Working of SVM
|
||||
|
||||
SVM works by mapping the input data to a high-dimensional feature space. In this feature space, the algorithm tries to find a hyperplane that maximizes the distance between the data points of different classes, known as **support vectors**. By maximizing this margin, SVM can generalize well and provide robust predictions on new data points.
|
||||
|
||||
There are two types of SVM:
|
||||
|
||||
1. **Linear SVM**: In linear SVM, a linear decision boundary is created to classify the data points into different classes.
|
||||
2. **Non-linear SVM**: Non-linear SVM uses techniques such as **kernel functions** to transform the data into a higher-dimensional space, where a linear decision boundary can be found.
|
||||
|
||||
SVM is also useful for regression tasks. In regression, the algorithm tries to fit a hyperplane that best represents the trend of the data points.
|
||||
|
||||
## Advantages of SVM
|
||||
|
||||
SVM has several advantages that contribute to its popularity:
|
||||
|
||||
1. **Effective in high-dimensional spaces**: SVM performs well even when the number of dimensions is larger than the number of samples, making it suitable for complex datasets.
|
||||
2. **Memory-efficient**: SVM uses a subset of training points (support vectors) to make predictions, making it memory-efficient.
|
||||
3. **Accurate results**: SVM finds the optimal decision boundary by maximizing the margin, resulting in accurate predictions.
|
||||
4. **Handles non-linear data**: By using kernel functions, SVM can handle non-linear data and find complex decision boundaries.
|
||||
|
||||
## Applications of SVM
|
||||
|
||||
SVM finds applications in various domains, including:
|
||||
|
||||
1. **Text classification**: SVM can classify text documents into multiple categories, making it useful for sentiment analysis, spam detection, and topic classification.
|
||||
2. **Image classification**: SVM is used for image recognition tasks, such as identifying objects, faces, and handwritten digits.
|
||||
3. **Bioinformatics**: SVM is employed in protein classification, gene expression analysis, and disease detection.
|
||||
4. **Finance**: SVM is utilized in credit scoring, stock market forecasting, and fraud detection.
|
||||
|
||||
## Conclusion
|
||||
|
||||
Support Vector Machines (SVM) are powerful machine learning algorithms that have proven to be effective in various domains. Their ability to handle high-dimensional data and provide accurate results makes them a popular choice for classification and regression tasks. By finding the optimal decision boundary, SVM can generalize well and yield robust predictions.
|
@ -1,39 +0,0 @@
|
||||
# Temporal Difference Learning (TD Learning)
|
||||
|
||||
Temporal Difference (TD) learning is a popular and widely used technique in the field of artificial intelligence and reinforcement learning. It is a combination of two important learning approaches, namely Monte Carlo methods and dynamic programming.
|
||||
|
||||
## Introduction
|
||||
|
||||
TD learning is a type of model-free reinforcement learning. It is used to estimate the value function or expected return of a given state in a Markov Decision Process (MDP) without explicitly knowing the underlying dynamics of the environment.
|
||||
|
||||
## How TD Learning Works
|
||||
|
||||
TD learning operates by bootstrapping, which means it updates the value function estimate based on the current estimate itself. The basic idea is to learn from each interaction with the environment by updating the value estimate according to the difference between the current estimate and the updated estimate.
|
||||
|
||||
TD learning achieves this by using a combination of prediction and control techniques. Prediction involves estimating the expected return or value of a specific state, while control refers to the process of adjusting actions to maximize the accumulated reward.
|
||||
|
||||
## Key Concepts in TD Learning
|
||||
|
||||
There are a few key concepts that are important to understand in TD learning:
|
||||
|
||||
1. **State-Value Functions** - State-value functions estimate the expected return starting from a specific state and following a specific policy. In TD learning, these functions are recursively updated based on the difference between the current estimate and the updated estimate.
|
||||
|
||||
2. **Action-Value Functions** - Action-value functions estimate the expected return from taking a specific action in a specific state and following a specific policy. These functions are also updated using temporal difference updates.
|
||||
|
||||
3. **Learning Rate** - TD learning employs a learning rate parameter that controls the weight given to new information compared to the existing estimate. It determines how fast the value function converges to the true values.
|
||||
|
||||
4. **Exploration vs. Exploitation** - TD learning balances exploration and exploitation by making decisions that are not only based on the current policy but also considering the potential reward from exploring different actions.
|
||||
|
||||
## Applications of TD Learning
|
||||
|
||||
TD learning has found widespread applications in various fields. Some notable examples include:
|
||||
|
||||
- Reinforcement learning problems: TD learning is often employed in reinforcement learning tasks, where agents learn to interact with an environment by maximizing the rewards obtained over time.
|
||||
|
||||
- Game playing: TD learning has been successfully applied to train intelligent agents for playing games. Notable examples include TD-Gammon, a backgammon-playing program that achieved remarkable performance through self-play and TD learning.
|
||||
|
||||
- Robotics and control applications: TD learning has been utilized in robotics and control systems to learn optimal policies or value functions for achieving specific goals or tasks.
|
||||
|
||||
## Conclusion
|
||||
|
||||
Temporal Difference learning is a powerful and versatile technique for reinforcement learning. Its ability to learn from each interaction with the environment and its combination of prediction and control methods make it valuable for various applications. By utilizing TD learning, intelligent systems and agents can learn to make optimal decisions and actions in complex and dynamic environments.
|
@ -1,50 +0,0 @@
|
||||
# Trust Region Policy Optimization (TRPO)
|
||||
|
||||
Trust Region Policy Optimization (TRPO) is a reinforcement learning algorithm that aims to optimize policies in reinforcement learning problems, with a particular focus on continuous control tasks. It was introduced by Schulman et al. in 2015 and has gained popularity for its ability to find near-optimal policies while ensuring stability and safety in training.
|
||||
|
||||
## Background
|
||||
|
||||
Reinforcement learning involves training an autonomous agent to learn optimal actions in an environment through trial and error. The agent interacts with the environment, receives feedback in the form of rewards, and adjusts its policy to maximize the cumulative rewards. However, optimizing policies in environments with high-dimensional continuous action spaces can be challenging.
|
||||
|
||||
TRPO addresses this challenge by leveraging a trust region approach, where the policy's updates are constrained within a trust region to ensure the model doesn't change too drastically in each iteration. This limitation prevents policy divergence and helps in efficient policy updates.
|
||||
|
||||
## Key Ideas and Mechanisms
|
||||
|
||||
TRPO achieves optimization stability and safety through two main mechanisms:
|
||||
|
||||
### Surrogate objective
|
||||
|
||||
TRPO optimizes a surrogate objective function called the Surrogate Advantage Function, which approximates the expected improvement in expected rewards. This objective function guides the policy optimization by estimating the advantage of each action taken by the policy in comparison to other possible actions.
|
||||
|
||||
### Trust region constraint
|
||||
|
||||
The trust region constraint helps limit policy changes during updates. It ensures that the updated policy does not deviate significantly from the previous one, preventing catastrophic changes that can lead to suboptimal policies. By constraining updates within a trust region, TRPO provides robustness and stability during training.
|
||||
|
||||
## Algorithm Steps
|
||||
|
||||
The TRPO algorithm typically consists of the following steps:
|
||||
|
||||
1. Collect a set of trajectories by executing the current policy in the environment.
|
||||
2. Compute the advantages for each state-action pair using the Surrogate Advantage Function.
|
||||
3. Calculate the policy update by optimizing the Surrogate Advantage Function subject to the trust region constraint.
|
||||
4. Perform a line search to find the optimal step size for the policy update under the trust region constraint.
|
||||
5. Update the policy parameters using the obtained step size.
|
||||
6. Repeat steps 1-5 until the policy converges.
|
||||
|
||||
## Benefits and Limitations
|
||||
|
||||
TRPO offers several benefits which make it an attractive choice for policy optimization in reinforcement learning:
|
||||
|
||||
- Stability: TRPO guarantees stability during training by ensuring updates are within a trust region.
|
||||
- Sample Efficiency: It makes efficient use of collected experience to optimize policies.
|
||||
- Convergence: TRPO is known to converge to near-optimal policies when properly tuned.
|
||||
|
||||
However, there are also a few limitations to consider:
|
||||
|
||||
- Computational Complexity: TRPO can be computationally expensive due to the need for multiple iterations and line searches.
|
||||
- Parameter Tuning: Fine-tuning the key hyperparameters is crucial for effective performance.
|
||||
- High-Dimensional Action Spaces: Although TRPO is tailored for continuous control problems, it might face challenges with high-dimensional action spaces.
|
||||
|
||||
## Conclusion
|
||||
|
||||
Trust Region Policy Optimization (TRPO) has emerged as a powerful and widely-used algorithm for policy optimization and reinforcement learning tasks, especially in continuous control settings. By combining the surrogate objective function and trust region constraint, it ensures stable and safe policy updates, leading to near-optimal performance. While TRPO has its limitations, its benefits in stability, sample efficiency, and convergence make it an important algorithm in modern reinforcement learning research and applications.
|
@ -1,106 +0,0 @@
|
||||
Sure! Here's an example of a Python script that demonstrates Actor-Critic Methods using the OpenAI Gym environment and the Keras library:
|
||||
|
||||
```python
|
||||
import gym
|
||||
import numpy as np
|
||||
from tensorflow.keras.models import Model
|
||||
from tensorflow.keras.layers import Input, Dense
|
||||
from tensorflow.keras.optimizers import Adam
|
||||
|
||||
|
||||
class ActorCriticAgent:
|
||||
def __init__(self, state_size, action_size):
|
||||
self.state_size = state_size
|
||||
self.action_size = action_size
|
||||
|
||||
# Hyperparameters for the agent
|
||||
self.lr_actor = 0.001
|
||||
self.lr_critic = 0.005
|
||||
self.discount_factor = 0.99
|
||||
|
||||
# Create models for the actor and critic
|
||||
self.actor = self.build_actor()
|
||||
self.critic = self.build_critic()
|
||||
|
||||
def build_actor(self):
|
||||
input = Input(shape=(self.state_size,))
|
||||
dense = Dense(24, activation='relu')(input)
|
||||
probs = Dense(self.action_size, activation='softmax')(dense)
|
||||
model = Model(inputs=input, outputs=probs)
|
||||
model.compile(loss='categorical_crossentropy', optimizer=Adam(lr=self.lr_actor))
|
||||
return model
|
||||
|
||||
def build_critic(self):
|
||||
input = Input(shape=(self.state_size,))
|
||||
dense = Dense(24, activation='relu')(input)
|
||||
value = Dense(1, activation='linear')(dense)
|
||||
model = Model(inputs=input, outputs=value)
|
||||
model.compile(loss='mse', optimizer=Adam(lr=self.lr_critic))
|
||||
return model
|
||||
|
||||
def get_action(self, state):
|
||||
state = np.reshape(state, [1, self.state_size])
|
||||
probs = self.actor.predict(state)[0]
|
||||
action = np.random.choice(self.action_size, p=probs)
|
||||
return action
|
||||
|
||||
def train_model(self, state, action, reward, next_state, done):
|
||||
target = np.zeros((1, self.action_size))
|
||||
advantages = np.zeros((1, self.action_size))
|
||||
|
||||
value = self.critic.predict(state)[0]
|
||||
next_value = self.critic.predict(next_state)[0]
|
||||
|
||||
if done:
|
||||
advantages[0][action] = reward - value
|
||||
target[0][action] = reward
|
||||
else:
|
||||
advantages[0][action] = reward + self.discount_factor * (next_value) - value
|
||||
target[0][action] = reward + self.discount_factor * next_value
|
||||
|
||||
self.actor.fit(state, advantages, epochs=1, verbose=0)
|
||||
self.critic.fit(state, target, epochs=1, verbose=0)
|
||||
|
||||
|
||||
if __name__ == "__main__":
|
||||
# Create the environment
|
||||
env = gym.make('CartPole-v1')
|
||||
state_size = env.observation_space.shape[0]
|
||||
action_size = env.action_space.n
|
||||
|
||||
# Create an instance of the agent
|
||||
agent = ActorCriticAgent(state_size, action_size)
|
||||
|
||||
scores, episodes = [], []
|
||||
EPISODES = 100
|
||||
|
||||
for episode in range(EPISODES):
|
||||
done = False
|
||||
score = 0
|
||||
state = env.reset()
|
||||
|
||||
while not done:
|
||||
action = agent.get_action(state)
|
||||
next_state, reward, done, info = env.step(action)
|
||||
agent.train_model(state, action, reward, next_state, done)
|
||||
score += reward
|
||||
state = next_state
|
||||
|
||||
if done:
|
||||
scores.append(score)
|
||||
episodes.append(episode)
|
||||
print("Episode:", episode, "Score:", score)
|
||||
|
||||
# Plot the scores
|
||||
import matplotlib.pyplot as plt
|
||||
plt.plot(episodes, scores, 'b')
|
||||
plt.xlabel("Episode")
|
||||
plt.ylabel("Score")
|
||||
plt.show()
|
||||
```
|
||||
|
||||
In this script, we create an `ActorCriticAgent` class that represents the agent using Actor-Critic Methods. We then use this agent to train on the `CartPole-v1` environment from OpenAI Gym. The agent has an actor model that outputs action probabilities and a critic model that predicts values. These models are trained using the actor-critic algorithm in the `train_model` method.
|
||||
|
||||
During training, the agent selects actions based on the actor model's output and updates its models using the observed rewards and states. The scores are stored and plotted at the end to visualize the agent's performance over multiple episodes.
|
||||
|
||||
Please make sure you have installed the required libraries (`gym`, `numpy`, `tensorflow`, `keras`, and `matplotlib`) before running this script.
|
@ -1,46 +0,0 @@
|
||||
Sure! Here's a Python script that demonstrates the use of Association Rules using the Apriori and FP-Growth algorithms.
|
||||
|
||||
```python
|
||||
import pandas as pd
|
||||
from mlxtend.preprocessing import TransactionEncoder
|
||||
from mlxtend.frequent_patterns import apriori, association_rules
|
||||
from mlxtend.frequent_patterns import fpgrowth
|
||||
|
||||
# Sample dataset
|
||||
dataset = [['Milk', 'Onion', 'Nutmeg', 'Kidney Beans', 'Eggs', 'Yogurt'],
|
||||
['Dill', 'Onion', 'Nutmeg', 'Kidney Beans', 'Eggs', 'Yogurt'],
|
||||
['Milk', 'Apple', 'Kidney Beans', 'Eggs'],
|
||||
['Milk', 'Unicorn', 'Corn', 'Kidney Beans', 'Yogurt'],
|
||||
['Corn', 'Onion', 'Onion', 'Kidney Beans', 'Ice cream', 'Eggs']]
|
||||
|
||||
# Create one-hot encoded DataFrame
|
||||
te = TransactionEncoder()
|
||||
te_data = te.fit(dataset).transform(dataset)
|
||||
df = pd.DataFrame(te_data, columns=te.columns_)
|
||||
|
||||
# Generate frequent itemsets using Apriori algorithm
|
||||
frequent_itemsets_apriori = apriori(df, min_support=0.2, use_colnames=True)
|
||||
print("Frequent Itemsets (Apriori):")
|
||||
print(frequent_itemsets_apriori)
|
||||
|
||||
# Generate association rules from frequent itemsets using confidence threshold
|
||||
rules_apriori = association_rules(frequent_itemsets_apriori, metric="confidence", min_threshold=0.7)
|
||||
print("\nAssociation Rules (Apriori):")
|
||||
print(rules_apriori)
|
||||
|
||||
# Generate frequent itemsets using FP-Growth algorithm
|
||||
frequent_itemsets_fpgrowth = fpgrowth(df, min_support=0.2, use_colnames=True)
|
||||
print("\nFrequent Itemsets (FP-Growth):")
|
||||
print(frequent_itemsets_fpgrowth)
|
||||
|
||||
# Generate association rules from frequent itemsets using confidence threshold
|
||||
rules_fpgrowth = association_rules(frequent_itemsets_fpgrowth, metric="confidence", min_threshold=0.7)
|
||||
print("\nAssociation Rules (FP-Growth):")
|
||||
print(rules_fpgrowth)
|
||||
```
|
||||
|
||||
This script uses the `mlxtend` library, which provides implementations of the Apriori and FP-Growth algorithms. It first creates a one-hot encoded DataFrame from the given dataset. Then, it applies the Apriori algorithm to generate frequent itemsets and prints them. Next, it applies the association rules algorithm to derive association rules from the frequent itemsets and prints them.
|
||||
|
||||
Similarly, it applies the FP-Growth algorithm to generate frequent itemsets and prints them. Finally, it applies the association rules algorithm to derive association rules from the frequent itemsets generated by FP-Growth and prints them.
|
||||
|
||||
You can replace the `dataset` variable with your own dataset to observe the results for your data. Make sure to install the `mlxtend` library before running this script by using the command `pip install mlxtend`.
|
@ -1,50 +0,0 @@
|
||||
Sure! Here's an example of a Python script that demonstrates the DBSCAN algorithm using the iris dataset from scikit-learn:
|
||||
|
||||
```python
|
||||
import numpy as np
|
||||
import matplotlib.pyplot as plt
|
||||
from sklearn import datasets
|
||||
from sklearn.cluster import DBSCAN
|
||||
from sklearn.preprocessing import StandardScaler
|
||||
|
||||
# Load the iris dataset
|
||||
iris = datasets.load_iris()
|
||||
X = iris.data
|
||||
|
||||
# Scale the features
|
||||
X = StandardScaler().fit_transform(X)
|
||||
|
||||
# Apply DBSCAN
|
||||
dbscan = DBSCAN(eps=0.3, min_samples=5)
|
||||
dbscan.fit(X)
|
||||
|
||||
# Get the predicted labels and number of clusters
|
||||
labels = dbscan.labels_
|
||||
n_clusters = len(set(labels)) - (1 if -1 in labels else 0)
|
||||
n_noise = list(labels).count(-1)
|
||||
|
||||
# Plot the results
|
||||
unique_labels = set(labels)
|
||||
colors = [plt.cm.Spectral(each)
|
||||
for each in np.linspace(0, 1, len(unique_labels))]
|
||||
|
||||
for k, col in zip(unique_labels, colors):
|
||||
if k == -1:
|
||||
# Black used for noise
|
||||
col = [0, 0, 0, 1]
|
||||
|
||||
class_member_mask = (labels == k)
|
||||
|
||||
xy = X[class_member_mask & core_samples_mask]
|
||||
plt.plot(xy[:, 0], xy[:, 1], 'o', markerfacecolor=tuple(col),
|
||||
markeredgecolor='k', markersize=6)
|
||||
|
||||
plt.title(f"Estimated number of clusters: {n_clusters}, Noise points: {n_noise}")
|
||||
plt.show()
|
||||
```
|
||||
|
||||
In this script, we first load the iris dataset and normalize the features using `StandardScaler`. Then we apply DBSCAN with `eps=0.3` (maximum distance between samples to form a cluster) and `min_samples=5` (minimum number of samples in a cluster).
|
||||
|
||||
Next, we retrieve the predicted labels and calculate the number of clusters and noise points. Finally, we plot the data points, highlighting the clusters and noise using different colors.
|
||||
|
||||
Note that you may need to have scikit-learn and matplotlib installed for this script to work.
|
@ -1,44 +0,0 @@
|
||||
Sure! Below is a Python script that demonstrates the concept of Decision Trees using the popular scikit-learn library.
|
||||
|
||||
```python
|
||||
# Import necessary libraries
|
||||
from sklearn.datasets import load_iris
|
||||
from sklearn.model_selection import train_test_split
|
||||
from sklearn.tree import DecisionTreeClassifier
|
||||
from sklearn import metrics
|
||||
|
||||
# Load the Iris dataset
|
||||
data = load_iris()
|
||||
X = data.data
|
||||
y = data.target
|
||||
|
||||
# Split the dataset into training and testing sets
|
||||
X_train, X_test, y_train, y_test = train_test_split(X, y, test_size=0.2, random_state=42)
|
||||
|
||||
# Create a Decision Tree classifier
|
||||
clf = DecisionTreeClassifier()
|
||||
|
||||
# Train the classifier on the training data
|
||||
clf.fit(X_train, y_train)
|
||||
|
||||
# Make predictions on the testing data
|
||||
y_pred = clf.predict(X_test)
|
||||
|
||||
# Evaluate the model
|
||||
accuracy = metrics.accuracy_score(y_test, y_pred)
|
||||
print("Accuracy:", accuracy)
|
||||
|
||||
# Visualize the Decision Tree
|
||||
from sklearn import tree
|
||||
import matplotlib.pyplot as plt
|
||||
|
||||
plt.figure(figsize=(12, 8))
|
||||
tree.plot_tree(clf, feature_names=data.feature_names, class_names=data.target_names, filled=True)
|
||||
plt.show()
|
||||
```
|
||||
|
||||
In this script, we first import the necessary libraries: `load_iris` from `sklearn.datasets` to load the Iris dataset, `train_test_split` from `sklearn.model_selection` to split the dataset into training and testing sets, `DecisionTreeClassifier` from `sklearn.tree` to create the Decision Tree classifier, and `metrics` from `sklearn` to evaluate the model.
|
||||
|
||||
We load the Iris dataset and split it into training and testing sets using a 80:20 split. Then, we create a Decision Tree classifier and train it on the training data. After that, we make predictions on the testing data and evaluate the model using accuracy as the metric.
|
||||
|
||||
Finally, we visualize the Decision Tree using `tree.plot_tree` from `sklearn` and `matplotlib.pyplot`. The resulting tree is displayed using a figure.
|
@ -1,35 +0,0 @@
|
||||
Sure! Here's a Python script that demonstrates the concept of Gaussian Mixture Models (GMM) using the scikit-learn library:
|
||||
|
||||
```python
|
||||
import numpy as np
|
||||
import matplotlib.pyplot as plt
|
||||
from sklearn.mixture import GaussianMixture
|
||||
|
||||
# Generate random data
|
||||
np.random.seed(0)
|
||||
n_samples = 1000
|
||||
n_components = 3
|
||||
X = np.concatenate([
|
||||
np.random.multivariate_normal([0, 0], [[1, 0], [0, 20]], int(0.1 * n_samples)),
|
||||
np.random.multivariate_normal([-5, 0], [[1, 0], [0, 1]], int(0.3 * n_samples)),
|
||||
np.random.multivariate_normal([5, 10], [[4, 0], [0, 4]], int(0.6 * n_samples))
|
||||
])
|
||||
|
||||
# Fit the GMM model
|
||||
gmm = GaussianMixture(n_components=n_components)
|
||||
gmm.fit(X)
|
||||
|
||||
# Predict the labels for the data samples
|
||||
y_pred = gmm.predict(X)
|
||||
|
||||
# Visualize the results
|
||||
plt.scatter(X[:, 0], X[:, 1], c=y_pred, cmap='viridis')
|
||||
plt.xlabel('X')
|
||||
plt.ylabel('Y')
|
||||
plt.title('Gaussian Mixture Models')
|
||||
plt.show()
|
||||
```
|
||||
|
||||
In this script, we first generate random data points from three different Gaussian distributions. We then use the `GaussianMixture` class from scikit-learn to fit a GMM model on this data. Next, we use the learned GMM model to predict the labels for each data sample. Finally, we visualize the results by plotting the data points colored according to their predicted labels.
|
||||
|
||||
Please make sure you have the scikit-learn and matplotlib libraries installed in your Python environment before running this script.
|
@ -1,34 +0,0 @@
|
||||
Sure! Below is an example of a Python script demonstrating Gradient Boosting Machines (GBM) using the scikit-learn library:
|
||||
|
||||
```python
|
||||
# Importing required libraries
|
||||
from sklearn.datasets import make_classification
|
||||
from sklearn.model_selection import train_test_split
|
||||
from sklearn.ensemble import GradientBoostingClassifier
|
||||
from sklearn.metrics import accuracy_score
|
||||
|
||||
# Generate a random classification dataset
|
||||
X, y = make_classification(n_samples=100, random_state=42)
|
||||
|
||||
# Split the dataset into training and testing sets
|
||||
X_train, X_test, y_train, y_test = train_test_split(X, y, test_size=0.2, random_state=42)
|
||||
|
||||
# Create and fit the Gradient Boosting Classifier
|
||||
gbm_model = GradientBoostingClassifier(random_state=42)
|
||||
gbm_model.fit(X_train, y_train)
|
||||
|
||||
# Predict the labels for the test set
|
||||
y_pred = gbm_model.predict(X_test)
|
||||
|
||||
# Calculate the accuracy score
|
||||
accuracy = accuracy_score(y_test, y_pred)
|
||||
print("Accuracy:", accuracy)
|
||||
```
|
||||
|
||||
In this script, we first generate a random classification dataset using the `make_classification` function from scikit-learn. Then, we split the dataset into training and testing sets using the `train_test_split` function.
|
||||
|
||||
Next, we create an instance of the Gradient Boosting Classifier using `GradientBoostingClassifier` and fit the model to the training data using the `fit` method.
|
||||
|
||||
After fitting the model, we predict the labels for the test set using the `predict` method.
|
||||
|
||||
Finally, we calculate the accuracy score by comparing the predicted labels with the true labels and print it out.
|
@ -1,52 +0,0 @@
|
||||
Sure! Here's a Python script that demonstrates Independent Component Analysis (ICA) using the scikit-learn library:
|
||||
|
||||
```python
|
||||
import numpy as np
|
||||
from sklearn.decomposition import FastICA
|
||||
import matplotlib.pyplot as plt
|
||||
|
||||
# Generate data
|
||||
np.random.seed(0)
|
||||
n_samples = 2000
|
||||
time = np.linspace(0, 8, n_samples)
|
||||
|
||||
s1 = np.sin(2 * time) # Signal 1: sinusoidal signal
|
||||
s2 = np.sign(np.sin(3 * time)) # Signal 2: square signal
|
||||
|
||||
S = np.c_[s1, s2]
|
||||
S += 0.2 * np.random.normal(size=S.shape) # Add noise
|
||||
|
||||
# Mix data
|
||||
A = np.array([[1, 1], [0.5, 2]]) # Mixing matrix
|
||||
X = np.dot(S, A.T) # Generate observations
|
||||
|
||||
# ICA
|
||||
ica = FastICA(n_components=2)
|
||||
S_ = ica.fit_transform(X) # Reconstruct signals
|
||||
A_ = ica.mixing_ # Estimated mixing matrix
|
||||
|
||||
# Plot results
|
||||
plt.figure(figsize=(8, 6))
|
||||
|
||||
models = [X, S, S_]
|
||||
names = ['Observations (mixed signals)',
|
||||
'True Sources',
|
||||
'ICA estimated sources']
|
||||
|
||||
colors = ['red', 'steelblue']
|
||||
|
||||
for i, (model, name) in enumerate(zip(models, names), 1):
|
||||
plt.subplot(3, 1, i)
|
||||
plt.title(name)
|
||||
for sig, color in zip(model.T, colors):
|
||||
plt.plot(sig, color=color)
|
||||
|
||||
plt.tight_layout()
|
||||
plt.show()
|
||||
```
|
||||
|
||||
This script demonstrates ICA by generating two mixed signals, `s1` and `s2`, adding noise, and then applying ICA to separate the original signals. The original signals are represented by the `S` matrix, and the observed mixed signals are stored in the `X` matrix. The `FastICA` class from scikit-learn is used to perform ICA.
|
||||
|
||||
The script plots three subplots: the observed mixed signals, the true sources, and the estimated sources achieved through ICA.
|
||||
|
||||
Make sure to install required dependencies (scikit-learn, numpy, matplotlib) before running the script.
|
@ -1,45 +0,0 @@
|
||||
Sure! Here's an example Python script that demonstrates Latent Dirichlet Allocation (LDA) using the scikit-learn library:
|
||||
|
||||
```python
|
||||
import pandas as pd
|
||||
from sklearn.feature_extraction.text import CountVectorizer
|
||||
from sklearn.decomposition import LatentDirichletAllocation
|
||||
|
||||
# Sample data
|
||||
documents = [
|
||||
'apple orange apple orange',
|
||||
'banana apple orange',
|
||||
'orange banana apple',
|
||||
'apple apple apple',
|
||||
'orange orange',
|
||||
]
|
||||
|
||||
# Tokenize and vectorize the documents
|
||||
vectorizer = CountVectorizer()
|
||||
X = vectorizer.fit_transform(documents)
|
||||
|
||||
# Apply LDA
|
||||
lda_model = LatentDirichletAllocation(n_components=2, random_state=0)
|
||||
lda_model.fit(X)
|
||||
|
||||
# Get the topics and their corresponding word probabilities
|
||||
feature_names = vectorizer.get_feature_names_out()
|
||||
topics = lda_model.components_
|
||||
|
||||
# Display the top words for each topic
|
||||
for topic_idx, topic in enumerate(topics):
|
||||
top_words = [feature_names[i] for i in topic.argsort()[:-4:-1]]
|
||||
print(f"Top words for topic {topic_idx+1}: {', '.join(top_words)}")
|
||||
```
|
||||
|
||||
In this script, we start by importing the necessary libraries: pandas for data manipulation, CountVectorizer for tokenization and vectorization of text documents, and LatentDirichletAllocation from sklearn.decomposition for performing LDA.
|
||||
|
||||
Next, we define a sample dataset of text documents. You can replace this with your own dataset.
|
||||
|
||||
We then use the CountVectorizer to transform the documents into a matrix representation with token counts. This matrix is then passed to the LatentDirichletAllocation model for training. In this example, we have specified `n_components=2` to indicate that we want to extract two topics.
|
||||
|
||||
After fitting the LDA model, we retrieve the feature names and the LDA model's components (topics and word probabilities).
|
||||
|
||||
Finally, we loop through each topic to display the top words with the highest probabilities for each topic.
|
||||
|
||||
Note that the results may vary each time you run the script due to the random nature of LDA.
|
@ -1,121 +0,0 @@
|
||||
Sure! Here's a Python script that demonstrates the Monte Carlo Tree Search (MCTS) algorithm:
|
||||
|
||||
```python
|
||||
import numpy as np
|
||||
import random
|
||||
|
||||
class Node:
|
||||
def __init__(self, state, parent=None):
|
||||
self.state = state
|
||||
self.parent = parent
|
||||
self.children = []
|
||||
self.visits = 0
|
||||
self.rewards = 0
|
||||
|
||||
def expand(self):
|
||||
possible_moves = self.state.get_possible_moves()
|
||||
for move in possible_moves:
|
||||
new_state = self.state.make_move(move)
|
||||
new_node = Node(new_state, parent=self)
|
||||
self.children.append(new_node)
|
||||
|
||||
def select(self):
|
||||
selected_child = max(self.children, key=lambda child: child.get_ucb_score())
|
||||
return selected_child
|
||||
|
||||
def simulate(self):
|
||||
current_state = self.state
|
||||
while not current_state.is_terminal():
|
||||
random_move = random.choice(current_state.get_possible_moves())
|
||||
current_state = current_state.make_move(random_move)
|
||||
return current_state.get_reward()
|
||||
|
||||
def backpropagate(self, reward):
|
||||
self.visits += 1
|
||||
self.rewards += reward
|
||||
if self.parent:
|
||||
self.parent.backpropagate(reward)
|
||||
|
||||
def get_ucb_score(self):
|
||||
exploration_factor = 1.414 # Adjust this for exploration vs exploitation trade-off
|
||||
exploitation_score = self.rewards / self.visits
|
||||
exploration_score = np.sqrt(np.log(self.parent.visits) / self.visits)
|
||||
return exploitation_score + exploration_factor * exploration_score
|
||||
|
||||
|
||||
class State:
|
||||
def __init__(self):
|
||||
self.board = np.zeros((3, 3))
|
||||
self.current_player = 1
|
||||
|
||||
def get_possible_moves(self):
|
||||
return [(i, j) for i in range(3) for j in range(3) if self.board[i][j] == 0]
|
||||
|
||||
def make_move(self, move):
|
||||
new_state = State()
|
||||
new_state.board = np.copy(self.board)
|
||||
new_state.current_player = -self.current_player
|
||||
new_state.board[move[0]][move[1]] = self.current_player
|
||||
return new_state
|
||||
|
||||
def is_terminal(self):
|
||||
return np.any(np.sum(self.board, axis=1) == 3) or np.any(np.sum(self.board, axis=0) == 3) \
|
||||
or np.trace(self.board) == 3 or np.trace(np.fliplr(self.board)) == 3 \
|
||||
or np.any(np.sum(self.board, axis=1) == -3) or np.any(np.sum(self.board, axis=0) == -3) \
|
||||
or np.trace(self.board) == -3 or np.trace(np.fliplr(self.board)) == -3 \
|
||||
or len(self.get_possible_moves()) == 0
|
||||
|
||||
def get_reward(self):
|
||||
if np.any(np.sum(self.board, axis=1) == 3) or np.any(np.sum(self.board, axis=0) == 3) \
|
||||
or np.trace(self.board) == 3 or np.trace(np.fliplr(self.board)) == 3:
|
||||
return 1
|
||||
elif np.any(np.sum(self.board, axis=1) == -3) or np.any(np.sum(self.board, axis=0) == -3) \
|
||||
or np.trace(self.board) == -3 or np.trace(np.fliplr(self.board)) == -3:
|
||||
return -1
|
||||
else:
|
||||
return 0
|
||||
|
||||
|
||||
def monte_carlo_tree_search(initial_state, iterations):
|
||||
root = Node(initial_state)
|
||||
|
||||
for _ in range(iterations):
|
||||
# Selection
|
||||
selected_node = root
|
||||
while selected_node.children:
|
||||
selected_node = selected_node.select()
|
||||
|
||||
# Expansion
|
||||
if not selected_node.state.is_terminal():
|
||||
selected_node.expand()
|
||||
selected_node = random.choice(selected_node.children)
|
||||
|
||||
# Simulation
|
||||
reward = selected_node.simulate()
|
||||
|
||||
# Backpropagation
|
||||
selected_node.backpropagate(reward)
|
||||
|
||||
best_child = max(root.children, key=lambda child: child.visits)
|
||||
return best_child.state.board
|
||||
|
||||
|
||||
# Test the Monte Carlo Tree Search algorithm on Tic-Tac-Toe game
|
||||
|
||||
initial_state = State()
|
||||
|
||||
best_move = monte_carlo_tree_search(initial_state, iterations=10000)
|
||||
|
||||
print("Best move found by Monte Carlo Tree Search:")
|
||||
print(best_move)
|
||||
```
|
||||
|
||||
In the above script, we define a `Node` class to represent each state in the game and a `State` class to maintain the current game state. The `monte_carlo_tree_search` function implements the MCTS algorithm and returns the best move found after the specified number of iterations.
|
||||
|
||||
In the Tic-Tac-Toe game example, each state is represented by a 3x3 game board. The `is_terminal` method checks if the game is over, and the `get_reward` method assigns rewards to terminal states (-1 for loss, 1 for win, 0 for draw). The `get_possible_moves` method returns all valid moves for the current state, and the `make_move` method creates a new state after making a move.
|
||||
|
||||
During each iteration of the MCTS algorithm, the `selection` step selects the most promising child node by applying the Upper Confidence Bound (UCB) formula. If the selected node is not terminal, the `expansion` step creates child nodes by simulating all possible moves. The `simulation` step randomly plays out the game from the selected child node until a terminal state is reached. Finally, the `backpropagation` step updates all nodes in the selected path with the simulation result. The process is repeated for the specified number of iterations.
|
||||
|
||||
After running the MCTS algorithm, the best move is determined by selecting the child node with the highest visit count from the root node. The resulting board configuration is returned as the best move.
|
||||
|
||||
Feel free to adjust the number of iterations and exploration factor to see different results.
|
@ -1,42 +0,0 @@
|
||||
Sure! The following is a python script that demonstrates the Naïve Bayes algorithm using the famous Iris dataset:
|
||||
|
||||
```python
|
||||
import numpy as np
|
||||
from sklearn.datasets import load_iris
|
||||
from sklearn.model_selection import train_test_split
|
||||
from sklearn.naive_bayes import GaussianNB
|
||||
from sklearn.metrics import accuracy_score
|
||||
|
||||
# Load the Iris dataset
|
||||
iris = load_iris()
|
||||
|
||||
# Split the dataset into training and testing sets
|
||||
X_train, X_test, y_train, y_test = train_test_split(iris.data, iris.target, test_size=0.2, random_state=42)
|
||||
|
||||
# Create an instance of the Naïve Bayes classifier
|
||||
classifier = GaussianNB()
|
||||
|
||||
# Train the classifier using the training data
|
||||
classifier.fit(X_train, y_train)
|
||||
|
||||
# Make predictions on the testing data
|
||||
y_pred = classifier.predict(X_test)
|
||||
|
||||
# Calculate accuracy of the model
|
||||
accuracy = accuracy_score(y_test, y_pred)
|
||||
print("Accuracy:", accuracy)
|
||||
```
|
||||
|
||||
In this script, we start by importing the necessary libraries: `numpy` for numerical operations, `sklearn.datasets` to load the Iris dataset, `sklearn.model_selection` to split the data into training and testing sets, `sklearn.naive_bayes` for the Naïve Bayes classifier, and `sklearn.metrics` for calculating accuracy.
|
||||
|
||||
Next, we load the Iris dataset using `load_iris()` function. Then we split the data into training and testing sets using `train_test_split()` function, where `test_size=0.2` indicates that 20% of the data will be used for testing.
|
||||
|
||||
We create an instance of the Naïve Bayes classifier using `GaussianNB()`. This classifier assumes that features follow a Gaussian distribution. If your data doesn't meet this assumption, you can explore other variants like multinomial or Bernoulli Naïve Bayes.
|
||||
|
||||
We train the classifier using the training data by calling the `fit()` method and passing in the features (X_train) and corresponding labels (y_train).
|
||||
|
||||
Then, we make predictions on the testing data using the `predict()` method and passing in the features of the test set (X_test).
|
||||
|
||||
Finally, we calculate the accuracy of the classifier by comparing the predicted labels with the true labels from the testing set using the `accuracy_score()` function.
|
||||
|
||||
Hope this helps to demonstrate the Naïve Bayes algorithm in python!
|
@ -1,63 +0,0 @@
|
||||
import numpy as np
|
||||
import matplotlib.pyplot as plt
|
||||
|
||||
# Create a simple neural network with one input layer, one hidden layer, and one output layer
|
||||
class NeuralNetwork:
|
||||
def __init__(self):
|
||||
self.weights1 = np.random.rand(3, 4) # weight matrix between input and hidden layer
|
||||
self.weights2 = np.random.rand(4, 1) # weight matrix between hidden and output layer
|
||||
self.bias1 = np.random.rand(1, 4) # bias matrix for hidden layer
|
||||
self.bias2 = np.random.rand(1, 1) # bias matrix for output layer
|
||||
|
||||
def sigmoid(self, x):
|
||||
# Sigmoid activation function
|
||||
return 1 / (1 + np.exp(-x))
|
||||
|
||||
def forward_propagation(self, X):
|
||||
# Perform forward propagation
|
||||
self.hidden_layer = self.sigmoid(np.dot(X, self.weights1) + self.bias1) # calculate hidden layer activations
|
||||
self.output_layer = self.sigmoid(np.dot(self.hidden_layer, self.weights2) + self.bias2) # calculate output layer activations
|
||||
return self.output_layer
|
||||
|
||||
def backward_propagation(self, X, y, output):
|
||||
# Perform backward propagation to update weights and biases
|
||||
self.error = y - output # calculate error
|
||||
self.delta_output = self.error * (output * (1 - output)) # calculate output gradient
|
||||
self.delta_hidden = np.dot(self.delta_output, self.weights2.T) * (self.hidden_layer * (1 - self.hidden_layer)) # calculate hidden gradient
|
||||
self.weights2 += np.dot(self.hidden_layer.T, self.delta_output) # update weights between hidden and output layer
|
||||
self.weights1 += np.dot(X.T, self.delta_hidden) # update weights between input and hidden layer
|
||||
self.bias2 += np.sum(self.delta_output, axis=0) # update bias for output layer
|
||||
self.bias1 += np.sum(self.delta_hidden, axis=0) # update bias for hidden layer
|
||||
|
||||
def train(self, X, y, epochs):
|
||||
# Train the neural network
|
||||
for _ in range(epochs):
|
||||
output = self.forward_propagation(X)
|
||||
self.backward_propagation(X, y, output)
|
||||
|
||||
def predict(self, X):
|
||||
# Make predictions
|
||||
return self.forward_propagation(X)
|
||||
|
||||
# Create a sample dataset for XOR gate
|
||||
X = np.array([[0, 0], [0, 1], [1, 0], [1, 1]])
|
||||
y = np.array([[0], [1], [1], [0]])
|
||||
|
||||
# Create and train the neural network
|
||||
nn = NeuralNetwork()
|
||||
nn.train(X, y, epochs=10000)
|
||||
|
||||
# Make predictions on the same dataset
|
||||
predictions = nn.predict(X)
|
||||
|
||||
# Print the predictions
|
||||
print("Predictions:")
|
||||
for i in range(len(predictions)):
|
||||
print(f"Input: {X[i]}, Predicted Output: {predictions[i]}")
|
||||
|
||||
# Plot the predictions
|
||||
plt.scatter(X[:, 0], X[:, 1], c=predictions.flatten(), cmap='viridis')
|
||||
plt.xlabel("Input 1")
|
||||
plt.ylabel("Input 2")
|
||||
plt.title("Neural Network Predictions for XOR Gate")
|
||||
plt.show()
|
@ -1,91 +0,0 @@
|
||||
Sure! Here's an example of a Python script that demonstrates the concept of Policy Gradients using the OpenAI Gym environment and a simple neural network:
|
||||
|
||||
```python
|
||||
import gym
|
||||
import numpy as np
|
||||
from keras.models import Sequential
|
||||
from keras.layers import Dense
|
||||
|
||||
# Create the policy network
|
||||
def build_policy_network(input_shape, output_units):
|
||||
model = Sequential()
|
||||
model.add(Dense(24, activation='relu', input_shape=input_shape))
|
||||
model.add(Dense(24, activation='relu'))
|
||||
model.add(Dense(output_units, activation='softmax'))
|
||||
model.compile(optimizer='adam', loss='categorical_crossentropy')
|
||||
return model
|
||||
|
||||
def choose_action(state, model):
|
||||
state = np.reshape(state, [1, input_shape[0]])
|
||||
prob_dist = model.predict(state).flatten()
|
||||
action = np.random.choice(num_actions, 1, p=prob_dist)[0]
|
||||
return action
|
||||
|
||||
def discount_rewards(rewards, gamma):
|
||||
discounted_rewards = np.zeros_like(rewards)
|
||||
running_sum = 0
|
||||
for t in reversed(range(len(rewards))):
|
||||
running_sum = running_sum * gamma + rewards[t]
|
||||
discounted_rewards[t] = running_sum
|
||||
return discounted_rewards
|
||||
|
||||
# Set hyperparameters
|
||||
learning_rate = 0.01
|
||||
num_episodes = 1000
|
||||
gamma = 0.99
|
||||
|
||||
# Create the environment
|
||||
env = gym.make('CartPole-v0')
|
||||
input_shape = env.observation_space.shape
|
||||
num_actions = env.action_space.n
|
||||
|
||||
# Build the policy network and initialize weights
|
||||
policy_network = build_policy_network(input_shape, num_actions)
|
||||
|
||||
# Start training
|
||||
for episode in range(num_episodes):
|
||||
state = env.reset()
|
||||
done = False
|
||||
episode_rewards = []
|
||||
episode_gradients = []
|
||||
|
||||
while not done:
|
||||
# Choose action based on the policy network
|
||||
action = choose_action(state, policy_network)
|
||||
|
||||
# Take the chosen action and observe the next state and reward
|
||||
next_state, reward, done, _ = env.step(action)
|
||||
|
||||
# Store the reward
|
||||
episode_rewards.append(reward)
|
||||
|
||||
# Compute the one-hot encoded action
|
||||
action_onehot = np.zeros(num_actions)
|
||||
action_onehot[action] = 1
|
||||
|
||||
# Compute the gradient of the policy network's output w.r.t. the action taken
|
||||
with tf.GradientTape() as tape:
|
||||
logits = policy_network.predict(np.expand_dims(state, axis=0))
|
||||
loss = tf.reduce_sum(tf.multiply(logits, tf.convert_to_tensor(action_onehot, dtype=tf.float32)))
|
||||
|
||||
# Store the gradients
|
||||
episode_gradients.append(tape.gradient(loss, policy_network.trainable_variables))
|
||||
|
||||
state = next_state
|
||||
|
||||
# Update the policy network
|
||||
rewards = discount_rewards(episode_rewards, gamma)
|
||||
for i in range(len(episode_gradients)):
|
||||
grads = episode_gradients[i]
|
||||
for j in range(len(grads)):
|
||||
policy_network.trainable_variables[j].assign_sub(learning_rate * grads[j] * rewards[i])
|
||||
|
||||
if episode % 100 == 0:
|
||||
print("Episode {}: Average reward = {}".format(episode, np.mean(episode_rewards)))
|
||||
```
|
||||
|
||||
This script uses the `gym` package to create the CartPole-v0 environment, which is a classic reinforcement learning problem. It then builds a simple neural network as the policy network, with two hidden layers and a softmax output layer for the action probabilities. It implements the `choose_action` function to sample actions based on the probabilities predicted by the policy network.
|
||||
|
||||
During training, the script collects rewards and gradients for each episode. It then applies the policy gradient update rule, computing the discounted rewards and updating the policy network weights accordingly. Finally, it prints the average reward for every 100 episodes.
|
||||
|
||||
Note that this script is a simplified example and may not converge to the optimal policy. Policy Gradients typically require careful tuning of hyperparameters, selecting suitable network architectures, and using more advanced techniques like baseline estimation and advantage normalization to improve the training stability and performance.
|
@ -1,36 +0,0 @@
|
||||
Certainly! Here's a Python script that demonstrates Principal Component Analysis (PCA):
|
||||
|
||||
```python
|
||||
import numpy as np
|
||||
import matplotlib.pyplot as plt
|
||||
from sklearn.decomposition import PCA
|
||||
|
||||
# Generate some random 2D data points
|
||||
np.random.seed(0)
|
||||
n_samples = 100
|
||||
X = np.random.randn(n_samples, 2) * 2
|
||||
|
||||
# Apply PCA
|
||||
pca = PCA(n_components=2)
|
||||
X_pca = pca.fit_transform(X)
|
||||
|
||||
# Plot the original data
|
||||
plt.figure(figsize=(8, 4))
|
||||
plt.subplot(1, 2, 1)
|
||||
plt.scatter(X[:, 0], X[:, 1], alpha=0.5)
|
||||
plt.title('Original Data')
|
||||
plt.xlabel('X')
|
||||
plt.ylabel('Y')
|
||||
|
||||
# Plot the PCA-transformed data
|
||||
plt.subplot(1, 2, 2)
|
||||
plt.scatter(X_pca[:, 0], X_pca[:, 1], alpha=0.5)
|
||||
plt.title('PCA Transformed Data')
|
||||
plt.xlabel('Principal Component 1')
|
||||
plt.ylabel('Principal Component 2')
|
||||
|
||||
plt.tight_layout()
|
||||
plt.show()
|
||||
```
|
||||
|
||||
In this script, we generate a random 2D dataset using `np.random.randn()`. We then apply PCA using `sklearn.decomposition.PCA` and transform the data using `fit_transform()`. Finally, we visualize the original data and the PCA-transformed data in separate subplots using `matplotlib.pyplot.scatter()`.
|
@ -1,160 +0,0 @@
|
||||
Sure! Here's a Python script that demonstrates Proximal Policy Optimization (PPO) using the OpenAI Gym's CartPole environment:
|
||||
|
||||
```python
|
||||
import gym
|
||||
import numpy as np
|
||||
import tensorflow as tf
|
||||
from tensorflow import keras
|
||||
from tensorflow.keras import layers
|
||||
|
||||
# Set up the CartPole environment
|
||||
env = gym.make("CartPole-v1")
|
||||
num_states = env.observation_space.shape[0]
|
||||
num_actions = env.action_space.n
|
||||
|
||||
# PPO Agent
|
||||
class PPOAgent:
|
||||
def __init__(self, num_states, num_actions):
|
||||
self.gamma = 0.99 # Discount factor
|
||||
self.epsilon = 0.2 # Clipping factor
|
||||
self.actor_lr = 0.0003 # Actor learning rate
|
||||
self.critic_lr = 0.001 # Critic learning rate
|
||||
|
||||
self.actor = self.build_actor()
|
||||
self.critic = self.build_critic()
|
||||
|
||||
def build_actor(self):
|
||||
inputs = layers.Input(shape=(num_states,))
|
||||
hidden = layers.Dense(128, activation="relu")(inputs)
|
||||
action_probs = layers.Dense(num_actions, activation="softmax")(hidden)
|
||||
|
||||
model = keras.Model(inputs=inputs, outputs=action_probs)
|
||||
optimizer = tf.keras.optimizers.Adam(learning_rate=self.actor_lr)
|
||||
model.compile(optimizer=optimizer, loss="categorical_crossentropy")
|
||||
return model
|
||||
|
||||
def build_critic(self):
|
||||
inputs = layers.Input(shape=(num_states,))
|
||||
hidden = layers.Dense(128, activation="relu")(inputs)
|
||||
value = layers.Dense(1, activation="linear")(hidden)
|
||||
|
||||
model = keras.Model(inputs=inputs, outputs=value)
|
||||
optimizer = tf.keras.optimizers.Adam(learning_rate=self.critic_lr)
|
||||
model.compile(optimizer=optimizer, loss="mean_squared_error")
|
||||
return model
|
||||
|
||||
def choose_action(self, state):
|
||||
state = np.expand_dims(state, axis=0)
|
||||
action_probs = self.actor.predict(state).flatten()
|
||||
|
||||
# Sample an action from the action probability distribution
|
||||
action = np.random.choice(num_actions, 1, p=action_probs)[0]
|
||||
return action
|
||||
|
||||
def compute_returns(self, rewards, dones, values):
|
||||
returns = np.zeros_like(rewards)
|
||||
discounted_sum = 0
|
||||
for i in reversed(range(len(rewards))):
|
||||
if dones[i]:
|
||||
discounted_sum = 0
|
||||
discounted_sum = rewards[i] + self.gamma * discounted_sum
|
||||
returns[i] = discounted_sum
|
||||
|
||||
advantages = returns - values
|
||||
advantages = (advantages - np.mean(advantages)) / (np.std(advantages) + 1e-10)
|
||||
return returns, advantages
|
||||
|
||||
def train(self, old_states, actions, rewards, dones, values):
|
||||
returns, advantages = self.compute_returns(rewards, dones, values)
|
||||
|
||||
# Convert inputs to numpy arrays for better indexing
|
||||
old_states = np.array(old_states)
|
||||
actions = np.array(actions)
|
||||
returns = np.array(returns)
|
||||
advantages = np.array(advantages)
|
||||
|
||||
num_samples = len(old_states)
|
||||
|
||||
# Actor training
|
||||
actions_one_hot = np.eye(num_actions)[actions]
|
||||
old_action_probs = self.actor.predict(old_states)
|
||||
old_action_probs = np.clip(old_action_probs, 1e-10, 1.0)
|
||||
old_action_probs = old_action_probs * actions_one_hot
|
||||
old_action_probs = np.sum(old_action_probs, axis=1)
|
||||
|
||||
with tf.GradientTape() as tape:
|
||||
new_action_probs = self.actor(old_states, training=True)
|
||||
new_action_probs = np.clip(new_action_probs, 1e-10, 1.0)
|
||||
new_action_probs = new_action_probs * actions_one_hot
|
||||
new_action_probs = np.sum(new_action_probs, axis=1)
|
||||
|
||||
ratio = new_action_probs / old_action_probs
|
||||
|
||||
surrogate1 = ratio * advantages
|
||||
surrogate2 = np.clip(ratio, 1 - self.epsilon, 1 + self.epsilon) * advantages
|
||||
actor_loss = -tf.reduce_mean(tf.minimum(surrogate1, surrogate2))
|
||||
|
||||
actor_grads = tape.gradient(actor_loss, self.actor.trainable_variables)
|
||||
self.actor.optimizer.apply_gradients(zip(actor_grads, self.actor.trainable_variables))
|
||||
|
||||
# Critic training
|
||||
with tf.GradientTape() as tape:
|
||||
values_pred = self.critic(old_states, training=True)
|
||||
critic_loss = tf.reduce_mean(tf.square(returns - values_pred))
|
||||
|
||||
critic_grads = tape.gradient(critic_loss, self.critic.trainable_variables)
|
||||
self.critic.optimizer.apply_gradients(zip(critic_grads, self.critic.trainable_variables))
|
||||
|
||||
# Initialize PPO agent
|
||||
agent = PPOAgent(num_states, num_actions)
|
||||
|
||||
# Training loop
|
||||
num_episodes = 500
|
||||
for episode in range(num_episodes):
|
||||
state = env.reset()
|
||||
done = False
|
||||
|
||||
old_states = []
|
||||
actions = []
|
||||
rewards = []
|
||||
dones = []
|
||||
values = []
|
||||
|
||||
while not done:
|
||||
# Collect data
|
||||
action = agent.choose_action(state)
|
||||
next_state, reward, done, _ = env.step(action)
|
||||
|
||||
old_states.append(state)
|
||||
actions.append(action)
|
||||
rewards.append(reward)
|
||||
dones.append(done)
|
||||
values.append(agent.critic.predict(np.expand_dims(state, axis=0)))
|
||||
|
||||
state = next_state
|
||||
|
||||
# Train the agent
|
||||
agent.train(old_states, actions, rewards, dones, values)
|
||||
|
||||
# Display rewards every 10 episodes
|
||||
if episode % 10 == 0:
|
||||
total_rewards = sum(rewards)
|
||||
print(f"Episode: {episode}, Rewards: {total_rewards}")
|
||||
|
||||
# Test the trained agent
|
||||
state = env.reset()
|
||||
done = False
|
||||
total_rewards = 0
|
||||
|
||||
while not done:
|
||||
env.render()
|
||||
action = agent.choose_action(state)
|
||||
state, reward, done, _ = env.step(action)
|
||||
total_rewards += reward
|
||||
|
||||
print(f"Total Rewards: {total_rewards}")
|
||||
|
||||
env.close()
|
||||
```
|
||||
|
||||
Make sure you have the OpenAI Gym package installed (`pip install gym`) and run the script. It will train a PPO agent on the CartPole-v1 environment and then test the trained agent. You should see the total rewards increasing as the agent learns to balance the pole on the cart.
|
@ -1,34 +0,0 @@
|
||||
Sure! Here's a python script that demonstrates the concept of Random Forests using the famous Iris dataset.
|
||||
|
||||
```python
|
||||
# Importing the required libraries
|
||||
from sklearn.ensemble import RandomForestClassifier
|
||||
from sklearn.datasets import load_iris
|
||||
from sklearn.model_selection import train_test_split
|
||||
from sklearn.metrics import accuracy_score
|
||||
|
||||
# Loading the Iris dataset
|
||||
iris = load_iris()
|
||||
X = iris.data
|
||||
y = iris.target
|
||||
|
||||
# Splitting the dataset into training and testing sets
|
||||
X_train, X_test, y_train, y_test = train_test_split(X, y, test_size=0.2, random_state=42)
|
||||
|
||||
# Creating a Random Forest classifier
|
||||
rf_classifier = RandomForestClassifier(n_estimators=100)
|
||||
|
||||
# Training the classifier
|
||||
rf_classifier.fit(X_train, y_train)
|
||||
|
||||
# Predicting on the test set
|
||||
y_pred = rf_classifier.predict(X_test)
|
||||
|
||||
# Calculating accuracy
|
||||
accuracy = accuracy_score(y_test, y_pred)
|
||||
print("Accuracy:", accuracy)
|
||||
```
|
||||
|
||||
In this script, we import the necessary libraries, load the Iris dataset, and split it into training and testing sets. Then, we create a Random Forest classifier with 100 decision trees (`n_estimators=100`) and train it on the training set. Finally, we predict the target variable for the test set and calculate the accuracy of the classifier.
|
||||
|
||||
Note that you may need to install scikit-learn (`pip install scikit-learn`) if you don't have it already installed.
|
@ -1,112 +0,0 @@
|
||||
Sure! Below is an example script that demonstrates the SARSA (State-Action-Reward-State-Action) algorithm using a simple grid world environment:
|
||||
|
||||
```python
|
||||
import numpy as np
|
||||
|
||||
# Define the grid world environment
|
||||
grid = [
|
||||
[0, 0, 0, 0],
|
||||
[0, -1, 0, -1],
|
||||
[0, 0, 0, -1],
|
||||
[0, -1, 0, 1]
|
||||
]
|
||||
|
||||
# Initialize Q-table
|
||||
Q = np.zeros((4, 4, 4)) # Q(s, a) - 4x4 grid, 4 actions (up, down, left, right)
|
||||
|
||||
# Define epsilon-greedy exploration
|
||||
epsilon = 0.1
|
||||
|
||||
# Define learning parameters
|
||||
alpha = 0.1 # Learning rate
|
||||
gamma = 0.9 # Discount factor
|
||||
|
||||
# Define action mapping
|
||||
actions = ['up', 'down', 'left', 'right']
|
||||
|
||||
# Get next action using epsilon-greedy exploration
|
||||
def get_action(state):
|
||||
if np.random.rand() < epsilon:
|
||||
action = np.random.choice(actions)
|
||||
else:
|
||||
action = actions[np.argmax(Q[state[0], state[1]])]
|
||||
return action
|
||||
|
||||
# Update Q-values using SARSA algorithm
|
||||
def update_q_values(state, action, reward, next_state, next_action):
|
||||
Q[state[0], state[1], actions.index(action)] += alpha * (
|
||||
reward + gamma * Q[next_state[0], next_state[1], actions.index(next_action)] -
|
||||
Q[state[0], state[1], actions.index(action)])
|
||||
|
||||
# Train the agent
|
||||
def train_agent():
|
||||
num_episodes = 1000
|
||||
|
||||
for episode in range(num_episodes):
|
||||
state = [3, 0] # Start state
|
||||
action = get_action(state)
|
||||
|
||||
while True:
|
||||
# Perform selected action
|
||||
if action == 'up':
|
||||
next_state = [state[0] - 1, state[1]]
|
||||
elif action == 'down':
|
||||
next_state = [state[0] + 1, state[1]]
|
||||
elif action == 'left':
|
||||
next_state = [state[0], state[1] - 1]
|
||||
else:
|
||||
next_state = [state[0], state[1] + 1]
|
||||
|
||||
# Check if next state is valid
|
||||
if next_state[0] < 0 or next_state[0] >= 4 or next_state[1] < 0 or next_state[1] >= 4:
|
||||
next_state = state
|
||||
|
||||
# Get next action using epsilon-greedy exploration
|
||||
next_action = get_action(next_state)
|
||||
|
||||
# Update Q-values
|
||||
update_q_values(state, action, grid[next_state[0]][next_state[1]], next_state, next_action)
|
||||
|
||||
# Update current state and action
|
||||
state = next_state
|
||||
action = next_action
|
||||
|
||||
# Break if goal state reached
|
||||
if grid[state[0]][state[1]] == 1:
|
||||
break
|
||||
|
||||
# Test the trained agent
|
||||
def test_agent():
|
||||
state = [3, 0] # Start state
|
||||
|
||||
while True:
|
||||
# Choose the best action based on Q-values
|
||||
action = actions[np.argmax(Q[state[0], state[1]])]
|
||||
|
||||
# Perform selected action
|
||||
if action == 'up':
|
||||
next_state = [state[0] - 1, state[1]]
|
||||
elif action == 'down':
|
||||
next_state = [state[0] + 1, state[1]]
|
||||
elif action == 'left':
|
||||
next_state = [state[0], state[1] - 1]
|
||||
else:
|
||||
next_state = [state[0], state[1] + 1]
|
||||
|
||||
# Print the current state and action taken
|
||||
print(f"Current state: {state}, Action: {action}")
|
||||
|
||||
# Update current state
|
||||
state = next_state
|
||||
|
||||
# Break if goal state reached
|
||||
if grid[state[0]][state[1]] == 1:
|
||||
print("Reached the goal!")
|
||||
break
|
||||
|
||||
# Train and test the agent
|
||||
train_agent()
|
||||
test_agent()
|
||||
```
|
||||
|
||||
This script demonstrates SARSA algorithm in a simple grid world environment, where the agent has to navigate from the starting state `[3, 0]` to the goal state `[3, 3]` while avoiding obstacles represented by `-1`. The agent uses the SARSA algorithm to learn optimal Q-values and then applies them to reach the goal state.
|
@ -1,38 +0,0 @@
|
||||
Sure! Here's an example script demonstrating the use of Support Vector Machines (SVM) for a binary classification problem using the famous iris dataset:
|
||||
|
||||
```python
|
||||
# Importing the required libraries
|
||||
from sklearn import datasets
|
||||
from sklearn.model_selection import train_test_split
|
||||
from sklearn.svm import SVC
|
||||
from sklearn.metrics import accuracy_score
|
||||
|
||||
# Loading the iris dataset
|
||||
iris = datasets.load_iris()
|
||||
X = iris.data
|
||||
y = iris.target
|
||||
|
||||
# Splitting the dataset into training and testing sets
|
||||
X_train, X_test, y_train, y_test = train_test_split(X, y, test_size=0.3, random_state=42)
|
||||
|
||||
# Creating the SVM classifier
|
||||
svm = SVC(kernel='linear')
|
||||
|
||||
# Training the SVM classifier
|
||||
svm.fit(X_train, y_train)
|
||||
|
||||
# Making predictions on the testing set
|
||||
y_pred = svm.predict(X_test)
|
||||
|
||||
# Calculating the accuracy of the model
|
||||
accuracy = accuracy_score(y_test, y_pred)
|
||||
print(f"Accuracy: {accuracy}")
|
||||
```
|
||||
|
||||
In this script, the iris dataset is first loaded using the `datasets.load_iris()` method from the sklearn library. The features matrix `X` and the target vector `y` are then initialized. Next, the dataset is split into training and testing sets using `train_test_split()` function from the `model_selection` module.
|
||||
|
||||
A Support Vector Machine classifier is created using `SVC()` class from the `svm` module, with the `kernel` parameter set to 'linear'. The classifier is then trained on the training set using the `fit()` method.
|
||||
|
||||
Predictions are made on the testing set using the `predict()` method, and the accuracy of the model is calculated using the `accuracy_score()` function from the `metrics` module. Finally, the accuracy is printed to the console.
|
||||
|
||||
You can modify this script according to your specific use case or dataset. Remember to install the required packages (`scikit-learn`) in your Python environment before running the script.
|
@ -1,65 +0,0 @@
|
||||
Sure! Here's a simple example of a Python script that demonstrates Temporal Difference Learning (TD Learning) using a simple grid world environment:
|
||||
|
||||
```python
|
||||
import numpy as np
|
||||
|
||||
# Environment
|
||||
grid_size = 4
|
||||
num_episodes = 100
|
||||
start_state = (0, 0)
|
||||
end_state = (grid_size - 1, grid_size - 1)
|
||||
actions = ['up', 'down', 'left', 'right']
|
||||
|
||||
# Hyperparameters
|
||||
alpha = 0.1 # learning rate
|
||||
gamma = 0.9 # discount factor
|
||||
|
||||
# Initialize state-action value function
|
||||
Q = np.zeros((grid_size, grid_size, len(actions)))
|
||||
|
||||
# Helper function to choose an action based on Q-values (epsilon-greedy policy)
|
||||
def choose_action(state, epsilon):
|
||||
if np.random.random() < epsilon:
|
||||
return np.random.choice(actions)
|
||||
return actions[np.argmax(Q[state])]
|
||||
|
||||
# Helper function to get the next state and reward based on the chosen action
|
||||
def get_next_state_reward(state, action):
|
||||
if action == 'up':
|
||||
next_state = (state[0] - 1, state[1])
|
||||
elif action == 'down':
|
||||
next_state = (state[0] + 1, state[1])
|
||||
elif action == 'left':
|
||||
next_state = (state[0], state[1] - 1)
|
||||
elif action == 'right':
|
||||
next_state = (state[0], state[1] + 1)
|
||||
|
||||
if next_state[0] < 0 or next_state[0] >= grid_size or next_state[1] < 0 or next_state[1] >= grid_size:
|
||||
# Hit wall, stay in the same state with a negative reward
|
||||
return state, -10
|
||||
elif next_state == end_state:
|
||||
# Reached the end, stay in the same state with a positive reward
|
||||
return state, 10
|
||||
else:
|
||||
return next_state, 0 # Regular move, stay in the same state with no reward
|
||||
|
||||
|
||||
# TD Learning algorithm
|
||||
for episode in range(num_episodes):
|
||||
state = start_state
|
||||
epsilon = 1.0 / (episode + 1) # epsilon-greedy exploration rate
|
||||
|
||||
while state != end_state:
|
||||
action = choose_action(state, epsilon)
|
||||
next_state, reward = get_next_state_reward(state, action)
|
||||
|
||||
# Update Q-values using Temporal Difference Learning
|
||||
Q[state][actions.index(action)] += alpha * (reward + gamma * np.max(Q[next_state]) - Q[state][actions.index(action)])
|
||||
|
||||
state = next_state
|
||||
|
||||
# Print the learned Q-values
|
||||
print(Q)
|
||||
```
|
||||
|
||||
In this script, we define a simple grid world environment with a start state, an end state, and possible actions ('up', 'down', 'left', 'right'). The script then uses the Temporal Difference Learning algorithm to update the state-action values in the Q-table based on the rewards obtained from interactions with the environment. Finally, it prints the learned Q-values.
|
@ -1,213 +0,0 @@
|
||||
Sure! Below is an example Python script that demonstrates the Trust Region Policy Optimization (TRPO) algorithm using OpenAI Gym's "CartPole-v1" environment:
|
||||
|
||||
```python
|
||||
import gym
|
||||
import numpy as np
|
||||
import tensorflow as tf
|
||||
|
||||
|
||||
class PolicyNetwork:
|
||||
def __init__(self, state_dim, action_dim, hidden_dim):
|
||||
self.state_dim = state_dim
|
||||
self.action_dim = action_dim
|
||||
self.hidden_dim = hidden_dim
|
||||
|
||||
self.states = tf.placeholder(tf.float32, [None, state_dim], name="states")
|
||||
self.actions = tf.placeholder(tf.int32, [None], name="actions")
|
||||
self.advantages = tf.placeholder(tf.float32, [None], name="advantages")
|
||||
|
||||
self.mean_network = self.build_network(scope="mean")
|
||||
self.sample_network = self.build_network(scope="sample")
|
||||
|
||||
self.sampled_actions = self.sample_network(self.states)
|
||||
|
||||
self.mean_weights = tf.get_collection(tf.GraphKeys.GLOBAL_VARIABLES, scope="mean")
|
||||
self.sample_weights = tf.get_collection(tf.GraphKeys.GLOBAL_VARIABLES, scope="sample")
|
||||
|
||||
self.policy_loss = self.compute_policy_loss()
|
||||
self.kl_divergence = self.compute_kl_divergence()
|
||||
self.gradient = self.compute_gradient()
|
||||
|
||||
def build_network(self, scope):
|
||||
with tf.variable_scope(scope):
|
||||
hidden_layer = tf.layers.dense(self.states, self.hidden_dim, activation=tf.nn.relu)
|
||||
output_layer = tf.layers.dense(hidden_layer, self.action_dim)
|
||||
output_probs = tf.nn.softmax(output_layer)
|
||||
|
||||
def network(states):
|
||||
feed_dict = {self.states: states}
|
||||
sess = tf.get_default_session()
|
||||
return sess.run(output_probs, feed_dict=feed_dict)
|
||||
|
||||
return network
|
||||
|
||||
def compute_policy_loss(self):
|
||||
indices = tf.range(tf.shape(self.sampled_actions)[0]) * tf.shape(self.sampled_actions)[1] + self.actions
|
||||
selected_action_probs = tf.gather(tf.reshape(self.sampled_actions, [-1]), indices)
|
||||
ratio = selected_action_probs / tf.stop_gradient(self.mean_network(self.states))
|
||||
surrogate_loss = -tf.reduce_mean(ratio * self.advantages)
|
||||
return surrogate_loss
|
||||
|
||||
def compute_kl_divergence(self):
|
||||
mean_network_probs = self.mean_network(self.states)
|
||||
sample_network_probs = tf.stop_gradient(self.sampled_actions)
|
||||
return tf.reduce_mean(tf.reduce_sum(mean_network_probs * tf.log(mean_network_probs / sample_network_probs), axis=1))
|
||||
|
||||
def compute_gradient(self):
|
||||
grads = tf.gradients(self.policy_loss, self.sample_weights)
|
||||
flat_grads = tf.concat([tf.reshape(grad, [-1]) for grad in grads], axis=0)
|
||||
return flat_grads
|
||||
|
||||
|
||||
def compute_advantages(rewards, next_value, discount_factor=0.99, gae_lambda=0.95):
|
||||
values = np.append(rewards, next_value)
|
||||
deltas = rewards + discount_factor * values[1:] - values[:-1]
|
||||
advantages = np.zeros_like(rewards)
|
||||
for t in reversed(range(len(rewards))):
|
||||
delta = deltas[t]
|
||||
advantages[t] = delta + discount_factor * gae_lambda * advantages[t+1]
|
||||
return advantages
|
||||
|
||||
|
||||
def run_episode(env, policy_network, render=False):
|
||||
states, actions, rewards = [], [], []
|
||||
state = env.reset()
|
||||
while True:
|
||||
if render:
|
||||
env.render()
|
||||
action_probs = policy_network.sample_network(np.expand_dims(state, axis=0))
|
||||
action = np.random.choice(len(action_probs[0]), p=action_probs[0])
|
||||
next_state, reward, done, _ = env.step(action)
|
||||
|
||||
states.append(state)
|
||||
actions.append(action)
|
||||
rewards.append(reward)
|
||||
|
||||
state = next_state
|
||||
|
||||
if done:
|
||||
break
|
||||
|
||||
return states, actions, rewards
|
||||
|
||||
|
||||
def train(env, policy_network, max_iterations=1000, max_episode_length=1000, cg_iterations=10, delta=0.01):
|
||||
optimizer = tf.train.AdamOptimizer(learning_rate=1e-3)
|
||||
trainable_variables = tf.trainable_variables()
|
||||
grads_placeholder = tf.placeholder(tf.float32, shape=[None])
|
||||
flat_grads_and_vars_placeholder = tf.placeholder(tf.float32, shape=[None])
|
||||
|
||||
grads = tf.gradients(policy_network.kl_divergence, trainable_variables)
|
||||
grads_placeholder_and_vars = list(zip(grads_placeholder, trainable_variables))
|
||||
flat_grads_and_vars_placeholder_and_vars = list(zip(flat_grads_and_vars_placeholder, trainable_variables))
|
||||
|
||||
compute_grads = tf.train.AdamOptimizer(learning_rate=1e-3).apply_gradients(grads_placeholder_and_vars)
|
||||
compute_flat_grad = flatten_gradients(grads)
|
||||
apply_flat_grad = unflatten_gradients(flat_grads_and_vars_placeholder, trainable_variables)
|
||||
|
||||
sess = tf.InteractiveSession()
|
||||
sess.run(tf.global_variables_initializer())
|
||||
|
||||
for iteration in range(max_iterations):
|
||||
episode_states, episode_actions, episode_rewards = run_episode(env, policy_network)
|
||||
|
||||
episode_advantages = compute_advantages(episode_rewards, 0)
|
||||
episode_mean = np.mean(episode_rewards)
|
||||
episode_std = np.std(episode_rewards)
|
||||
|
||||
feed_dict = {
|
||||
policy_network.states: np.array(episode_states),
|
||||
policy_network.actions: np.array(episode_actions),
|
||||
policy_network.advantages: episode_advantages
|
||||
}
|
||||
|
||||
# Update policy network
|
||||
sess.run(optimizer.minimize(policy_network.policy_loss), feed_dict=feed_dict)
|
||||
|
||||
# Update value function (critic network)
|
||||
for _ in range(cg_iterations):
|
||||
sess.run(compute_flat_grad, feed_dict=feed_dict)
|
||||
|
||||
flat_grads = sess.run(compute_flat_grad, feed_dict=feed_dict)
|
||||
feed_dict[flat_grads_placeholder] = flat_grads
|
||||
|
||||
step_direction = conjugate_gradients(sess, compute_flat_grad, feed_dict)
|
||||
step_size = np.sqrt(2 * delta / (np.dot(step_direction, hessian_vector_product(sess, state, compute_flat_grad, feed_dict)) + 1e-8))
|
||||
feed_dict[flat_grads_and_vars_placeholder] = step_size * step_direction
|
||||
|
||||
sess.run(apply_flat_grad, feed_dict=feed_dict)
|
||||
|
||||
sess.close()
|
||||
|
||||
|
||||
def conjugate_gradients(sess, compute_flat_grad_fn, feed_dict, cg_iterations=10, residual_tol=1e-10):
|
||||
x = np.zeros_like(np.array(feed_dict).flatten())
|
||||
b = sess.run(compute_flat_grad_fn, feed_dict=feed_dict)
|
||||
r = b.copy()
|
||||
p = b.copy()
|
||||
rsold = np.dot(r, r)
|
||||
|
||||
for _ in range(cg_iterations):
|
||||
Ap = sess.run(compute_flat_grad_fn, feed_dict={x: p})
|
||||
alpha = rsold / (np.dot(p, Ap) + 1e-8)
|
||||
x += alpha * p
|
||||
r -= alpha * Ap
|
||||
rsnew = np.dot(r, r)
|
||||
|
||||
if np.sqrt(rsnew) < residual_tol:
|
||||
break
|
||||
|
||||
p = r + (rsnew / rsold) * p
|
||||
rsold = rsnew
|
||||
|
||||
return x
|
||||
|
||||
|
||||
def hessian_vector_product(sess, state, compute_flat_grad_fn, feed_dict, damping=0.1):
|
||||
grads = sess.run(compute_flat_grad_fn, feed_dict=feed_dict)
|
||||
constraints = tf.placeholder(tf.float32, shape=[None])
|
||||
compute_kl_grads = tf.gradients(policy_network.kl_divergence, trainable_variables)
|
||||
gradient_products = tf.reduce_sum(compute_kl_grads * constraints)
|
||||
feed_dict.update({constraints: grads})
|
||||
return sess.run(gradient_products, feed_dict=feed_dict)
|
||||
|
||||
|
||||
def flatten_gradients(grads):
|
||||
flat_grads = []
|
||||
for grad in grads:
|
||||
flat_grads.append(tf.reshape(grad, [-1]))
|
||||
return tf.concat(flat_grads, axis=0)
|
||||
|
||||
|
||||
def unflatten_gradients(grads_placeholder, trainable_variables):
|
||||
grads = []
|
||||
start = 0
|
||||
for var in trainable_variables:
|
||||
var_shape = var.shape.as_list()
|
||||
var_size = np.prod(var_shape)
|
||||
grads.append(tf.reshape(grads_placeholder[start:start+var_size], var_shape))
|
||||
start += var_size
|
||||
return grads
|
||||
|
||||
|
||||
def main():
|
||||
env = gym.make('CartPole-v1')
|
||||
|
||||
state_dim = env.observation_space.shape[0]
|
||||
action_dim = env.action_space.n
|
||||
hidden_dim = 32
|
||||
|
||||
policy_network = PolicyNetwork(state_dim, action_dim, hidden_dim)
|
||||
|
||||
train(env, policy_network, max_iterations=100)
|
||||
|
||||
env.close()
|
||||
|
||||
|
||||
if __name__ == "__main__":
|
||||
main()
|
||||
```
|
||||
|
||||
In this script, the TRPO algorithm is used to optimize a policy network to solve the CartPole-v1 environment from the Gym library. The `PolicyNetwork` class defines the policy network, and the `train` function implements the TRPO algorithm to train the network. The `compute_advantages`, `run_episode`, `conjugate_gradients`, `hessian_vector_product`, `flatten_gradients`, and `unflatten_gradients` functions are helper functions used in the training process.
|
||||
|
||||
Note that this implementation assumes you have TensorFlow and Gym libraries installed. You may need to install additional dependencies if necessary.
|
@ -1,46 +0,0 @@
|
||||
Sure! Here's an example of a Python script that demonstrates the k-Nearest Neighbors (k-NN) algorithm using the scikit-learn library:
|
||||
|
||||
```python
|
||||
import numpy as np
|
||||
from sklearn.datasets import load_iris
|
||||
from sklearn.model_selection import train_test_split
|
||||
from sklearn.neighbors import KNeighborsClassifier
|
||||
from sklearn.metrics import accuracy_score
|
||||
|
||||
# Load the Iris dataset
|
||||
iris = load_iris()
|
||||
X = iris.data
|
||||
y = iris.target
|
||||
|
||||
# Split the dataset into training and test sets
|
||||
X_train, X_test, y_train, y_test = train_test_split(X, y, test_size=0.2, random_state=42)
|
||||
|
||||
# Create a k-NN classifier with k = 3
|
||||
k = 3
|
||||
knn = KNeighborsClassifier(n_neighbors=k)
|
||||
|
||||
# Fit the classifier to the training data
|
||||
knn.fit(X_train, y_train)
|
||||
|
||||
# Predict the classes for the test set
|
||||
y_pred = knn.predict(X_test)
|
||||
|
||||
# Calculate the accuracy of the classifier
|
||||
accuracy = accuracy_score(y_test, y_pred)
|
||||
print(f"Accuracy: {accuracy}")
|
||||
|
||||
# Example usage: Predict the class for new data
|
||||
new_data = np.array([[5.0, 3.6, 1.4, 0.2]])
|
||||
predicted_class = knn.predict(new_data)
|
||||
print(f"Predicted class: {predicted_class}")
|
||||
```
|
||||
|
||||
In this script, we first load the famous Iris dataset using the scikit-learn `load_iris` function. Then, we split the dataset into training and test sets using the `train_test_split` function, with 80% for training and 20% for testing.
|
||||
|
||||
Next, we create a k-NN classifier with `k = 3` using the `KNeighborsClassifier` class from scikit-learn. We fit the classifier to the training data using the `fit` method.
|
||||
|
||||
After that, we predict the classes for the test set using the `predict` method. We calculate the accuracy of the classifier by comparing the predicted classes with the true classes using the `accuracy_score` function.
|
||||
|
||||
Finally, we demonstrate the usage of the trained classifier by predicting the class for a new data point with the `predict` method.
|
||||
|
||||
Note that this is a basic example to showcase the concept of k-NN. In practice, you would need to perform more extensive data preprocessing, feature scaling, hyperparameter tuning, and evaluation to build a more robust and accurate model.
|
@ -1,39 +0,0 @@
|
||||
Sure! Here's a Python script that demonstrates the t-SNE algorithm using the famous Iris dataset for visualization:
|
||||
|
||||
```python
|
||||
import numpy as np
|
||||
import matplotlib.pyplot as plt
|
||||
from sklearn import datasets
|
||||
from sklearn.manifold import TSNE
|
||||
|
||||
# Load the Iris dataset
|
||||
iris = datasets.load_iris()
|
||||
X = iris.data
|
||||
y = iris.target
|
||||
|
||||
# Applying t-SNE
|
||||
tsne = TSNE(n_components=2, random_state=42)
|
||||
X_tsne = tsne.fit_transform(X)
|
||||
|
||||
# Plotting the t-SNE results
|
||||
colors = ['r', 'g', 'b']
|
||||
target_names = iris.target_names
|
||||
|
||||
plt.figure(figsize=(10, 6))
|
||||
for i, target_name in enumerate(target_names):
|
||||
plt.scatter(X_tsne[y == i, 0], X_tsne[y == i, 1], c=colors[i], label=target_name)
|
||||
|
||||
plt.xlabel('First t-SNE Component')
|
||||
plt.ylabel('Second t-SNE Component')
|
||||
plt.title('t-SNE Visualization of Iris Dataset')
|
||||
plt.legend()
|
||||
plt.show()
|
||||
```
|
||||
|
||||
In this script, we first import the necessary libraries (`numpy`, `matplotlib`, `sklearn.datasets`, and `sklearn.manifold.TSNE`) to perform the t-SNE algorithm. Then, we load the Iris dataset using the `datasets.load_iris()` from scikit-learn. The data and target variables are assigned to `X` and `y` arrays, respectively.
|
||||
|
||||
After that, we create an instance of the t-SNE algorithm with `TSNE(n_components=2, random_state=42)`. We choose 2 components (`n_components=2`) since we want to visualize the data in 2D. The `random_state` parameter is set to ensure reproducibility in the results. We then apply the t-SNE algorithm to the data using `fit_transform(X)`, which returns the transformed data as `X_tsne`.
|
||||
|
||||
Finally, we plot the t-SNE results using `matplotlib`. Each data point is plotted in a scatter plot, with different colors representing different classes ('setosa', 'versicolor', and 'virginica') in the Iris dataset.
|
||||
|
||||
To run this script, ensure that you have the necessary libraries installed (`numpy`, `matplotlib`, and `scikit-learn`). Save it as a .py file and execute it using a Python interpreter. The script will show a plot with the t-SNE visualization of the Iris dataset.
|
@ -1,39 +0,0 @@
|
||||
# Understanding k-Nearest Neighbors (k-NN)
|
||||
|
||||
k-Nearest Neighbors (k-NN) is a popular and intuitive algorithm used in machine learning for both classification and regression tasks. It is a non-parametric and lazy learning algorithm, meaning it does not make any assumptions about the underlying data distribution and it only takes action when predictions are requested.
|
||||
|
||||
## How does k-NN work?
|
||||
|
||||
The basic idea behind k-NN is to classify or predict the value of a new datapoint based on the majority vote or average of its k nearest neighbors in the feature space. The choice of k is a hyperparameter that can be optimized based on the dataset and problem at hand.
|
||||
|
||||
Here is how k-NN works for classification:
|
||||
1. Calculate the distance between the new datapoint and all other datapoints in the dataset.
|
||||
2. Select the k nearest neighbors based on the calculated distances.
|
||||
3. Assign the class label to the new datapoint based on the majority vote of its neighbors.
|
||||
|
||||
For regression, the process is similar:
|
||||
1. Calculate the distance between the new datapoint and all other datapoints in the dataset.
|
||||
2. Select the k nearest neighbors based on the calculated distances.
|
||||
3. Predict the value of the new datapoint by taking the average of the target values of its neighbors.
|
||||
|
||||
## Distance Metrics in k-NN
|
||||
|
||||
The choice of distance metric is crucial in k-NN, as it determines the similarity between datapoints. The most commonly used distance metrics are Euclidean distance and Manhattan distance. Euclidean distance calculates the straight-line distance between two points in a 2D or multi-dimensional space. Manhattan distance calculates the distance by summing the absolute differences between the coordinates of two points.
|
||||
|
||||
Other distance metrics like Minkowski distance and Hamming distance can also be used depending on the nature of the data.
|
||||
|
||||
## Strengths and Weaknesses of k-NN
|
||||
|
||||
k-NN has several strengths that make it a popular choice for various applications:
|
||||
- Simplicity: k-NN is easy to understand and implement, making it accessible to users with non-technical backgrounds.
|
||||
- No training phase: k-NN does not require an explicit training phase and can immediately make predictions once the dataset is available.
|
||||
- Versatility: k-NN can handle a wide range of data types and is not limited to linearly separable data.
|
||||
|
||||
However, k-NN also has some limitations:
|
||||
- Computationally expensive: As k-NN needs to compute distances for every datapoint in the dataset, it can be slow and memory-intensive for large datasets.
|
||||
- Sensitivity to irrelevant features: Since k-NN considers all features equally, irrelevant or noisy features can negatively impact the accuracy of predictions.
|
||||
- Optimal k-value selection: Choosing the correct value of k is crucial for the accuracy of the k-NN algorithm and requires careful tuning and validation.
|
||||
|
||||
## Conclusion
|
||||
|
||||
k-Nearest Neighbors is a straightforward and effective algorithm for both classification and regression tasks. It makes predictions based on the similarity of new datapoints with their nearest neighbors. Although it has some limitations, k-NN remains a valuable tool in the machine learning toolkit due to its simplicity, versatility, and ability to handle various data types.
|
@ -1,31 +0,0 @@
|
||||
# t-SNE: Dimentionality Reduction Technique
|
||||
|
||||

|
||||
|
||||
t-SNE, which stands for t-Distributed Stochastic Neighbor Embedding, is a machine learning technique used for dimensionality reduction and visualization of high-dimensional data. It was introduced by Laurens van der Maaten and Geoffrey Hinton in 2008.
|
||||
|
||||
## Why t-SNE?
|
||||
|
||||
Dealing with high-dimensional data can be challenging as it becomes difficult to interpret and visualize the data effectively. Traditional visualization techniques like scatter plots fail to capture the complexity of high-dimensional data, which is where t-SNE comes to the rescue.
|
||||
|
||||
t-SNE helps in reducing the dimensionality of the data while preserving the local structures and relationships among the data points. It achieves this by constructing a probability distribution over pairs of high-dimensional data points and a similar distribution over pairs of low-dimensional points. It then minimizes the divergence between these two distributions using gradient descent, resulting in a low-dimensional representation of the data that can be easily visualized.
|
||||
|
||||
## How does it work?
|
||||
|
||||
The t-SNE algorithm consists of two main steps:
|
||||
|
||||
### Step 1: Constructing Similarity Measures
|
||||
In this step, t-SNE constructs a similarity matrix that reflects the pairwise similarities between data points in the high-dimensional space. It does so using a Gaussian kernel to calculate the conditional probability of similarity between two points. The bandwidth of the kernel determines the scale at which similarities decay with increasing distance.
|
||||
|
||||
### Step 2: Dimensionality Reduction
|
||||
Once the similarity matrix is constructed, t-SNE aims to find a low-dimensional representation of the data that best preserves the relationships depicted in the similarity matrix. It constructs a similar probability distribution in the low-dimensional space and minimizes the Kullback-Leibler divergence between the high-dimensional and low-dimensional distributions. This optimization is achieved using stochastic gradient descent.
|
||||
|
||||
## Advantages and Limitations
|
||||
|
||||
t-SNE has gained popularity due to its ability to effectively visualize high-dimensional data by preserving local structures. It often reveals hidden patterns, clusters, and outliers that might not be apparent in the original data.
|
||||
|
||||
However, it's important to be aware of some limitations of t-SNE. Firstly, t-SNE is non-linear, meaning that the distances in the reduced space may not correspond to the original distances accurately. Secondly, t-SNE can be highly sensitive to the parameters chosen, such as the perplexity, learning rate, and number of iterations. The perplexity determines the balance between preserving local and global structures, and it often requires experimentation to find the optimal value.
|
||||
|
||||
## Conclusion
|
||||
|
||||
t-SNE is a powerful technique for visualizing high-dimensional data and uncovering underlying structures. It has become an essential tool in various domains, including image recognition, natural language processing, bioinformatics, and more. By leveraging t-SNE, researchers and data scientists can gain valuable insights into their data, leading to better understanding and decision-making.
|
@ -1,23 +0,0 @@
|
||||
# How AI is Revolutionizing 3D Printing
|
||||
|
||||

|
||||
|
||||
Artificial Intelligence (AI) has emerged as a transformative force in various industries, and 3D printing is no exception. This cutting-edge technology, which creates physical objects by layering materials on top of each other, is being further enhanced by AI algorithms and techniques. AI is revolutionizing 3D printing by optimizing designs, improving manufacturing processes, and enhancing the overall capabilities of this innovative technology.
|
||||
|
||||
## Enhancing Design Optimization
|
||||
Designing for 3D printing is a complex task that requires careful consideration of structural integrity, material usage, and manufacturing constraints. This is where AI shines. AI algorithms, such as generative design, can process large datasets and generate designs optimized for 3D printing.
|
||||
|
||||
Generative design combines input parameters and AI to explore a vast array of possible design solutions, ultimately providing engineers with alternatives that were previously unimaginable. It takes into account factors like stress distribution, weight reduction, and material usage, resulting in novel designs that are both efficient and cost-effective. By leveraging AI-driven design optimization, 3D printing can produce intricate and high-performance objects with unparalleled ease.
|
||||
|
||||
## Improving Manufacturing Processes
|
||||
AI is also enhancing the manufacturing aspect of 3D printing by streamlining and automating various processes. Traditional 3D printing often involves manual tweaking of parameters, trial and error, and time-consuming optimization. AI algorithms can significantly reduce these inefficiencies.
|
||||
|
||||
Machine learning algorithms can analyze past printing data and fine-tune parameters to improve the printing process. They can adapt to various materials and geometries, identify potential issues beforehand, and suggest adjustments for optimal results. AI also aids in real-time monitoring of the printing process, enabling early detection of any anomalies or errors. By learning and improving from each printing session, AI continuously optimizes and enhances the manufacturing process, saving time and reducing material wastage.
|
||||
|
||||
## Expanding Application Capabilities
|
||||
AI is broadening the horizons of 3D printing by enabling the creation of objects with complex geometries and unique functionalities. With AI algorithms, designers can create intricate lattice structures and textures that weren't possible with traditional manufacturing techniques.
|
||||
|
||||
Furthermore, AI-driven 3D printing is pushing the boundaries of material science. Machine learning algorithms can predict material behavior and facilitate the development of new materials with specific properties. This opens up possibilities for advanced applications, such as printed electronics, bio-printing, and even printing with materials like glass or ceramics. AI's assistance enables 3D printers to go beyond their previous limitations and encourages innovation across industries.
|
||||
|
||||
## Conclusion
|
||||
The combination of AI and 3D printing holds immense potential for revolutionizing manufacturing and design processes. AI enables the creation of optimized designs, more efficient manufacturing techniques, and novel applications. As this synergistic collaboration continues to evolve, it will unlock the true potential of 3D printing, driving innovation and transforming various industries in the process.
|
@ -1,23 +0,0 @@
|
||||
# How AI is Revolutionizing Agriculture
|
||||
|
||||

|
||||
|
||||
Agriculture is one of the oldest and most essential industries in the world. As technology continues to advance, artificial intelligence (AI) is making its way into the agricultural sector, revolutionizing the way we grow crops, manage livestock, and make farming decisions. With the help of AI, farmers around the world are experiencing increased efficiency, sustainability, and productivity. Let's explore how AI is transforming agriculture.
|
||||
|
||||
## Precision Farming
|
||||
AI-powered technologies are enabling farmers to practice precision agriculture with measurable benefits. By collecting and analyzing data from various sources, such as sensors, satellites, and drones, AI algorithms can provide real-time insights into crop health, soil conditions, and weather patterns. This information allows farmers to optimize irrigation, fertilizer usage, and pest control measures, resulting in higher yields, reduced costs, and minimized environmental impact.
|
||||
|
||||
## Crop Monitoring
|
||||
Monitoring crops manually can be time-consuming and labor-intensive. AI-powered image recognition systems can process vast amounts of visual data captured by drones or satellites and identify early signs of crop diseases, nutrient deficiencies, or invasive weeds. With AI, farmers can quickly detect problems and take proactive measures, preventing extensive crop damage and reducing the need for excessive pesticide or herbicide use.
|
||||
|
||||
## Livestock Monitoring
|
||||
The application of AI in agriculture goes beyond crops. AI-powered systems equipped with sensors and cameras can monitor livestock behavior, health, and productivity. By analyzing data patterns, farmers can detect anomalies or signs of distress among animals, facilitating timely interventions and improving overall animal welfare. AI can also automate various tasks such as tracking feeding schedules, milk production, or egg collection, reducing manual labor and streamlining farm management.
|
||||
|
||||
## Autonomous Farming
|
||||
Self-driving vehicles and robots are finding their place in agriculture. AI-powered autonomous machines can handle various farming tasks, such as planting seeds, harvesting crops, or spraying fertilizers. These machines can work day and night without fatigue, often with higher precision than human labor. By applying AI algorithms and machine learning, these autonomous systems can adapt to different terrains, accurately identify plants, and make precise adjustments according to the needs of each crop.
|
||||
|
||||
## Improved Decision-Making
|
||||
AI can analyze vast amounts of agricultural data, including historical records, climate patterns, market trends, and scientific literature. By processing this information, AI algorithms can provide farmers with valuable insights and recommendations for better decision-making. For example, AI can suggest the optimal time for planting or harvesting, predict market demand, or identify potential disease outbreaks in specific geographical regions. By utilizing AI, farmers can make data-driven choices, minimizing risk and maximizing profitability.
|
||||
|
||||
## Conclusion
|
||||
Artificial intelligence is swiftly transforming the agriculture industry, empowering farmers with powerful tools and solutions. From precise farming techniques to livestock management, AI is revolutionizing how we grow and produce food. With the ability to monitor, analyze, and optimize various aspects of agriculture, AI is helping farmers become more efficient, sustainable, and environmentally friendly. As technology continues to advance, we can expect AI to play an increasingly significant role in shaping the future of agriculture.
|
@ -1,41 +0,0 @@
|
||||
# How AI is Revolutionizing Astronomical Research
|
||||
|
||||

|
||||
|
||||
The vastness of the universe has always captivated human beings, urging us to explore its mysteries and expand our knowledge. Over the years, technological advancements have played a crucial role in enhancing our understanding of outer space. Today, one of the most groundbreaking technologies driving the frontier of astronomical research is Artificial Intelligence (AI).
|
||||
|
||||
## Enhancing Data Analysis
|
||||
|
||||
Astronomers are flooded with petabytes of data generated by telescopes and satellites on a regular basis. Analyzing this massive amount of data manually is not only time-consuming but also prone to human errors. AI algorithms, coupled with machine learning, have brought about a revolution in processing and analyzing this data.
|
||||
|
||||
AI systems can efficiently categorize and classify celestial objects based on their properties, such as shape, color, size, and luminosity. By training AI models on large datasets of labeled observations, astronomers can quickly identify and understand various features and patterns in the universe.
|
||||
|
||||
## Discovering New Celestial Objects
|
||||
|
||||
Exploring the cosmos is a never-ending quest, and the discovery of new celestial objects is a thrilling aspect of astronomical research. AI is now playing a pivotal role in automating the process of identifying new objects in the vast sea of data.
|
||||
|
||||
With the help of AI, astronomers can detect and classify new stars, exoplanets, quasars, and other celestial bodies more accurately and efficiently. By training deep learning models on existing astronomical data, AI algorithms can recognize subtle patterns that human eyes might overlook. This enables researchers to have a deeper understanding of our universe and its composition.
|
||||
|
||||
## Advancing Image Recognition Technology
|
||||
|
||||
Images captured by telescopes are at the heart of astronomical research. Traditionally, astronomers rely on human observations to analyze celestial images, which can be both time-consuming and prone to subjective biases. AI has revolutionized this process by enabling advanced image recognition technology.
|
||||
|
||||
By utilizing convolutional neural networks (CNN), AI algorithms excel in automated image analysis. They can identify specific features or objects in images, such as galaxies, nebulae, or even transient events like supernovae. This exceptional image recognition capability has revolutionized the exploration of outer space, assisting astronomers in identifying rare and peculiar phenomena, leading to new scientific breakthroughs.
|
||||
|
||||
## Predicting Celestial Events
|
||||
|
||||
AI has also proven to be an invaluable tool in predicting various celestial events accurately. By analyzing historical data and training machine learning algorithms, astronomers can forecast astronomical phenomena with great precision. From predicting the trajectories of asteroids and comets to the occurrence of meteor showers or solar flares, AI contributes to developing reliable models for understanding and forecasting these cosmic occurrences.
|
||||
|
||||
These predictions prove vital in safeguarding our planet from potential threats and help researchers plan observational campaigns to witness rare astronomical events.
|
||||
|
||||
## Collaboration and Data Sharing
|
||||
|
||||
The AI revolution in astronomy has also fostered increased collaboration and data sharing among scientists worldwide. By automating data analysis processes and providing comprehensive catalogs of classified celestial objects, AI algorithms facilitate the swift exchange of information.
|
||||
|
||||
This shared knowledge promotes collaborations among research institutions and enables astronomers to develop a broader and more accurate understanding of the universe.
|
||||
|
||||
## Looking Ahead
|
||||
|
||||
As AI technology continues to advance, we can expect even more significant breakthroughs in astronomical research. From the discovery of new planetary systems to unraveling the mysteries of dark matter, AI is set to push the boundaries of our knowledge about the universe.
|
||||
|
||||
As we tap deeper into the immense potential of AI, astronomical research will witness unprecedented growth, uncovering hidden celestial wonders, and unlocking the secrets of our cosmos.
|
@ -1,33 +0,0 @@
|
||||
# How AI is Revolutionizing Augmented Reality (AR)
|
||||
|
||||

|
||||
|
||||
## Introduction
|
||||
|
||||
Artificial Intelligence (AI) and Augmented Reality (AR) are two groundbreaking technologies that have the potential to transform the way we interact with the world. While they can each bring significant benefits on their own, their convergence is creating new opportunities and pushing the boundaries of what is possible. In this article, we will explore how AI is revolutionizing Augmented Reality and the impact it is having on various industries.
|
||||
|
||||
## Enhancing Object Recognition and Tracking
|
||||
|
||||
One of the key challenges in AR is accurately detecting and tracking objects in the real world. Traditional computer vision techniques have limitations in handling complex scenes and occlusion. This is where AI comes into play. AI-powered algorithms powered by deep learning and neural networks can process vast amounts of visual data to achieve superior object recognition and tracking capabilities. This enables AR applications to overlay virtual objects seamlessly onto real-world scenes, providing a more immersive and realistic experience for users.
|
||||
|
||||
## Real-Time Scene Understanding and Interaction
|
||||
|
||||
AI algorithms can also empower AR systems with real-time scene understanding and interaction capabilities. By analyzing and interpreting the environment, AI can enable AR applications to understand depth, distance, and spatial relationships between objects. This allows for more advanced and interactive AR experiences, such as realistic physics simulations, accurate occlusion handling, and dynamic object behavior in response to user actions. AI can also enable AR systems to detect gestures, facial expressions, and emotions, enhancing user engagement and interaction with augmented content.
|
||||
|
||||
## Personalization and Contextual Awareness
|
||||
|
||||
Another area where AI greatly impacts AR is personalization and contextual awareness. AI algorithms can learn from user behavior, preferences, and external data sources to provide personalized and contextually relevant augmented content. With the help of Machine Learning (ML) techniques, AR apps can adapt and tailor their content recommendations based on user demographics, interests, location, and even historical data. This level of personalization enhances user satisfaction and engagement, and ultimately leads to improved AR experiences.
|
||||
|
||||
## Advancements in Simulated Environments
|
||||
|
||||
AI is also revolutionizing AR by improving simulated environments. In AR, virtual objects need to interact seamlessly with the real world, which requires accurate physics simulations and realistic rendering. AI-powered algorithms can generate sophisticated models and simulations that mimic real-world behaviors and physics. This enables more realistic and immersive AR experiences, such as virtual try-on for clothes or furniture, virtual training simulations, and architectural visualizations. AI can also optimize rendering and graphics capabilities, ensuring smooth and visually compelling AR scenes.
|
||||
|
||||
## Impact Across Industries
|
||||
|
||||
The fusion of AI and AR has wide-ranging implications across various industries. In healthcare, AI-powered AR can assist surgeons in navigation and provide real-time guidance during complex procedures. In retail, AI can enable virtual try-on solutions, personalized shopping experiences, and targeted advertising. In education, AI-enhanced AR can provide interactive and immersive learning experiences. In manufacturing, AI and AR can enhance assembly line processes, quality control, and worker training. The scope of AI and AR integration is vast and continues to expand, presenting endless possibilities for innovation.
|
||||
|
||||
## Conclusion
|
||||
|
||||
The convergence of AI and AR technologies is transforming the augmented reality landscape. With AI's capability to enhance object recognition, real-time scene understanding, and personalization, AR experiences are becoming more immersive, interactive, and contextually relevant. AI is also driving advancements in simulated environments, enabling realistic physics simulations and visually compelling AR scenes. As AI continues to advance, we can expect AR applications to become even more sophisticated, unlocking a multitude of possibilities across industries and revolutionizing the way we perceive and interact with the world around us.
|
||||
|
||||
> Note: *This article is for informational purposes only and does not constitute professional advice. The information contained herein does not reflect the views of OpenAI.*
|
@ -1,32 +0,0 @@
|
||||
# How AI is Helping and Revolutionizing: Autonomous Drones
|
||||
|
||||

|
||||
|
||||
Artificial Intelligence (AI) has risen to prominence in recent years, transforming numerous industries. One field where AI is making a significant impact is in the development and use of autonomous drones. Combining AI with drones has the potential to revolutionize various sectors, from delivery services to search and rescue missions.
|
||||
|
||||
## Enhanced Sensing and Perception
|
||||
One of the key areas where AI is helping autonomous drones is in their sensing and perception capabilities. Traditional drones rely on human operators to control their flight and navigate safely. However, with AI-enabled drones, these abilities are greatly enhanced.
|
||||
|
||||
AI algorithms can analyze data from various sensors onboard a drone, such as cameras, lidar, and infrared sensors, to continuously assess the surroundings. This enhanced perception allows autonomous drones to identify and avoid obstacles, monitor changes in the environment, and adjust their flight in real-time. With AI, drones can react quickly and safely to unexpected situations, making them more reliable and efficient in various applications.
|
||||
|
||||
## Intelligent Navigation and Mapping
|
||||
AI plays a crucial role in enabling autonomous drones to navigate complex environments and build detailed maps. Through machine learning algorithms, drones can learn from their previous flights and the experiences of other drones, improving their autonomous navigation capabilities over time.
|
||||
|
||||
By using AI-powered mapping techniques, drones can create accurate and high-resolution maps of areas they survey or visit. This capability is particularly useful for industries such as agriculture, construction, and infrastructure inspection, where detailed maps provide valuable insights. Additionally, AI can enable autonomous drones to plan efficient flight paths, optimize routes, and avoid restricted areas, making them more efficient and versatile.
|
||||
|
||||
## Object Detection and Recognition
|
||||
Another area where AI revolutionizes autonomous drones is in object detection and recognition. Advanced AI algorithms can analyze the visual data received from drone cameras to identify and classify objects of interest in real-time.
|
||||
|
||||
This capability opens up a range of possibilities for industries like logistics and delivery services. Autonomous drones can use AI to detect and track specific objects or landmarks, such as package delivery points or emergency signs. By leveraging AI, drones can accurately locate and navigate towards their intended targets, ensuring precise and efficient deliveries.
|
||||
|
||||
## Smart Decision Making
|
||||
AI-enabled drones are not only capable of perceiving their surroundings or navigating autonomously but also making intelligent decisions. By using machine learning and data analysis techniques, drones can process vast amounts of information and adapt to changing conditions.
|
||||
|
||||
In emergency situations or search and rescue missions, autonomous drones equipped with AI algorithms can make split-second decisions based on the data they collect. For example, they can detect signs of distress, analyze patterns in the data to locate missing persons, or react promptly to environmental changes.
|
||||
|
||||
## Conclusion
|
||||
The integration of AI technologies with autonomous drones is revolutionizing various industries and expanding the possibilities of unmanned aerial vehicles. Enhanced sensing and perception, intelligent navigation and mapping, object detection and recognition, and smart decision-making are just some of the areas where AI brings significant advancements.
|
||||
|
||||
As AI continues to evolve, we can expect even greater capabilities from autonomous drones. With further improvements in machine learning algorithms and the processing power of onboard computers, autonomous drones have the potential to become indispensable assets in sectors such as transportation, delivery, surveillance, and disaster response.
|
||||
|
||||
As technology continues to advance, it's crucial to ensure ethical and responsible use of AI-enabled autonomous drones, considering privacy concerns, regulations, and public safety. The combination of AI and autonomous drones opens up a world of possibilities, where efficiency, accuracy, and safety are at the forefront.
|
@ -1,37 +0,0 @@
|
||||
# How AI is Revolutionizing Bioinformatics
|
||||
|
||||

|
||||
|
||||
Artificial intelligence (AI) has emerged as a powerful tool across various industries, and one area where it is making a significant impact is bioinformatics. Bioinformatics leverages computational techniques to analyze and interpret biological data, contributing to advances in healthcare, drug discovery, and personalized medicine. With the integration of AI, bioinformatics is experiencing a revolution that enhances its capabilities and opens up new possibilities.
|
||||
|
||||
## Accelerating Genomic Analysis
|
||||
|
||||
Genomics, the study of an organism's complete set of DNA, generates vast amounts of complex data. Analyzing this data to extract meaningful insights is a monumental task that can be time-consuming and resource-intensive. AI algorithms can significantly accelerate genomic analysis, allowing researchers to process and understand data more efficiently.
|
||||
|
||||
AI-powered algorithms enable faster identification of patterns and relationships within genomic data. Machine learning models can recognize recurring motifs, uncover hidden genetic variations, and identify novel gene functions. These capabilities not only speed up the analysis process but also contribute to identifying potential disease-causing genes, predicting drug response, and facilitating targeted therapies.
|
||||
|
||||
## Precision Medicine and Treatment
|
||||
|
||||
Precision medicine aims to tailor medical interventions to individual characteristics, considering genetic, environmental, and lifestyle factors. AI technologies play a crucial role in advancing this field by analyzing vast amounts of patient data and extracting relevant insights.
|
||||
|
||||
Machine learning algorithms can identify patterns in large datasets, linking genomic information with clinical data such as patient outcomes, treatment response, and disease progression. By analyzing this integrated data, AI can predict disease risk or prognosis, recommend personalized treatments, and optimize drug dosages. This approach enhances the effectiveness of medical interventions, minimizes side effects, and improves patient outcomes.
|
||||
|
||||
## Drug Discovery and Development
|
||||
|
||||
The process of drug discovery is time-consuming and costly. AI is transforming this field by accelerating the identification of potential drug candidates and reducing the number of compounds that need to undergo extensive laboratory testing. With AI algorithms, researchers can analyze extensive databases of chemical structures and predict their biological activity, assisting in identifying molecules with therapeutic potential.
|
||||
|
||||
Additionally, AI enables the prediction of drug-target interactions, helping researchers understand how molecules interact with specific biological targets. This knowledge aids in rational drug design, allowing scientists to develop more effective and targeted therapies. As a result, the integration of AI expedites drug discovery, making the process more efficient and cost-effective.
|
||||
|
||||
## Enhancing Diagnostic Capabilities
|
||||
|
||||
AI-powered tools are revolutionizing diagnostic capabilities in bioinformatics. By analyzing medical images, AI algorithms can recognize and classify patterns associated with different diseases, improving the accuracy and efficiency of diagnoses.
|
||||
|
||||
For instance, deep learning algorithms have demonstrated exceptional performance in interpreting medical images such as MRIs or CT scans. By learning from vast datasets, AI models can identify early signs of diseases, detect anomalies, and even predict patient outcomes. This enables prompt and accurate diagnoses, leading to more effective treatment strategies and improved patient care.
|
||||
|
||||
## Ethical Considerations
|
||||
|
||||
While the integration of AI in bioinformatics brings numerous benefits, it also raises ethical considerations. Data privacy, security, and the potential for algorithmic biases are important issues that need to be addressed. It is crucial to establish robust regulations, secure data-sharing frameworks, and develop transparent AI models to ensure the ethical use of AI in bioinformatics.
|
||||
|
||||
## Conclusion
|
||||
|
||||
Artificial intelligence is revolutionizing bioinformatics by accelerating genomic analysis, advancing precision medicine, expediting drug discovery, and enhancing diagnostic capabilities. These advancements result in improved patient care, more efficient medical interventions, and the development of targeted therapies. However, ethical considerations must accompany these advancements to ensure the responsible use of AI in bioinformatics. With continued research and development, AI will continue to empower bioinformatics and contribute to significant breakthroughs in the field of life sciences.
|
@ -1,23 +0,0 @@
|
||||
# AI Revolutionizing Chatbots and Virtual Assistants
|
||||
|
||||
Artificial Intelligence (AI) has revolutionized various industries, from healthcare to finance, and now it is transforming the way we interact with technology through chatbots and virtual assistants. These AI-powered tools have significantly enhanced efficiency, communication, and customer experience across different sectors. Let's explore how AI is reshaping the world of chatbots and virtual assistants.
|
||||
|
||||
## Enhanced Natural Language Processing (NLP)
|
||||
With the advent of AI, chatbots and virtual assistants have become better at understanding and processing human language. Natural Language Processing (NLP) algorithms empower these AI tools to recognize and comprehend human speech patterns, dialects, and even contextual nuances. This breakthrough has eliminated many of the limitations of traditional chat systems and has opened up new avenues for communication between humans and machines.
|
||||
|
||||
## Improved Customer Service Experience
|
||||
AI-powered chatbots and virtual assistants have become invaluable in improving customer service experiences across various industries. These AI tools can promptly handle customer queries, provide personalized assistance, and offer real-time support 24/7. With advanced algorithms, chatbots can accurately understand customer intent and provide tailored responses, leading to higher customer satisfaction and improved brand loyalty.
|
||||
|
||||
## Efficient Task Automation
|
||||
AI has enabled enhanced automation capabilities for chatbots and virtual assistants. These AI tools can now perform a wide range of tasks, including managing appointments, answering frequently asked questions, placing orders, and even performing simple transactions. By automating such routine tasks, businesses can save time, reduce costs, and improve overall operational efficiency.
|
||||
|
||||
## Personalized Recommendations and Assistance
|
||||
Through AI algorithms, chatbots and virtual assistants can analyze vast amounts of user data and generate personalized recommendations. Whether it's suggesting relevant products based on user preferences, recommending movies or music based on past choices, or providing customized travel itineraries, AI-powered assistants can deliver a highly personalized user experience. This level of personalization helps businesses in effectively targeting their customers and building stronger relationships.
|
||||
|
||||
## Multilingual Support and Global Reach
|
||||
Language barriers pose a significant challenge in global operations. However, AI-powered chatbots and virtual assistants can easily overcome this obstacle. With powerful machine translation algorithms, these AI tools can seamlessly communicate in multiple languages, breaking down language barriers and enabling businesses to cater to diverse customer bases around the world. This expands market reach, promotes inclusivity, and fosters global growth.
|
||||
|
||||
## Continuous Learning and Improvement
|
||||
AI empowers chatbots and virtual assistants to continuously learn and improve over time. Using machine learning techniques, these AI tools can analyze user interactions, identify patterns, and adapt to better serve their users. By constantly gaining knowledge and improving their understanding of user behavior, chatbots and virtual assistants can deliver more accurate and relevant responses, creating a seamless user experience.
|
||||
|
||||
In conclusion, AI has brought remarkable advancements to the realm of chatbots and virtual assistants. From improved natural language processing and task automation to enhanced customer service experiences and personalized recommendations, AI has revolutionized the capabilities of these tools. As AI technology continues to advance, chatbots and virtual assistants will become even more sophisticated, enabling organizations across industries to provide exceptional user experiences while optimizing their operations.
|
@ -1,31 +0,0 @@
|
||||
# How AI is Revolutionizing Content Creation
|
||||
|
||||
*Artificial Intelligence (AI) has made its way into various industries, transforming the way we live and work. One such field that AI is significantly impacting is content creation. With its ability to process vast amounts of data, learn from patterns, and mimic human creativity, AI is revolutionizing the way content is produced, curated, and consumed.*
|
||||
|
||||
## Automated Content Generation
|
||||
|
||||
Gone are the days when content creation was solely reliant on human writers and creators. AI-powered tools and algorithms are now capable of generating original content autonomously. These tools leverage Natural Language Processing (NLP) and Machine Learning (ML) techniques to understand context, style, and tone, enabling the production of well-written articles, reports, and even creative pieces like poems and stories.
|
||||
|
||||
Automated content generation not only saves time and resources but also opens doors for businesses and individuals to produce large volumes of content at scale. Organizations can now create relevant, personalized content for their target audiences faster than ever before.
|
||||
|
||||
## Enhanced Content Curation
|
||||
|
||||
AI algorithms excel at gathering and analyzing vast amounts of data from various sources. This capability has revolutionized content curation, enabling personalized recommendations and streamlining the search process for users.
|
||||
|
||||
Platforms like social media, news aggregators, and video streaming services utilize AI algorithms to curate content tailored to each user's preferences, behavior, and interests. By analyzing user interactions, AI-powered recommendation systems can suggest relevant articles, videos, or products, enhancing user satisfaction and engagement.
|
||||
|
||||
## Intelligent Editing and Proofreading
|
||||
|
||||
AI-powered editing tools have become the writer's best friend. These tools assist in detecting grammar and spelling errors, suggest improvements in sentence structure, and even provide feedback on the overall readability and tone of the content. As the algorithms learn from user inputs and corrections, they continually improve, evolving into more sophisticated and helpful writing partners over time.
|
||||
|
||||
These intelligent editing tools not only save time but also enhance the quality and consistency of content. Writers can now focus on the creative aspects of their work, leaving the technical aspects to be perfected by AI.
|
||||
|
||||
## Personalized Content Recommendations
|
||||
|
||||
AI algorithms can analyze user behavior, preferences, and historical data to deliver highly personalized content recommendations. This level of personalization allows content creators and marketers to engage with their target audience more effectively.
|
||||
|
||||
Through AI-powered analytics, content creators can gain insights into what types of content perform well, which topics resonate with their audience, and even predict future preferences. By understanding user preferences and behavior, content creators can tailor their work to fulfill the individual needs of their audience, leading to increased user satisfaction and engagement.
|
||||
|
||||
## Conclusion
|
||||
|
||||
AI is undeniably revolutionizing content creation. With its automated content generation, enhanced curation, intelligent editing, and personalized recommendations, AI is changing the way content is produced, consumed, and experienced. While AI cannot replace human creativity and ingenuity, it complements and empowers content creators to become more efficient, effective, and insightful in their work. As AI continues to evolve, its impact on content creation will only grow, promising a future filled with possibilities.
|
@ -1,29 +0,0 @@
|
||||
# How AI is Helping and Revolutionizing Credit Scoring
|
||||
|
||||

|
||||
|
||||
Artificial Intelligence (AI) has transformed various industries, and one area where its impact is particularly notable is credit scoring. Traditionally, credit scoring has been a manual and time-consuming process, relying on subjective judgment and limited data. However, with the advent of AI, credit scoring has become more efficient, accurate, and fair. This article explores how AI is helping and revolutionizing credit scoring.
|
||||
|
||||
## Speed and Efficiency
|
||||
|
||||
AI has significantly accelerated the credit scoring process. It can quickly analyze vast amounts of data, including credit reports, bank statements, social media profiles, and transaction history. This allows lenders to make faster and more informed decisions. What used to take several days or weeks can now be accomplished in a matter of minutes. As a result, individuals seeking credit can receive faster approvals, enabling them to make timely financial decisions.
|
||||
|
||||
## Accuracy and Risk Assessment
|
||||
|
||||
Traditional credit scoring models were limited in their ability to accurately assess risk. They focused primarily on past credit history, disregarding other crucial factors. AI algorithms, on the other hand, can analyze numerous data points to evaluate creditworthiness more comprehensively. By considering factors like income, employment history, spending habits, and even social media behavior, AI-enabled credit scoring models provide a more accurate risk assessment. This helps lenders make more informed decisions, reducing the chances of defaults and improving overall loan performance.
|
||||
|
||||
## Non-Traditional Data
|
||||
|
||||
AI has made it possible to incorporate non-traditional data sources into credit scoring models. For instance, someone with a limited credit history or no credit history at all can be evaluated using alternative data. By analyzing factors like rental payments, utility bills, or educational background, AI algorithms can assess creditworthiness more effectively. This enables lenders to serve a wider range of individuals who may have been excluded from access to credit due to traditional scoring methods.
|
||||
|
||||
## Fairness and Bias Reduction
|
||||
|
||||
One of the critical issues with traditional credit scoring is the potential for bias. Biases based on race, gender, or income can unfairly impact individuals' creditworthiness. AI algorithms, when designed and trained properly, can reduce these biases. By relying on objective data and algorithms, AI-based credit scoring models can help ensure fair lending practices. Moreover, AI systems can be continuously monitored to identify and rectify any potential biases, leading to fairer credit decisions and increased financial inclusion.
|
||||
|
||||
## Fraud Detection
|
||||
|
||||
AI is also revolutionizing fraud detection in credit scoring. By continuously analyzing patterns and anomalies in transaction data, AI algorithms can detect fraudulent activities in real-time. Whether it's identifying stolen credit card usage or detecting suspicious account behavior, AI-powered systems can quickly flag potential fraud, protecting both lenders and borrowers.
|
||||
|
||||
## Conclusion
|
||||
|
||||
In conclusion, AI is revolutionizing the credit scoring industry by improving speed, accuracy, fairness, and fraud detection. It enables lenders to evaluate creditworthiness more efficiently, considering non-traditional data sources and reducing biases. With AI, credit decisions are made faster and more accurately, benefiting both borrowers and lenders. As AI technology continues to advance, credit scoring will continue to evolve, leading to a more inclusive and reliable financial system.
|
@ -1,33 +0,0 @@
|
||||
# How AI is Revolutionizing Customer Segmentation
|
||||
|
||||
Image Source: [Unsplash](https://unsplash.com/photos/U2BIwH_wHTc)
|
||||
|
||||
Customer segmentation, the process of dividing customers into distinct groups based on similar characteristics, is a vital strategy for businesses to optimize marketing efforts and improve customer experiences. Traditionally, this process has relied heavily on market research and data analysis techniques. However, with the rise of Artificial Intelligence (AI) technologies, customer segmentation has undergone a significant transformation, revolutionizing the way businesses understand and target their audience.
|
||||
|
||||
## Enhanced Accuracy and Efficiency
|
||||
|
||||
AI algorithms can process vast and complex datasets in a fraction of the time it would take humans to complete. This speed and precision enable businesses to identify customer segments more accurately and efficiently. By examining multiple parameters simultaneously, AI can uncover hidden patterns and correlations that would easily be missed by human analysts. As a result, the segmentation criteria become more nuanced, leading to a better understanding of customers and their behavior.
|
||||
|
||||
## Personalized Marketing Campaigns
|
||||
|
||||
One of the most notable aspects of AI-powered customer segmentation is its ability to deliver hyper-personalized marketing campaigns. By segmenting customers based on a wide range of attributes, such as demographics, purchase history, browsing behavior, and social media interactions, AI algorithms can identify individual preferences and deliver tailored content to each customer.
|
||||
|
||||
With personalized campaigns, businesses can create a more intimate connection with their customers, enhance customer satisfaction, and drive higher engagement and conversion rates. By leveraging AI-enabled customer segmentation, companies can deliver the right message at the right time, increasing the likelihood of customer loyalty and brand advocacy.
|
||||
|
||||
## Predictive Analytics and Forecasting
|
||||
|
||||
AI-driven customer segmentation also allows businesses to employ predictive analytics and forecasting techniques to gain insights into future customer behavior trends. By analyzing past customer interactions and historical data, AI algorithms can anticipate future behavior patterns and make accurate predictions about customers' likelihood to purchase, churn, or engage with particular campaigns.
|
||||
|
||||
This forward-looking approach enables companies to proactively strategize and allocate resources more effectively, reducing marketing costs and increasing return on investment (ROI). By leveraging predictive analytics, businesses can adapt their marketing strategies in real-time, ensuring they remain relevant and competitive in the ever-evolving market landscape.
|
||||
|
||||
## Real-time Customer Insights
|
||||
|
||||
AI-powered customer segmentation provides businesses with the agility to adapt their marketing strategies based on real-time customer insights. By continuously monitoring and analyzing customer behavior, AI algorithms can detect changes in preferences, needs, and interests, allowing businesses to adjust their marketing efforts accordingly.
|
||||
|
||||
For example, if a segment of customers starts displaying a particular interest in a specific product, AI-powered segmentation can help businesses identify this trend immediately and customize marketing campaigns to target that segment specifically. Real-time insights allow businesses to respond promptly to market shifts, deliver relevant content, and maintain a competitive edge.
|
||||
|
||||
## Conclusion
|
||||
|
||||
The advent of AI has revolutionized customer segmentation, empowering businesses to better understand their customers, optimize marketing efforts, and improve overall customer experiences. By leveraging AI algorithms' speed, accuracy, and predictive capabilities, companies can personalize marketing campaigns, predict future behavior, and adapt strategies in real-time.
|
||||
|
||||
As AI technology continues to advance, businesses that harness the power of AI-powered customer segmentation will enjoy a significant competitive advantage. By staying ahead of market trends and meeting customers' evolving demands, companies can create lasting customer relationships and drive business growth in the era of intelligent automation.
|
@ -1,29 +0,0 @@
|
||||
# How AI is Revolutionizing Cybersecurity
|
||||
|
||||

|
||||
|
||||
Artificial Intelligence (AI) has emerged as a game-changer in almost every industry, and cybersecurity is no exception. With the increasing sophistication of cyber threats, organizations are turning to AI-powered technologies to strengthen their defenses. The ability of AI to analyze large amounts of data, detect anomalies, and automate responses has revolutionized the field of cybersecurity, delivering improved protection, threat intelligence, and incident response capabilities. Here’s how AI is transforming cybersecurity landscape:
|
||||
|
||||
## Advanced Threat Detection
|
||||
|
||||
Traditional security tools often struggle to detect sophisticated and rapidly evolving cyber threats. AI brings a new level of sophistication to threat detection by leveraging machine learning algorithms that can identify patterns, anomalies, and outliers in real-time. By analyzing massive datasets and learning from historical cyber attacks, AI systems can identify even the most elusive threats. They can also adapt and evolve as new threats emerge, making them invaluable in the battle against cybercrime.
|
||||
|
||||
## Behavior-Based Authentication
|
||||
|
||||
Authentication is a crucial aspect of cybersecurity, and AI has made significant advancements in this area as well. Instead of relying solely on static credentials like usernames and passwords, AI systems can analyze user behavior and establish patterns to determine if an account has been compromised. By continuously learning from user interactions, AI can detect abnormal activities and flag potential security risks, providing an additional layer of protection against unauthorized access.
|
||||
|
||||
## Automated Incident Response
|
||||
|
||||
The ability to respond swiftly to cyber threats is vital for minimizing the potential damage. AI enables real-time threat detection and automated incident response, allowing organizations to save valuable time and resources. AI-powered response systems can identify, analyze, and contain threats faster than human operators, limiting the impact of a potential security breach. By automating routine security tasks, IT teams can focus on more strategic initiatives and mitigate risks before they escalate.
|
||||
|
||||
## Enhanced Malware Detection
|
||||
|
||||
Malware is one of the most common and pervasive cybersecurity threats. AI has dramatically improved malware detection capabilities by analyzing code, behavior, and network traffic associated with malicious software. Machine learning algorithms can identify previously unseen malware and zero-day exploits, leading to more effective defense strategies. AI systems can also mitigate false positives and false negatives, reducing the risks of both missed threats and unnecessary alarms.
|
||||
|
||||
## Predictive Analytics and Threat Intelligence
|
||||
|
||||
AI can predict future attack patterns based on historical data and trends. By analyzing vast amounts of cybersecurity information, AI systems can identify potential vulnerabilities and generate accurate threat intelligence. This proactive approach helps organizations stay one step ahead of cybercriminals, allowing them to implement preventive measures before an attack occurs. From identifying vulnerabilities in computer networks to predicting phishing campaigns and social engineering tactics, AI empowers organizations to anticipate and counter potential cyber threats effectively.
|
||||
|
||||
## Conclusion
|
||||
|
||||
The ever-evolving nature of cyber threats demands advanced and proactive security measures, and AI emerges as a transformative force in cybersecurity. By leveraging machine learning, behavior analysis, and predictive analytics, organizations can significantly enhance their defense capabilities. AI-powered cybersecurity systems excel in detecting and mitigating sophisticated threats, automating incident response, and uncovering vulnerabilities. With AI's continuous evolution and adaptation, the fight against cybercrime becomes more robust, enabling organizations to safeguard their valuable assets in an increasingly digital world.
|
@ -1,29 +0,0 @@
|
||||
# AI Revolutionizing Drug Discovery
|
||||
|
||||
Artificial Intelligence (AI) has emerged as a groundbreaking technology in various domains, and one area where it has shown immense potential is drug discovery. The process of developing new drugs is lengthy, expensive, and often hit-or-miss. However, AI is now changing the game by enhancing efficiency, reducing costs, and accelerating the discovery of life-saving pharmaceuticals.
|
||||
|
||||
## Shortening the Timeline
|
||||
|
||||
Traditional drug discovery involves exhaustive experiments and testing, which can take years to complete. Through AI, this timeline can be significantly shortened. Machine learning algorithms can analyze vast amounts of data, including genetic and biological information, medical literature, and even existing drug databases. AI models can spot patterns, identify potential drug targets, and predict the efficacy of candidate compounds, streamlining the research process.
|
||||
|
||||
## Enhanced Efficiency in Screening
|
||||
|
||||
AI algorithms have the ability to sift through millions of chemical compounds to identify potential drug candidates. Instead of relying on time-consuming laboratory experiments, AI models can simulate interactions between molecules, predicting their behavior and binding affinity. This accelerates the screening process, highlighting the most promising compounds for further investigation.
|
||||
|
||||
## Repurposing Existing Drugs
|
||||
|
||||
AI can also contribute to drug discovery by reevaluating existing drugs for new applications. By analyzing the properties of known compounds and comparing them with different diseases or conditions, AI can identify potential drug candidates for repurposing. This approach is more cost-effective and time-efficient compared to developing entirely new drugs, potentially leading to the discovery of treatments for previously unaddressed medical conditions.
|
||||
|
||||
## Reducing Costs and Failures
|
||||
|
||||
Developing a new drug is an expensive endeavor, with a high rate of failure. AI helps in minimizing both costs and the number of failed experiments. By predicting the properties and behavior of molecules, AI can provide researchers with valuable insights into the drug development process, helping them prioritize the most promising avenues and avoid potentially dead-end experiments. Moreover, AI-driven simulations and predictive models allow researchers to understand the potential side effects and toxicity of a compound before it enters costly clinical trials.
|
||||
|
||||
## Accelerating Personalized Medicine
|
||||
|
||||
AI is also playing a crucial role in enabling personalized medicine. By analyzing large-scale patient data, AI algorithms can identify molecular signatures associated with different diseases, allowing for targeted and personalized treatments. This approach holds immense potential for tailoring drugs based on an individual's genetic makeup, leading to more effective and safer therapies.
|
||||
|
||||
## Ethical Considerations
|
||||
|
||||
While AI has shown tremendous promise in revolutionizing drug discovery, there are ethical considerations that must be addressed. The transparency and interpretability of AI models, data privacy, and ensuring unbiased algorithms are crucial in maintaining public trust and avoiding potential pitfalls.
|
||||
|
||||
In conclusion, AI is transforming the field of drug discovery. By leveraging the power of machine learning and advanced algorithms, researchers can save time, reduce costs, and increase the success rate of discovering life-saving drugs. With continued research and development, AI-driven drug discovery holds the promise of revolutionizing the healthcare industry and improving patient outcomes worldwide.
|
@ -1,31 +0,0 @@
|
||||
# How AI is Revolutionizing E-commerce Visual Recognition
|
||||
|
||||
Technology has always been a driving force behind the evolution of industries, and the field of e-commerce is no exception. In recent years, Artificial Intelligence (AI) has emerged as a powerful tool that is reshaping the way businesses operate. One area in particular where AI is making a significant impact is e-commerce visual recognition. With the ability to analyze images and videos at an unprecedented speed and accuracy, AI is transforming the way consumers and businesses interact in the online shopping world.
|
||||
|
||||
## Enhanced Search and Personalization
|
||||
|
||||
AI-powered visual recognition technology is revolutionizing the way we search for products online. Traditional search engines have long relied on text-based queries, but AI is taking product search to a whole new level. Visual search capabilities allow users to find items by simply uploading an image, rather than using keywords. This is particularly useful when customers are unable to accurately describe the product they desire, or when they are unsure of how to phrase their search query.
|
||||
|
||||
Additionally, AI enables personalized recommendations based on visual preferences. By analyzing a user's browsing history and visual engagement with various products, AI algorithms can create a tailored shopping experience. This not only enhances customer satisfaction but also increases conversion rates for e-commerce businesses.
|
||||
|
||||
## Streamlined Product Categorization and Tagging
|
||||
|
||||
In the world of online shopping, it is crucial for businesses to accurately categorize and tag their products. AI-powered visual recognition simplifies this process by automatically assigning categories and tags based on the visual content of the product images. This eliminates the need for manual sorting and tagging, saving businesses a significant amount of time and resources.
|
||||
|
||||
Moreover, AI can accurately identify and group visually similar items, even across different brands and product lines. This enables e-commerce platforms to offer customers a seamless shopping experience with accurate product recommendations and related items.
|
||||
|
||||
## Improved Inventory Management and Demand Forecasting
|
||||
|
||||
Managing inventory and accurately forecasting demand are key challenges faced by e-commerce businesses. AI-powered visual recognition technology helps overcome these obstacles by providing real-time insights into inventory levels and demand patterns.
|
||||
|
||||
By analyzing visual cues, such as product popularity, customer engagement, and social media trends, AI algorithms can predict future demand with a high degree of accuracy. This empowers businesses to optimize their inventory management, reduce costs associated with overstocking or stockouts, and ultimately increase profitability.
|
||||
|
||||
## Preventing Counterfeit Products and Fraud
|
||||
|
||||
Counterfeit products pose a significant threat to e-commerce businesses and consumer trust. However, AI is playing a crucial role in combating this issue. E-commerce platforms are leveraging visual recognition technology to detect counterfeit products by analyzing product images, logos, and packaging. By comparing images against known authentic products, AI algorithms can identify potential fakes and help prevent them from reaching the market.
|
||||
|
||||
Furthermore, AI-powered fraud detection algorithms can analyze visual patterns and behaviors to identify suspicious activities, such as fake reviews, click fraud, or account takeovers. This enhances security for both businesses and customers, fostering a safer online shopping environment.
|
||||
|
||||
## Conclusion
|
||||
|
||||
AI-powered visual recognition technology is transforming the world of e-commerce. By enhancing search capabilities, streamlining product categorization, improving inventory management, and combating counterfeits and fraud, AI is revolutionizing how businesses and consumers interact in the online shopping landscape. As technology continues to advance, we can expect even more innovative applications of AI in e-commerce visual recognition, further enhancing the customer experience and driving business growth.
|
@ -1,22 +0,0 @@
|
||||
# How AI is Revolutionizing E-learning Platforms
|
||||
|
||||
## Introduction
|
||||
Artificial Intelligence (AI) has made significant advancements in various fields, and one area where its impact is particularly noteworthy is in e-learning platforms. With the ability to analyze vast amounts of data, understand patterns, and adapt to individual needs, AI is transforming the way we learn. In this article, we will explore how AI is helping and revolutionizing e-learning platforms.
|
||||
|
||||
## Personalized Learning
|
||||
One of the biggest challenges in traditional education is providing personalized learning experiences to each student. AI-powered e-learning platforms have the capability to address this issue by creating personalized learning paths for individuals. By analyzing students' preferences, performance, and learning styles, AI algorithms can recommend tailored content, exercises, and assessments. This personalized approach allows students to learn at their own pace and focus on areas where they need improvement, resulting in better learning outcomes.
|
||||
|
||||
## Intelligent Tutoring
|
||||
AI-powered tutors are becoming increasingly popular in e-learning platforms. These tutors can understand and respond to students' questions, providing real-time assistance and guidance. Using natural language processing (NLP) and machine learning algorithms, AI tutors can offer explanations, clarify doubts, and even engage in interactive dialogues with learners. This personalized support helps students navigate complex topics, fosters deeper understanding, and encourages active engagement in the learning process.
|
||||
|
||||
## Automated Grading and Feedback
|
||||
Grading and providing feedback on assignments can be time-consuming for educators. AI algorithms can automate this process, saving valuable time and effort. Machine learning models can be trained to assess and grade assignments, essays, and quizzes with a high degree of accuracy. Moreover, these models can provide detailed feedback to students, highlighting areas of improvement and suggesting further resources. This not only speeds up the grading process but also ensures consistency and fairness in assessments.
|
||||
|
||||
## Content Recommendation
|
||||
AI-powered recommendation systems have become commonplace in many online platforms, including e-learning. These systems analyze learners' behavior, preferences, and performance to suggest relevant and personalized content. By taking into account factors such as the difficulty level, learning style, and prior knowledge of the learner, AI algorithms can recommend courses, modules, and resources that are most suitable for individual needs. This helps learners discover new topics, explore diverse perspectives, and make the most of their e-learning experience.
|
||||
|
||||
## Intelligent Data Analytics
|
||||
AI enables e-learning platforms to collect and analyze vast amounts of data in real-time. This data includes learners' interactions, progress, and performance metrics. By leveraging AI algorithms, educators and administrators can gain valuable insights into learners' behavior, identify areas of improvement, and make data-driven decisions. For example, AI analytics can detect patterns indicating learner disengagement, allowing educators to intervene and provide timely support. Additionally, AI analytics can help in evaluating the effectiveness of different instructional strategies, enabling continuous improvement in e-learning platforms.
|
||||
|
||||
## Conclusion
|
||||
AI has brought significant advancements to e-learning platforms and is revolutionizing the way we learn. Personalized learning, intelligent tutoring, automated grading, content recommendation, and intelligent data analytics are just a few examples of how AI is enhancing e-learning experiences. As AI continues to evolve, we can expect further innovation in e-learning platforms, making education more accessible, engaging, and effective for learners worldwide.
|
@ -1,25 +0,0 @@
|
||||
# How AI is Revolutionizing Elderly Care Robotics
|
||||
|
||||

|
||||
|
||||
The field of robotics has made significant advancements in recent years, especially in the domain of elderly care. With the help of Artificial Intelligence (AI), robots are now being developed and deployed to assist and provide care for the elderly in ways that were once unimaginable. This technological revolution is transforming the lives of senior citizens, enabling them to live more comfortably and independently while reducing the burden on caregivers. Let's explore how AI is revolutionizing elderly care robotics.
|
||||
|
||||
## Enhanced Assistance for Activities of Daily Living (ADLs)
|
||||
|
||||
One of the primary areas in which AI is making a difference in elderly care robotics is by providing enhanced assistance with Activities of Daily Living (ADLs). AI-powered robots are capable of performing tasks such as feeding, bathing, and dressing elderly individuals, thus enabling them to maintain their dignity and independence. These robots can understand human speech, interpret gestures, and even anticipate their users' needs through machine learning algorithms. By reducing the reliance on human caregivers for basic tasks, AI-powered robots are allowing seniors to lead more autonomous lives.
|
||||
|
||||
## Continuous Monitoring and Improved Safety
|
||||
|
||||
AI is also being harnessed to improve the safety and well-being of the elderly. With advanced sensors and intelligent algorithms, robots can now continuously monitor the vital signs of seniors, including heart rate, blood pressure, and sleep patterns. AI algorithms can detect anomalies and alert healthcare professionals or caregivers in case of emergencies. Additionally, robots with fall detection capabilities can quickly respond when an elderly person falls and issue an alert if necessary. The use of AI-powered robots for continuous monitoring minimizes the risks associated with isolated older adults and provides peace of mind to both the elderly and their loved ones.
|
||||
|
||||
## Social Interaction and Companionship
|
||||
|
||||
Loneliness and social isolation are major challenges faced by many elderly individuals. AI-powered robots are helping to address this issue by providing social interaction and companionship. These robots are equipped with natural language processing capabilities, enabling them to hold conversations and engage with the elderly in a meaningful way. They can play music, read books, share news, and provide personalized entertainment. Through machine learning algorithms, these robots learn from their interactions with seniors, adapting their behavior to offer companionship that better suits the individual's preferences and needs.
|
||||
|
||||
## Cognitive Stimulation and Memory Support
|
||||
|
||||
AI-powered robots are also contributing to cognitive stimulation and memory support for the elderly. These robots can engage in cognitive exercises, memory games, and brain training activities to keep the mind active. They can also provide medication reminders, help manage schedules, and even assist with tasks like grocery shopping. By leveraging AI, these robots tailor their approaches based on individual capabilities and sensory perception. They offer personalized support to enhance cognitive function and memory retention, thereby improving the overall mental well-being of seniors.
|
||||
|
||||
## Conclusion
|
||||
|
||||
The integration of AI in elderly care robotics is transforming the way we support and care for our aging population. These robots, equipped with AI algorithms, are revolutionizing elderly care by assisting with ADLs, continuously monitoring vital signs, providing social interaction and companionship, as well as offering cognitive stimulation and memory support. By leveraging AI technology, these robots aim to enhance the quality of life for the elderly, promote independence, and alleviate the burden on caregivers. As the field of robotics and AI further advances, we can expect to witness even more profound changes in elderly care, making the lives of senior citizens healthier, happier, and more comfortable.
|
@ -1,35 +0,0 @@
|
||||
# How AI is Revolutionizing Energy Consumption Optimization
|
||||
|
||||

|
||||
|
||||
Artificial intelligence (AI) has the power to revolutionize numerous industries, and one such area experiencing a significant transformation is energy consumption optimization. As we endeavor to tackle climate change and build a sustainable future, AI is proving instrumental in optimizing energy usage, reducing waste, and achieving greater efficiency.
|
||||
|
||||
## Smart Grids and Demand Response
|
||||
|
||||
One of the major applications of AI in energy consumption optimization is the implementation of smart grids. These intelligent grids leverage AI algorithms to balance electricity supply and demand, resulting in optimized energy usage. By analyzing historical data, real-time demand patterns, weather conditions, and consumer behavior, AI algorithms can predict electricity demand accurately.
|
||||
|
||||
Based on these predictions, AI can optimize energy distribution by rerouting power to different areas in real-time. This ability to balance the load reduces the risk of power outages during high demand periods and ensures an uninterrupted energy supply.
|
||||
|
||||
Moreover, AI-powered demand response systems enable utilities to incentivize consumers to reduce their electricity consumption during peak hours. By providing real-time feedback, personalized recommendations, and financial incentives, AI systems encourage consumers to shift their usage to non-peak hours, significantly reducing strain on the grid and minimizing energy wastage.
|
||||
|
||||
## Energy Efficiency in Buildings
|
||||
|
||||
AI is also transforming energy consumption optimization in buildings. Machine learning algorithms, paired with advanced sensors and IoT devices, enable energy management systems to continuously monitor and analyze energy consumption patterns within a building.
|
||||
|
||||
By collecting and analyzing vast amounts of data on energy usage, temperature, occupancy, and weather conditions, AI algorithms can identify inefficiencies and suggest energy-saving measures. For example, the system can automatically adjust temperature settings, turn off lights in unoccupied rooms, or optimize equipment schedules to reduce energy waste.
|
||||
|
||||
Machine learning algorithms can also learn from historical data to predict patterns and recommend long-term energy efficiency measures. By uncovering hidden insights and patterns, AI systems help building owners and managers make data-driven decisions to optimize energy consumption, reduce costs, and enhance sustainability.
|
||||
|
||||
## Renewable Energy Optimization
|
||||
|
||||
Renewable energy sources, such as solar and wind, are crucial in the fight against climate change. However, their intermittent nature presents challenges in maintaining a stable energy supply. AI plays a vital role in optimizing the integration of renewable energy into the power grid.
|
||||
|
||||
By leveraging machine learning algorithms, AI systems can forecast the availability of renewable energy sources based on weather conditions and historical data. This enables grid operators to proactively manage energy supply and demand, ensuring optimal utilization of renewable energy.
|
||||
|
||||
Furthermore, AI can optimize the placement and operation of renewable energy systems. By analyzing factors such as geographical location, climate patterns, and energy demand, AI algorithms can determine the most efficient locations for solar panels or wind turbines. This helps maximize energy generation while minimizing costs and environmental impacts.
|
||||
|
||||
## Conclusion
|
||||
|
||||
AI technology offers immense potential in revolutionizing energy consumption optimization across various sectors. From smart grids balancing energy supply and demand to AI-powered energy efficiency systems in buildings and optimization of renewable energy integration, the possibilities are vast.
|
||||
|
||||
By applying AI algorithms, businesses, utilities, and individuals can achieve higher energy efficiency, reduce costs, and minimize environmental footprint. As we continue to explore and harness the power of AI in our quest for a sustainable future, energy consumption optimization will undoubtedly play a critical role in building a greener and more efficient world.
|
@ -1,25 +0,0 @@
|
||||
# How AI is Revolutionizing Facial Recognition
|
||||
|
||||
Artificial intelligence (AI) has permeated nearly every aspect of our lives, and one area where its impact has been particularly profound is facial recognition technology. AI-powered facial recognition has revolutionized various industries, from security and law enforcement to marketing and personal devices. With unprecedented accuracy and efficiency, AI has made facial recognition an indispensable tool in today's world.
|
||||
|
||||
## Enhanced Security and Law Enforcement
|
||||
|
||||
AI-powered facial recognition has significantly strengthened security measures worldwide. It has become a crucial component in identifying and apprehending criminals, enhancing public safety, and thwarting potential threats. AI algorithms can quickly scan vast databases of images and videos to identify individuals accurately, even in real-time scenarios. This cutting-edge technology enables law enforcement agencies to track down suspects more effectively, prevent crimes, and even anticipate security breaches.
|
||||
|
||||
## Simplified Identity Verification
|
||||
|
||||
Traditionally, identity verification was a time-consuming and cumbersome process involving manual checks and document verification. However, AI-powered facial recognition has simplified this process significantly. Whether it's at airports, financial institutions, or other identity-dependent scenarios, facial recognition technology ensures seamless and efficient identity verification. By analyzing unique facial features and matching them against existing records, AI algorithms can authenticate individuals swiftly and accurately.
|
||||
|
||||
## Enhanced User Experience on Personal Devices
|
||||
|
||||
AI has transformed the way we interact with our personal devices, making facial recognition an integral part of our everyday lives. From unlocking smartphones to making secure payments, AI-powered facial recognition has made these tasks more convenient, efficient, and secure. By using deep learning algorithms, AI can map facial features to create highly detailed and accurate facial models, ensuring reliable and secure authentication. As a result, users no longer need to remember complex passwords or patterns, enhancing both convenience and security.
|
||||
|
||||
## Personalized Marketing and Retail Experiences
|
||||
|
||||
AI-powered facial recognition has opened up endless possibilities for marketers and retailers to deliver personalized experiences to their customers. By analyzing facial expressions, AI algorithms decode emotions, preferences, and reactions, allowing companies to tailor their products, promotions, and advertisements accordingly. For example, AI-powered smart billboards can display ads based on the age, gender, and even the mood of the passersby, ensuring higher engagement and impact. This level of personalization not only enhances customer satisfaction but also drives business growth.
|
||||
|
||||
## Ethical Considerations and Mitigating Biases
|
||||
|
||||
While the benefits of AI-powered facial recognition are undeniable, it also raises ethical concerns regarding privacy, surveillance, and potential biases. Misuse of facial recognition technology could violate individuals' privacy, leading to concerns about mass surveillance and infringement of personal freedoms. Additionally, biases in datasets used to train AI models can lead to inaccurate identifications or discrimination. To address these concerns, there is a growing need for transparent governance, proper regulations, and bias mitigation techniques to ensure the responsible and ethical use of facial recognition technology.
|
||||
|
||||
In conclusion, AI-powered facial recognition has revolutionized various industries, bringing unparalleled accuracy, efficiency, and convenience. Its impact ranges from enhanced security and law enforcement to personalized marketing experiences, simplifying identity verification, and transforming user experiences on personal devices. While ethical considerations need to be addressed, there is no doubt that facial recognition technology will continue to evolve and shape our future, making it an essential tool in our increasingly digital world.
|
@ -1,31 +0,0 @@
|
||||
# How AI is Revolutionizing Fraud Detection
|
||||
|
||||
Artificial Intelligence (AI) is rapidly transforming various industries, and one area where it is making a significant impact is in fraud detection. Traditional methods of identifying fraudulent activities often fall short due to the complexity and ever-evolving nature of fraud schemes. However, with the power of AI, organizations are now able to detect and mitigate fraudulent behavior more effectively than ever before.
|
||||
|
||||
## Enhancing Accuracy through Machine Learning
|
||||
|
||||
Machine Learning (ML) algorithms are the backbone of AI-powered fraud detection systems. ML algorithms can analyze vast amounts of data, detect patterns, and identify anomalies that may indicate fraudulent activities. By continuously learning from new data and adapting to emerging fraud trends, AI systems can improve their accuracy and stay one step ahead of fraudsters.
|
||||
|
||||
ML algorithms are proficient in analyzing structured and unstructured data from a wide range of sources, including transaction records, customer behavior, and user profiles. They can quickly identify suspicious patterns or indicators of fraud that may not be apparent to human analysts. This ability to process massive volumes of data in real-time significantly reduces false positives and false negatives, resulting in an enhanced fraud detection rate.
|
||||
|
||||
## Advanced Analytics and Predictive Models
|
||||
|
||||
AI-driven fraud detection systems leverage advanced analytics and predictive models to identify potential fraud cases proactively. By analyzing historical data, these models can identify patterns, trends, and anomalies that may indicate fraudulent behavior. The systems can then assign a risk score to individual transactions or user behaviors, making it easier for organizations to prioritize their investigations and allocate resources effectively.
|
||||
|
||||
Using predictive models, AI systems can also accurately forecast the likelihood of future fraud incidents. This enables businesses and financial institutions to take preventative measures, such as strengthening security protocols, adding additional authentication layers, or notifying customers about potential risks associated with specific transactions or behaviors.
|
||||
|
||||
## Real-Time Fraud Prevention
|
||||
|
||||
Traditional fraud detection methods often suffer from delays, as they rely on manually generated reports or periodic audits. In contrast, AI-powered solutions can instantly flag potentially fraudulent activities in real-time. By analyzing transactions, user behaviors, and other relevant data in real-time, AI systems can promptly detect and prevent fraud while minimizing the impact on legitimate customers.
|
||||
|
||||
AI algorithms continuously monitor and assess multiple factors simultaneously, such as transaction amounts, frequency, geographic locations, and device information. When an unusual activity is detected, the system can automatically trigger preventive measures, such as halting transactions, sending alerts to customers, or conducting further investigations. These proactive measures not only prevent financial losses but also safeguard customer trust and maintain brand reputation.
|
||||
|
||||
## Combating Sophisticated Fraud Techniques
|
||||
|
||||
Fraudsters are constantly developing new and sophisticated techniques to evade detection. However, AI-powered fraud detection systems are well-equipped to combat these evolving methods. By incorporating Natural Language Processing (NLP) and sentiment analysis, AI systems can detect fraud attempts hidden in textual data, such as emails, chat transcripts, or social media conversations. This capability enables organizations to rapidly respond to emerging threats and protect themselves against previously unknown fraud vectors.
|
||||
|
||||
Moreover, AI algorithms can learn from millions of fraud cases, uncovering intricate patterns and relationships that may be invisible to human analysis. This empowers fraud detection systems to identify emerging fraud trends and adjust their detection capabilities accordingly. By continuously adapting to new patterns and techniques, AI systems enhance their fraud detection and prevention capabilities with minimal manual intervention.
|
||||
|
||||
## Conclusion
|
||||
|
||||
AI has significantly revolutionized fraud detection by enabling organizations to proactively identify and combat fraudulent activities with accuracy and speed. Through the power of machine learning, advanced analytics, and predictive models, AI systems can process vast amounts of complex data to detect anomalies and patterns indicative of fraud. Real-time monitoring and proactive prevention measures not only reduce financial losses but also safeguard customer trust. As fraudsters become more sophisticated, AI-driven fraud detection systems continue to evolve to stay one step ahead and protect businesses and individuals from various types of fraudulent activities.
|
@ -1,33 +0,0 @@
|
||||
# How AI is Revolutionizing Handwriting Recognition
|
||||
|
||||
In recent years, the field of Artificial Intelligence (AI) has experienced significant advancements in various domains. One such domain is handwriting recognition, where AI has played a vital role in revolutionizing the way handwritten text is interpreted and utilized. With the help of AI-powered algorithms, handwriting recognition technology has become smarter, more accurate, and increasingly accessible. Let's delve into how AI is transforming this field.
|
||||
|
||||
## Enhanced Accuracy and Speed
|
||||
|
||||
Traditional handwriting recognition algorithms rely on rule-based methods, which often struggle with the variability and complexity of handwritten text. However, AI algorithms are capable of learning from vast amounts of data, enabling them to detect patterns and generate accurate predictions with unparalleled precision. These algorithms can recognize different handwriting styles, including cursive writing, block letters, and even calligraphy.
|
||||
|
||||
Moreover, AI-powered recognition systems are much faster than their traditional counterparts. They can process and analyze large volumes of handwritten data within seconds, significantly improving efficiency and productivity in tasks that involve handwritten text interpretation.
|
||||
|
||||
## Natural Language Processing
|
||||
|
||||
AI has greatly expanded the capabilities of handwriting recognition systems by incorporating natural language processing techniques. This allows the algorithms to not only recognize individual written words or characters but also to understand the context and semantics behind them. Consequently, AI-powered models can now interpret complete sentences or paragraphs and perform various tasks such as language translation, sentiment analysis, and text summarization.
|
||||
|
||||
## Intelligent Data Extraction
|
||||
|
||||
Efficiently extracting important information from handwritten forms or documents can be a time-consuming task. However, AI-enabled handwriting recognition technologies are making this process smarter and more efficient. By leveraging machine learning algorithms, these systems can automatically locate and extract key data elements such as names, addresses, dates, and numbers from handwritten text, regardless of the writing style or variations.
|
||||
|
||||
This intelligent data extraction capability is highly valuable in sectors such as finance, healthcare, and legal industries, where handwritten documents play a significant role. It reduces the manual effort required for data entry, minimizes errors, and improves overall productivity.
|
||||
|
||||
## Improved Accessibility and Digitalization
|
||||
|
||||
The integration of AI in handwriting recognition has made it more accessible and user-friendly. Gone are the days when one had to purchase expensive specialized equipment or software to digitize handwritten text. Now, anyone with a smartphone or a tablet can effortlessly convert their handwritten notes into digital format using various AI-powered apps or tools available.
|
||||
|
||||
This digitalization of handwritten text has opened up new possibilities in terms of storage, retrieval, and sharing of information. It enhances collaboration, allows for easy searchability, and eliminates the risk of losing or misplacing important handwritten documents.
|
||||
|
||||
## Future Implications and Potential
|
||||
|
||||
The advancements in AI-powered handwriting recognition have significant implications for various domains. For example, in education, AI can assist students by analyzing their handwritten work, providing feedback, and aiding in their learning process. In the healthcare industry, AI-driven recognition systems can quickly and accurately interpret doctors' handwritten prescriptions, reducing the risk of medication errors.
|
||||
|
||||
With ongoing research and development, AI algorithms are expected to become even more proficient in deciphering handwriting, regardless of its complexity or variation. This opens up endless possibilities for applications in forensics, historical document analysis, and many other fields.
|
||||
|
||||
In conclusion, AI has undoubtedly revolutionized handwriting recognition. The combination of enhanced accuracy, natural language processing, intelligent data extraction, improved accessibility, and digitalization has transformed the way we interpret and utilize handwritten text. As AI technology continues to progress, we can expect further advancements in this domain, resulting in a multitude of exciting applications and improved efficiency in numerous industries.
|
@ -1,35 +0,0 @@
|
||||
# The Role of AI in Revolutionizing Healthcare Diagnosis
|
||||
|
||||
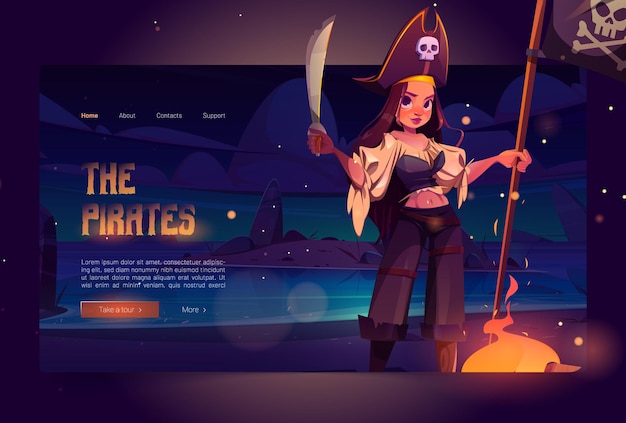
|
||||
|
||||
Artificial Intelligence (AI) has been making significant advancements in various fields, and one area where it is making a tremendous impact is healthcare diagnosis. By harnessing the power of AI, healthcare professionals are now able to improve accuracy, efficiency, and patient outcomes like never before. Let's explore how AI is revolutionizing healthcare diagnosis.
|
||||
|
||||
## Early Detection and Diagnosis
|
||||
|
||||
AI-enabled systems have the capability to analyze vast amounts of medical data and spot patterns that human experts might overlook. By applying machine learning algorithms to patient records, lab results, and medical imaging such as X-rays or MRIs, AI systems can detect early signs of diseases or conditions that could often be missed during a manual assessment.
|
||||
|
||||
This early detection and diagnosis can be a game-changer, especially for complex diseases like cancer, where early intervention significantly improves treatment success rates. AI-driven diagnostic tools empower healthcare professionals with more accurate and timely insights, saving valuable time in the diagnostic process and facilitating prompt treatment planning.
|
||||
|
||||
## Precision Medicine
|
||||
|
||||
AI's ability to process immense amounts of genetic and clinical data has opened doors to personalized treatment strategies, known as precision medicine. Through genetic profiling, AI algorithms can identify specific biomarkers associated with diseases, providing insights into the most effective medications or therapies for individual patients.
|
||||
|
||||
By tailoring treatments based on an individual's genetic makeup, precision medicine not only enhances the effectiveness of therapies but also reduces adverse side effects. The use of AI in precision medicine enables healthcare professionals to deliver more targeted, personalized care, improving patient outcomes and quality of life.
|
||||
|
||||
## Imaging and Medical Diagnosis
|
||||
|
||||
Medical imaging plays a vital role in diagnosing and monitoring various conditions. AI-powered imaging analysis algorithms offer invaluable assistance to radiologists by helping detect abnormalities, highlighting potential problem areas, and classifying images quickly and accurately.
|
||||
|
||||
For instance, AI algorithms trained on vast image datasets can diagnose conditions such as pneumonia or tuberculosis in chest X-rays with a remarkable level of accuracy. Automated image analysis not only saves time but also reduces the chances of human error in interpreting complex images, ultimately leading to better and more reliable diagnoses.
|
||||
|
||||
## Enhancing Telemedicine
|
||||
|
||||
The rise of telemedicine, accelerated by the COVID-19 pandemic, has presented new challenges in healthcare delivery. However, AI has stepped in to provide innovative solutions by facilitating remote diagnosis and bridging gaps in healthcare access.
|
||||
|
||||
AI-powered chatbots and virtual assistants assist patients in self-triage, directing them to the appropriate level of care based on their symptoms. These AI tools can analyze a patient's reported symptoms, medical history, and risk factors to generate informed suggestions. Furthermore, remote monitoring devices powered by AI algorithms can track vital signs and identify significant changes that require medical attention, enabling healthcare providers to intervene proactively.
|
||||
|
||||
## Conclusion
|
||||
|
||||
Artificial Intelligence is transforming healthcare diagnosis in unimaginable ways. By leveraging AI's capabilities in data analysis, pattern recognition, and machine learning, healthcare professionals can now offer more accurate and personalized diagnoses, leading to enhanced patient outcomes and improved overall healthcare delivery.
|
||||
|
||||
As AI continues to evolve, it holds the promise of further revolutionizing healthcare diagnosis, reducing medical errors, improving treatment efficacy, and ultimately saving more lives. The synergy between AI and healthcare is shaping a future where diagnoses are faster, more precise, and accessible to all, enabling a healthier society for generations to come.
|
@ -1,31 +0,0 @@
|
||||
# How AI is Revolutionizing Human Resources (HR)
|
||||
|
||||
Artificial Intelligence (AI) has rapidly emerged as a game-changer in various industries, and Human Resources (HR) is no exception. With its advanced capabilities, AI has the potential to revolutionize traditional HR practices and streamline the entire employee lifecycle. From recruitment to onboarding, performance management, and employee engagement, AI is transforming HR functions by automating time-consuming tasks, improving decision-making processes, and enhancing overall efficiency.
|
||||
|
||||
## Transforming Recruitment Processes
|
||||
|
||||
Recruiting the right talent is a critical aspect of HR, and AI is significantly simplifying and enhancing the recruitment process by providing valuable insights and automated solutions. AI-powered platforms can scan and analyze numerous resumes in seconds, matching them with desired skill sets and qualifications. This saves HR professionals countless hours spent manually screening resumes and allows them to focus on more strategic tasks.
|
||||
|
||||
Furthermore, AI algorithms can evaluate interviewees' facial expressions, tone of voice, and choice of words during video interviews. This analysis helps in identifying potential cultural or personality mismatches with the organization, enabling HR teams to make better hiring decisions.
|
||||
|
||||
## Enabling Objective Performance Evaluations
|
||||
|
||||
Traditional performance evaluations are often subject to bias and can be inconsistent. AI solutions are addressing these challenges by introducing objective and data-driven performance evaluations. With the help of AI algorithms, HR professionals can analyze various data points, such as productivity levels, sales figures, and customer satisfaction ratings. This data-driven analysis ensures that performance evaluations are fair and based on concrete evidence, promoting a more transparent and equitable work environment.
|
||||
|
||||
## Enhancing Employee Experience and Engagement
|
||||
|
||||
Employee experience and engagement are crucial for maintaining a productive workforce. AI tools and chatbots are being implemented to streamline employee queries, providing instant responses to commonly asked questions about policies, benefits, or procedures. These virtual assistants free up HR professionals from repetitive queries, allowing them to focus on more strategic initiatives that foster employee engagement and retention.
|
||||
|
||||
AI is also revolutionizing employee feedback systems. Traditional annual employee surveys are being replaced by continuous feedback mechanisms facilitated by AI. Sentiment analysis tools can gauge employees' moods by analyzing their emails, workplace chat messages, or other forms of communication. This enables HR teams to identify potential issues, address concerns in real-time, and make data-driven decisions to improve overall employee experience and engagement.
|
||||
|
||||
## Personalizing Learning and Development
|
||||
|
||||
AI technology is empowering HR departments to deliver personalized learning and development (L&D) experiences. AI-powered systems can analyze employees' skill sets, learning styles, and career paths to offer customized training programs. By understanding individual learning preferences and providing tailored content, AI optimizes the effectiveness of L&D initiatives, enhancing the learning experience for employees.
|
||||
|
||||
## Improving HR Analytics and Decision-Making
|
||||
|
||||
Data plays a crucial role in informed decision-making, and AI is revolutionizing HR analytics. AI algorithms can efficiently analyze vast amounts of HR data, identify patterns, predict attrition rates, and even recognize early warning signs of employee burnout or disengagement. This enables HR professionals to take proactive measures to retain top talent, identify skill gaps, and optimize workforce planning.
|
||||
|
||||
Ultimately, AI enhances HR professionals' ability to make data-driven decisions, transforming them into strategic partners who create value for the organization.
|
||||
|
||||
In conclusion, AI is revolutionizing Human Resources by automating labor-intensive tasks, providing data-driven insights, and streamlining various HR functions. By leveraging AI technology, HR departments can focus more on strategic initiatives, improving employee experience, enabling better decision-making, and ultimately driving organizational success.
|
@ -1,35 +0,0 @@
|
||||
# AI Revolutionizing Language Learning Apps
|
||||
|
||||

|
||||
|
||||
In recent years, there has been a remarkable transformation in the way we learn languages, thanks to the integration of artificial intelligence (AI) into language learning applications. With the advent of AI, these apps have become more personalized, interactive, and effective, providing learners with an immersive language learning experience. Let's explore how AI is revolutionizing language learning apps.
|
||||
|
||||
## Intelligent Tutoring
|
||||
|
||||
Traditional language learning involved a one-size-fits-all approach, where learners had to follow a preset curriculum regardless of their individual needs and proficiency levels. AI has completely transformed this approach. Language learning apps now utilize AI algorithms to assess learners' abilities, interests, and learning styles. Based on this data, AI-powered apps provide personalized tutoring experiences tailored to individual learners. Learners can receive recommendations on what to learn next, access relevant resources, and interact with virtual tutors who adapt to their progress.
|
||||
|
||||
## Natural Language Processing
|
||||
|
||||
Understanding and using natural language is at the core of language learning. AI-powered language learning apps use natural language processing (NLP) algorithms to understand learners' speech and provide accurate feedback. Voice recognition capabilities allow learners to practice speaking the language and receive instant feedback on pronunciation and grammar. With AI, learners can engage in meaningful conversations with chatbots or virtual assistants, further enhancing their language skills.
|
||||
|
||||
## Adaptive Learning
|
||||
|
||||
Another significant contribution of AI to language learning apps is adaptive learning. AI algorithms track learners' progress and identify areas where they need improvement. By continuously analyzing learner data, AI-driven apps adapt the curriculum and learning materials to focus on those weak areas. Adaptive learning ensures that learners progress at their own pace, reinforcing their knowledge and addressing their specific weaknesses. This tailor-made approach increases learning efficiency and overall retention.
|
||||
|
||||
## Gamification and Personalization
|
||||
|
||||
AI has also brought gamification elements to language learning apps, making the process more engaging and enjoyable. By leveraging AI algorithms, apps can create personalized learning games, quizzes, and challenges that are specifically designed to enhance language skills. Gamification not only motivates learners but also creates an immersive environment where learners can practice the language while having fun.
|
||||
|
||||
## Conversational Practice
|
||||
|
||||
Language learners often face challenges in finding real-life opportunities to practice their speaking skills. AI-powered language learning apps have introduced conversational practice through chatbots or virtual language partners. These virtual entities engage in conversations with learners, providing them with an opportunity to practice speaking in a judgment-free environment. With AI's ability to simulate human-like conversations, learners can practice various scenarios and improve their fluency and conversational skills.
|
||||
|
||||
## Enhanced Content Recommendations
|
||||
|
||||
AI algorithms play a crucial role in suggesting relevant content to learners. By analyzing users' behavior, preferences, and language proficiency, language learning apps recommend appropriate reading materials, podcasts, videos, and other resources to enhance the learning experience. These content recommendations ensure that learners have access to authentic and engaging materials that align with their interests and level of proficiency.
|
||||
|
||||
## Future Possibilities
|
||||
|
||||
As AI continues to evolve, the future of language learning apps looks even more promising. With advancements in machine learning and natural language understanding, AI-driven apps may achieve near-human-like conversation capabilities, supporting learners in even more realistic language practice scenarios. Additionally, AI can enable apps to assess learners' emotions, shaping their learning experience accordingly and providing a more personalized and empathetic approach.
|
||||
|
||||
In conclusion, AI has revolutionized language learning apps, offering learners a personalized, immersive, and effective learning experience. From intelligent tutoring to adaptive learning and gamification, AI-driven apps have redefined how we learn languages. With the ongoing advancements in AI technology, language learning is expected to become even more engaging, personalized, and efficient in the future.
|
@ -1,31 +0,0 @@
|
||||
# How AI is Revolutionizing Language Translation Services
|
||||
|
||||
Language translation services have come a long way in breaking down language barriers and connecting people from different parts of the world. With advancements in Artificial Intelligence (AI), language translation has not only become more accurate but also faster and more accessible than ever before. AI-powered translation technology is revolutionizing the way we communicate globally, bridging the gap between languages and cultures.
|
||||
|
||||
## Enhanced Accuracy
|
||||
|
||||
AI has significantly enhanced the accuracy of language translation services. Traditional translation tools relied on word-to-word or phrase-to-phrase translations, often resulting in awkward and inaccurate translations that failed to capture the true meaning and intent of the original text. Machine Learning algorithms in AI have changed the game by allowing the technology to learn from vast amounts of data and create more contextually accurate translations.
|
||||
|
||||
Neural Machine Translation (NMT), a technique used in AI-powered translation models, uses deep learning algorithms to analyze and understand the context of the entire sentence, rather than simply translating word by word. This enables the system to produce more coherent and accurate translations, capturing the nuances and idiomatic expressions that are often lost in word-based translations.
|
||||
|
||||
## Faster Translation
|
||||
|
||||
AI has also significantly expedited the translation process. Human translators require a considerable amount of time to translate lengthy documents or multiple documents in quick succession. AI-powered translation services equipped with NMT models leverage parallel computing power to process translation tasks faster than ever before.
|
||||
|
||||
With the ability to process an immense amount of data simultaneously, AI provides almost real-time translation services, making communication between individuals speaking different languages more efficient and seamless. This speed is especially valuable in domains like customer service, where quick responses are crucial.
|
||||
|
||||
## More Languages Supported
|
||||
|
||||
Traditionally, language translation services were limited to popular languages, leaving many languages underrepresented. AI has revolutionized this aspect by making translation services available for a wide range of languages. Machine Learning algorithms enable AI models to learn and understand multiple languages, making translations accessible and accurate for less common and minority languages as well.
|
||||
|
||||
This expansion of language support has broad implications, especially in business and international diplomacy, where communication across various languages is critical. AI-driven translation services empower businesses to reach a global audience and facilitate collaboration between people from diverse linguistic backgrounds.
|
||||
|
||||
## User-Friendly Translation Tools
|
||||
|
||||
Thanks to AI technology, translation services have become more user-friendly and accessible. AI-powered translation applications and tools provide seamless integration with various devices, including smartphones and tablets. Users can simply type or dictate the content they want to be translated and receive instant translations in their desired language.
|
||||
|
||||
Mobile applications, incorporating AI-powered translation, enable travelers to overcome language barriers more easily by translating signs, menus, or conversations in real-time. This accessibility is invaluable in creating a more interconnected and inclusive world.
|
||||
|
||||
## Conclusion
|
||||
|
||||
The impact of AI on language translation services cannot be overstated. From improving accuracy to expediting the translation process and supporting a wider range of languages, AI is reshaping the way we communicate and breaking down language barriers on a global scale. As AI continues to evolve, we can expect even more sophisticated translation services that will further enhance intercultural connections and foster mutual understanding across borders.
|
@ -1,31 +0,0 @@
|
||||
# How AI is Revolutionizing Learning Analytics in Education
|
||||
|
||||

|
||||
|
||||
Artificial Intelligence (AI) is increasingly making a significant impact on various industries, and education is no exception. One area where AI is transforming education is learning analytics. By analyzing large amounts of data, AI-powered systems can provide educators with valuable insights to enhance teaching methods, personalize learning experiences, and improve student outcomes. Let's explore how AI is revolutionizing learning analytics in education.
|
||||
|
||||
## Personalized Adaptive Learning
|
||||
|
||||
In traditional education systems, teachers are often limited in their ability to customize learning experiences for each student. However, with AI-driven learning analytics, educators can gain a deep understanding of each learner's strengths, weaknesses, and progress. By leveraging this data, AI systems can create personalized learning paths for individual students. These personalized adaptive learning approaches help students learn at their own pace, ensuring that they receive targeted support and practice in areas where they struggle, ultimately leading to improved outcomes.
|
||||
|
||||
## Early Identification of Learning Difficulties
|
||||
|
||||
AI algorithms can analyze vast amounts of data, including student performance records, behavior patterns, engagement levels, and more. This enables AI systems to identify potential learning difficulties at an early stage. By detecting patterns that indicate struggling students, educators can intervene promptly, offering customized support and intervention strategies. Early identification means students can receive the necessary help before falling behind, increasing their chances of success.
|
||||
|
||||
## Intelligent Content Recommendation
|
||||
|
||||
AI-powered content recommendation systems have become an integral part of many online platforms, including e-learning platforms. These systems analyze user behavior, preferences, and learning patterns to provide personalized content recommendations. By leveraging AI's ability to understand the unique needs and interests of students, educators can ensure they receive relevant and engaging learning materials, which can significantly enhance their learning experience and motivation.
|
||||
|
||||
## Predictive Analytics for Student Success
|
||||
|
||||
By analyzing historical student data, AI can identify factors that contribute to student success. AI-driven predictive analytics models can assess various parameters, such as attendance, engagement, grades, and demographic information. With this information, educators can predict the likelihood of students' success or failure and take proactive measures accordingly. Early intervention based on predictive analytics enables educators to implement targeted support systems, ultimately increasing students' chances of achieving their goals.
|
||||
|
||||
## Intelligent Tutoring Systems
|
||||
|
||||
AI-powered intelligent tutoring systems are rapidly gaining popularity in education. These systems leverage machine learning algorithms to provide personalized, one-on-one tutoring to students. By constantly analyzing student performance and adapting instruction based on individual needs, intelligent tutoring systems can provide tailored support to maximize learning outcomes. These systems use natural language processing, computer vision, and other AI technologies to interact with students, answer their questions, and provide explanations, offering a highly adaptive and personalized learning experience.
|
||||
|
||||
## Ethical Considerations and Challenges
|
||||
|
||||
While AI revolutionizes learning analytics in education, it also raises some ethical considerations and challenges. Protecting student data privacy, ensuring fairness and transparency in algorithmic decision-making, and addressing biases are just a few of the concerns that need careful attention. Educators, policymakers, and developers need to collaborate to establish ethical frameworks, guidelines, and regulations to leverage the benefits of AI while safeguarding students' rights and well-being.
|
||||
|
||||
In conclusion, AI is revolutionizing learning analytics in education by offering personalized adaptive learning experiences, early identification of learning difficulties, intelligent content recommendations, predictive analytics for student success, and intelligent tutoring systems. Leveraging the power of AI, educators can enhance teaching methods, provide targeted support, and improve student outcomes. However, it is crucial to address the ethical considerations and challenges that arise with the integration of AI in education to ensure responsible and beneficial use for all.
|
@ -1,39 +0,0 @@
|
||||
# AI Revolutionizing Legal Document Analysis
|
||||
|
||||
*Artificial Intelligence (AI) has become a game-changer in various industries, and the legal sector is no exception. By leveraging AI technology, legal professionals can now handle and analyze vast amounts of legal documents more efficiently and accurately, saving time and resources. This article explores how AI is revolutionizing legal document analysis and its significant impact in the legal world.*
|
||||
|
||||
## Enhancing Efficiency
|
||||
|
||||
In the legal field, analyzing documents is a time-consuming and labor-intensive task. Legal professionals spend countless hours reviewing contracts, agreements, and other legal texts to extract crucial information. However, with AI-powered document analysis tools, this process has been greatly accelerated and streamlined.
|
||||
|
||||
AI algorithms can swiftly process and categorize large volumes of legal documents, significantly reducing the time and effort required. By automating mundane and repetitive tasks, legal professionals can focus on more complex and strategic aspects of their work.
|
||||
|
||||
## Accurate Data Extraction
|
||||
|
||||
Legal documents often contain critical information buried within paragraphs and sections, making it cumbersome to identify and extract the necessary data. AI systems equipped with natural language processing (NLP) capabilities can efficiently identify and extract key information from legal texts.
|
||||
|
||||
Using techniques like machine learning and text recognition, AI algorithms can pinpoint relevant clauses, dates, names, and other significant details in legal documents. This accurate data extraction not only saves time but also minimizes the risk of human error, ensuring the precision and reliability of extracted information.
|
||||
|
||||
## Improved Due Diligence
|
||||
|
||||
AI-based document analysis has revolutionized the due diligence process in legal matters. During corporate mergers, acquisitions, or similar transactions, conducting a thorough review of legal documents is vital. AI-powered systems can quickly analyze vast datasets, identifying potential risks, loopholes, or inconsistencies that legal professionals might overlook.
|
||||
|
||||
By examining extensive volumes of legal documents, AI algorithms enable legal teams to make more informed decisions and provide comprehensive reports on the potential risks and implications. This not only enhances the due diligence process but also helps in mitigating any legal risks associated with the transaction.
|
||||
|
||||
## Streamlined Legal Research
|
||||
|
||||
Historical legal cases, court decisions, and precedents play a crucial role in forming legal arguments and strategies. However, manually researching relevant case law and statutes can be tedious and time-consuming. AI-powered legal research tools have revolutionized this process by automating the search and analysis across vast legal databases.
|
||||
|
||||
AI algorithms can quickly retrieve and summarize large volumes of legal texts, providing lawyers with relevant case law, precedents, and legal opinions. This expedites the research process and enables lawyers to build stronger arguments based on comprehensive legal analysis.
|
||||
|
||||
## Ensuring Compliance
|
||||
|
||||
Compliance with laws, regulations, and contractual obligations is of utmost importance for any organization. AI-powered document analysis can play a significant role in ensuring compliance by automatically reviewing legal documents against predefined rules and regulations.
|
||||
|
||||
By comparing legal texts against a set of rules, AI systems can identify any potential violations or inconsistencies. This enables organizations to proactively identify and address compliance issues and minimize legal risks in real-time.
|
||||
|
||||
## Conclusion
|
||||
|
||||
The adoption of AI in legal document analysis has revolutionized the way legal professionals handle and process vast amounts of legal texts. By automating tasks, extracting accurate data, improving due diligence, streamlining research, and ensuring compliance, AI has become an invaluable assistant to legal practitioners.
|
||||
|
||||
While AI technology does not replace the expertise and judgment of legal professionals, it significantly enhances their efficiency and effectiveness. As AI continues to evolve, it will further transform the legal industry, allowing legal professionals to focus on higher-value tasks and deliver better outcomes for their clients.
|
@ -1,27 +0,0 @@
|
||||
# How AI is Revolutionizing Natural Language Processing (NLP)
|
||||
|
||||
Artificial Intelligence (AI) has been making significant strides in the field of Natural Language Processing (NLP) in recent years, revolutionizing how machines understand and interact with human language. NLP is a branch of AI that focuses on the interaction between computers and human language, enabling machines to understand, interpret, and respond to natural language input.
|
||||
|
||||
## Enhanced Language Understanding
|
||||
|
||||
One of the key areas where AI is transforming NLP is in language understanding. Traditional NLP models often struggled with context, understanding idioms, sarcasm, and nuanced language. However, with the advent of AI, deep learning algorithms coupled with neural networks have greatly enhanced language understanding capabilities. AI algorithms are now able to analyze huge amounts of text data, learn patterns, and discern the underlying meaning, providing more accurate and contextually relevant results.
|
||||
|
||||
## Machine Translation
|
||||
|
||||
AI-powered NLP is also revolutionizing machine translation, making language barriers a thing of the past. Neural machine translation models employ advanced AI techniques to translate text from one language to another, providing more accurate and coherent translations. Google's Neural Machine Translation (GNMT) system is a prime example of how AI is transforming translation services, enabling faster and more precise translations across multiple languages.
|
||||
|
||||
## Natural Language Interfaces
|
||||
|
||||
The rise of AI in NLP has also spurred the development of natural language interfaces, allowing users to interact with machines using spoken or written language. Virtual assistants like Siri, Alexa, and Google Assistant leverage advanced NLP algorithms to understand user queries, retrieve relevant information, and provide appropriate responses. These AI-powered interfaces have made human-machine interaction more intuitive and seamless, enhancing user experience across various devices and platforms.
|
||||
|
||||
## Sentiment Analysis
|
||||
|
||||
AI has empowered NLP systems with the ability to perform sentiment analysis, which involves identifying and understanding the emotions behind text. Sentiment analysis algorithms analyze text data to determine whether the expressed sentiment is positive, negative, or neutral. This has wide-ranging applications in social media monitoring, customer feedback analysis, and brand reputation management. By employing AI, businesses can gain invaluable insights into customer sentiment, allowing them to make data-driven decisions and improve customer satisfaction.
|
||||
|
||||
## Text Summarization and Generation
|
||||
|
||||
Another area where AI is revolutionizing NLP is in text summarization and generation. AI models, such as GPT-3 (Generative Pre-trained Transformer 3), can generate human-like text by training on vast amounts of data. They can summarize lengthy texts, extract important information, and even generate content based on prompts. AI-powered text generation has the potential to streamline content creation, assist in research, and increase efficiency in various industries.
|
||||
|
||||
## Conclusion
|
||||
|
||||
Artificial Intelligence has unleashed a new era of progress and innovation in Natural Language Processing. With advanced algorithms and neural networks, machines are now able to understand human language, translate with greater accuracy, analyze sentiment, and generate coherent text. As AI continues to evolve, we can expect further breakthroughs that will revolutionize how we interact with machines, opening up new possibilities and transforming various industries with the power of Natural Language Processing.
|
@ -1,26 +0,0 @@
|
||||
# How AI is Revolutionizing Online Gaming
|
||||
|
||||
Artificial Intelligence (AI) has become an integral part of our lives, transforming various industries across the globe. One of the areas where AI is making significant strides is online gaming. As technology advances, AI is revolutionizing the way we play, compete, and interact in the digital realm. Let's take a closer look at how AI is reshaping the world of online gaming.
|
||||
|
||||
## Enhancing Gameplay Experience
|
||||
AI technology has the potential to enrich the overall gameplay experience. Traditional games rely on pre-programmed responses and actions, limiting the player's interaction with the game environment. However, AI brings in dynamic decision-making capabilities, allowing games to adapt and respond to individual actions, creating a sense of realism and engagement.
|
||||
|
||||
By incorporating machine learning algorithms, AI-powered games can learn from player behavior and adapt the gameplay accordingly. This ability offers endless possibilities, as games can evolve and adjust the difficulty level based on each player's skills, making the experience personalized and more challenging.
|
||||
|
||||
## Smarter Non-Player Characters (NPCs)
|
||||
NPCs are an essential part of any gaming experience, providing challenges, storyline progression, and autonomous interactions within the game world. AI enables the development of smarter NPCs that can act more human-like, enhancing the immersion of the players.
|
||||
|
||||
Using AI algorithms, NPCs can now exhibit advanced decision-making and reasoning abilities, making their behavior more realistic and unpredictable. They can detect patterns, remember player actions, and create responses accordingly. This makes the game world feel more dynamic and alive, providing players with a truly immersive experience.
|
||||
|
||||
## Real-time Analytics and Anti-cheating
|
||||
Online gaming often faces challenges such as cheating, where players try to gain an unfair advantage using illegal methods. AI has stepped in to tackle this issue by providing real-time analytics and anti-cheating measures.
|
||||
|
||||
AI algorithms can detect suspicious behavior patterns, automatically identify cheaters, and even predict potential cheating attempts. This enables game developers to take necessary action promptly, maintaining the fairness and integrity of the gaming environment. With AI's help, game developers can now create a more secure and enjoyable online gaming experience for honest players.
|
||||
|
||||
## Personalized Recommendations and Matchmaking
|
||||
Another significant way AI is improving online gaming is through personalized recommendations and matchmaking systems. By analyzing player behavior data, AI algorithms can suggest games or in-game content that align with individual preferences and play styles. This customization enhances the gaming experience, ensuring players are exposed to content that suits their interests.
|
||||
|
||||
AI-powered matchmaking systems also play a crucial role in enhancing the competitive aspect of online gaming. By considering factors such as players' skill levels, preferences, and historical performance, AI can create balanced matches, leading to fair and exciting gameplay. This improves player satisfaction and encourages them to continue playing, fostering a vibrant online gaming community.
|
||||
|
||||
## Conclusion
|
||||
AI is undoubtedly revolutionizing the online gaming landscape, providing exciting advancements and opportunities. From enhancing gameplay experience and creating smarter NPCs to tackling cheating and providing personalized recommendations, AI is transforming how we play and interact in the virtual world. As AI technology continues to evolve, we can expect even more innovative and immersive online gaming experiences in the future.
|
@ -1,31 +0,0 @@
|
||||
# How AI is Revolutionizing Personalized Marketing
|
||||
|
||||
Artificial Intelligence (AI) has become an integral part of our day-to-day lives, making tasks easier, faster, and more efficient. Personalized marketing is no exception to the transformative power of AI. With its ability to analyze vast amounts of data and generate insights in real-time, AI is revolutionizing personalized marketing strategies like never before.
|
||||
|
||||
## Understanding Customer Behavior
|
||||
|
||||
One of the primary benefits of AI in personalized marketing is its ability to understand customer behavior at a granular level. By analyzing data from various sources such as social media, browsing patterns, purchase history, and demographics, AI algorithms can identify patterns and preferences unique to each individual. This enables marketers to tailor their campaigns based on specific customer needs and preferences.
|
||||
|
||||
## Enhanced Customer Segmentation
|
||||
|
||||
AI-powered tools help marketers segment their customer base more effectively. Rather than relying on broad categories, AI algorithms are capable of creating micro-segments by clustering individuals based on behavior, interests, and preferences. This level of granularity allows for more targeted marketing campaigns, ensuring that customers receive highly relevant content and offers.
|
||||
|
||||
## Hyper-Personalized Recommendations
|
||||
|
||||
Gone are the days of generic recommendations. AI algorithms can generate hyper-personalized product recommendations by analyzing customer data to understand their preferences and needs. By leveraging machine learning and predictive analytics, AI can suggest products or services that customers are most likely to purchase, increasing conversion rates and customer satisfaction.
|
||||
|
||||
## Real-Time Campaign Optimization
|
||||
|
||||
AI's ability to process information in real-time enables marketers to optimize their campaigns on the fly. By monitoring response rates, click-through rates, and conversions, AI algorithms can identify underperforming campaigns and suggest immediate adjustments. This dynamic approach allows marketers to continuously improve their strategies, ensuring that their messages resonate with customers in the most effective way.
|
||||
|
||||
## Chatbots for Personalized Customer Engagement
|
||||
|
||||
AI-powered chatbots have revolutionized customer engagement by providing personalized experiences 24/7. These chatbots leverage natural language processing and machine learning to understand customer inquiries and provide instant responses. By utilizing customer data and past interactions, chatbots can offer tailored recommendations, resolve issues, and provide a seamless customer experience.
|
||||
|
||||
## Ethical Considerations
|
||||
|
||||
While AI offers immense potential for personalized marketing, it is important to address ethical considerations. With access to vast amounts of customer data, marketers have a responsibility to enact robust data protection measures. Transparency, consent, and ensuring data privacy are critical to building trust with customers and using AI technology responsibly.
|
||||
|
||||
## Conclusion
|
||||
|
||||
AI's ability to process and analyze massive amounts of data is transforming personalized marketing in significant ways. From understanding customer behavior to hyper-personalized recommendations, AI enables marketers to create more targeted, engaging, and effective campaigns. Chatbots enhance customer engagement, while real-time campaign optimization ensures maximum impact. However, ethical considerations must accompany the use of AI in personalized marketing to maintain customer trust and privacy. As AI continues to evolve, personalized marketing will undoubtedly reach new heights, benefiting both businesses and customers alike.
|
@ -1,26 +0,0 @@
|
||||
# How AI is Revolutionizing Predictive Analytics
|
||||
|
||||
Artificial Intelligence (AI) is making significant advances across various industries, and one area where it is proving to be incredibly valuable is in predictive analytics. By leveraging AI technologies, businesses can gain deep insights into data patterns, predict future trends, and make well-informed decisions. Here, we explore how AI is revolutionizing the field of predictive analytics.
|
||||
|
||||
## Enhanced Data Processing
|
||||
AI algorithms have the ability to process vast amounts of data in real-time, far beyond the capabilities of human analysts. Predictive analytics requires extensive data analysis to identify patterns, correlations, and anomalies. With AI, data processing becomes faster, more accurate, and scalable. AI algorithms can identify hidden patterns and connections that may not be noticeable to human analysts, improving the accuracy of predictions.
|
||||
|
||||
## Advanced Machine Learning
|
||||
One of the core components of predictive analytics is machine learning (ML), and AI brings unprecedented advancements to this field. ML algorithms can learn from large datasets, automatically detect patterns, and adapt their models accordingly. AI-powered predictive analytics systems continuously improve their accuracy by learning from new data. This enables organizations to make data-driven decisions based on reliable predictions.
|
||||
|
||||
## Automated Predictive Modeling
|
||||
Generating accurate predictive models requires expertise and extensive statistical knowledge. With AI, the process of building models is automated, reducing human effort and error. AI algorithms can automatically select the most appropriate model based on the data and business problem at hand. This means that even organizations with limited data science resources can harness the power of predictive analytics.
|
||||
|
||||
## Improved Customer Insights
|
||||
AI-powered predictive analytics provides businesses with new ways to understand their customers. By analyzing vast amounts of customer data, such as browsing history, purchase patterns, and demographics, AI algorithms can predict customer behavior with high precision. This allows companies to personalize marketing campaigns, improve customer segmentation, and make targeted recommendations, resulting in enhanced customer satisfaction and loyalty.
|
||||
|
||||
## Fraud Detection and Risk Management
|
||||
Predictive analytics plays a crucial role in fraud detection and risk management across various industries. AI algorithms can analyze vast amounts of data, including transaction records and customer behavior, to detect anomalies and identify potential risks. By continuously monitoring patterns and detecting suspicious activities in real-time, AI-powered systems can significantly reduce fraud and mitigate risks more effectively.
|
||||
|
||||
## Healthcare and Disease Prediction
|
||||
AI is transforming the healthcare industry by improving disease prediction and early detection. Predictive analytics algorithms can analyze patient data, medical records, and genetic information to identify patterns indicating the likelihood of certain diseases. This enables healthcare professionals to intervene at an early stage, improve patient outcomes, and allocate resources efficiently.
|
||||
|
||||
## Conclusion
|
||||
AI is revolutionizing the field of predictive analytics, enabling organizations to make data-driven decisions and gain a competitive edge. With enhanced data processing capabilities, advanced machine learning techniques, and automated predictive modeling, AI empowers businesses to uncover valuable insights from large datasets. Moreover, AI-powered predictive analytics can enhance customer insights, facilitate fraud detection, and drive advancements in healthcare. As AI continues to evolve, its impact on predictive analytics will undoubtedly continue to reshape industries and drive innovation.
|
||||
|
||||
> "Artificial intelligence will reach human levels by around 2029. Follow that out further to, say, 2045, and we will have multiplied the intelligence - the human biological machine intelligence of our civilization - a billion-fold." - Ray Kurzweil
|
@ -1,26 +0,0 @@
|
||||
# How AI is Revolutionizing Predictive Maintenance
|
||||
|
||||

|
||||
|
||||
Predictive maintenance is a crucial aspect of many industries, ensuring efficient operations and minimizing downtime. Traditionally, maintenance activities were based on scheduled intervals, often resulting in unnecessary costs and inconveniences. However, thanks to advancements in Artificial Intelligence (AI) and Machine Learning (ML), predictive maintenance has witnessed a drastic transformation, leading to improved reliability, reduced costs, and enhanced efficiency.
|
||||
|
||||
AI algorithms have the ability to process large volumes of data from machinery and equipment, identifying patterns and predicting potential failures before they occur. This transformative technology has revolutionized the way maintenance operations function, making them more proactive and responsive. Here's how AI is reshaping predictive maintenance:
|
||||
|
||||
## 1. Condition Monitoring and Anomaly Detection
|
||||
AI-powered systems can continuously monitor equipment conditions by collecting and processing real-time data. By utilizing ML algorithms, it becomes possible to analyze various parameters such as temperature, vibration, pressure, and more. Anomalies and deviations from normal operating conditions are immediately flagged, enabling operators to perform preventive actions, reducing the risk of downtime and costly repairs.
|
||||
|
||||
## 2. Predicting Remaining Useful Life (RUL)
|
||||
One of the significant advantages of AI in predictive maintenance is its capability to predict the remaining useful life of machinery and equipment accurately. By assessing historical data, operating conditions, and patterns of failure, AI algorithms can estimate the remaining operational lifespan of assets. This allows businesses to optimize maintenance activities, performing necessary repairs or replacements just in time, minimizing downtime and maximizing equipment utilization.
|
||||
|
||||
## 3. Intelligent Fault Diagnosis
|
||||
AI enables intelligent fault diagnosis by using historical maintenance records and real-time data. By analyzing patterns and correlations, these algorithms can identify the root causes of failures and provide accurate diagnoses. This reduces the time and effort involved in diagnosing issues manually, allowing maintenance teams to quickly resolve problems, resulting in faster turnaround times and increased uptime.
|
||||
|
||||
## 4. Optimal Maintenance Strategies
|
||||
Implementing an optimal maintenance strategy is crucial for efficient operations. AI systems leverage data-driven insights to determine the most effective maintenance approach for each asset. By considering factors such as asset criticality, cost of repairs, availability of spare parts, and workload, AI algorithms can recommend maintenance plans that minimize costs while ensuring optimal performance. This enables organizations to allocate resources effectively while avoiding unnecessary maintenance activities and associated expenses.
|
||||
|
||||
## 5. Improved Supply Chain Management
|
||||
AI-enabled predictive maintenance also impacts supply chain management positively. By analyzing the predicted failure patterns of equipment, organizations can proactively manage their spare parts inventory. Stocking the right parts in the right quantities allows for reduced lead times, minimizing downtime in the event of unexpected failures. This preventive approach optimizes the procurement process and lowers inventory costs while maintaining operational continuity.
|
||||
|
||||
Overall, AI has revolutionized predictive maintenance by making it more data-driven, proactive, and efficient. By leveraging AI algorithms and ML techniques, organizations can prioritize maintenance activities, reduce costs, and maximize equipment availability. Embracing this transformative technology empowers industries to ensure reliable operations, minimize downtime, and stay ahead in an increasingly competitive marketplace.
|
||||
|
||||
*[ML]: Machine Learning
|
@ -1,52 +0,0 @@
|
||||
# Use Cases
|
||||
|
||||
- [3D_Printing_usecase.md](3D_Printing_usecase.md)
|
||||
- [Agricultural_AI_usecase.md](Agricultural_AI_usecase.md)
|
||||
- [Astronomical_Research_usecase.md](Astronomical_Research_usecase.md)
|
||||
- [Augmented_Reality_(AR)_usecase.md](Augmented_Reality_(AR)_usecase.md)
|
||||
- [Autonomous_Drones_usecase.md](Autonomous_Drones_usecase.md)
|
||||
- [Bioinformatics_usecase.md](Bioinformatics_usecase.md)
|
||||
- [Chatbots_and_Virtual_Assistants_usecase.md](Chatbots_and_Virtual_Assistants_usecase.md)
|
||||
- [Content_Creation_usecase.md](Content_Creation_usecase.md)
|
||||
- [Credit_Scoring_usecase.md](Credit_Scoring_usecase.md)
|
||||
- [Customer_Segmentation_usecase.md](Customer_Segmentation_usecase.md)
|
||||
- [Cybersecurity_usecase.md](Cybersecurity_usecase.md)
|
||||
- [Drug_Discovery_usecase.md](Drug_Discovery_usecase.md)
|
||||
- [E-commerce_Visual_Recognition_usecase.md](E-commerce_Visual_Recognition_usecase.md)
|
||||
- [E-learning_Platforms_usecase.md](E-learning_Platforms_usecase.md)
|
||||
- [Elderly_Care_Robotics_usecase.md](Elderly_Care_Robotics_usecase.md)
|
||||
- [Energy_Consumption_Optimization_usecase.md](Energy_Consumption_Optimization_usecase.md)
|
||||
- [Facial_Recognition_usecase.md](Facial_Recognition_usecase.md)
|
||||
- [Fraud_Detection_usecase.md](Fraud_Detection_usecase.md)
|
||||
- [Handwriting_Recognition_usecase.md](Handwriting_Recognition_usecase.md)
|
||||
- [Healthcare_Diagnosis_usecase.md](Healthcare_Diagnosis_usecase.md)
|
||||
- [Human_Resources_(HR)_usecase.md](Human_Resources_(HR)_usecase.md)
|
||||
- [Language_Learning_Apps_usecase.md](Language_Learning_Apps_usecase.md)
|
||||
- [Language_Translation_Services_usecase.md](Language_Translation_Services_usecase.md)
|
||||
- [Learning_Analytics_in_Education_usecase.md](Learning_Analytics_in_Education_usecase.md)
|
||||
- [Legal_Document_Analysis_usecase.md](Legal_Document_Analysis_usecase.md)
|
||||
- [Natural_Language_Processing_(NLP)_usecase.md](Natural_Language_Processing_(NLP)_usecase.md)
|
||||
- [Online_Gaming_usecase.md](Online_Gaming_usecase.md)
|
||||
- [Personalized_Marketing_usecase.md](Personalized_Marketing_usecase.md)
|
||||
- [Predictive_Analytics_usecase.md](Predictive_Analytics_usecase.md)
|
||||
- [Predictive_Maintenance_usecase.md](Predictive_Maintenance_usecase.md)
|
||||
- [Recommendation_Systems_usecase.md](Recommendation_Systems_usecase.md)
|
||||
- [Retail_Inventory_Management_usecase.md](Retail_Inventory_Management_usecase.md)
|
||||
- [Robotics_in_Manufacturing_usecase.md](Robotics_in_Manufacturing_usecase.md)
|
||||
- [Search_Engine_Optimization_(SEO)_usecase.md](Search_Engine_Optimization_(SEO)_usecase.md)
|
||||
- [Seismic_Interpretation_usecase.md](Seismic_Interpretation_usecase.md)
|
||||
- [Self-Driving_Cars_usecase.md](Self-Driving_Cars_usecase.md)
|
||||
- [Sentiment_Analysis_usecase.md](Sentiment_Analysis_usecase.md)
|
||||
- [Simulation_and_Modeling_usecase.md](Simulation_and_Modeling_usecase.md)
|
||||
- [Smart_Agriculture_usecase.md](Smart_Agriculture_usecase.md)
|
||||
- [Smart_Grids_usecase.md](Smart_Grids_usecase.md)
|
||||
- [Smart_Home_Devices_usecase.md](Smart_Home_Devices_usecase.md)
|
||||
- [Social_Media_Monitoring_usecase.md](Social_Media_Monitoring_usecase.md)
|
||||
- [Speech_Recognition_usecase.md](Speech_Recognition_usecase.md)
|
||||
- [Supply_Chain_Optimization_usecase.md](Supply_Chain_Optimization_usecase.md)
|
||||
- [Telemedicine_usecase.md](Telemedicine_usecase.md)
|
||||
- [Traffic_Management_usecase.md](Traffic_Management_usecase.md)
|
||||
- [Virtual_Reality_(VR)_usecase.md](Virtual_Reality_(VR)_usecase.md)
|
||||
- [Voice-Activated_Assistants_usecase.md](Voice-Activated_Assistants_usecase.md)
|
||||
- [Waste_Management_usecase.md](Waste_Management_usecase.md)
|
||||
- [Weather_Forecasting_usecase.md](Weather_Forecasting_usecase.md)
|
@ -1,37 +0,0 @@
|
||||
# How AI is Revolutionizing Recommendation Systems
|
||||
|
||||
Artificial Intelligence (AI) has become an integral part of our daily lives, revolutionizing various industries and sectors. One area where AI is making a significant impact is in the field of recommendation systems. These systems analyze and predict user preferences, making personalized recommendations for products, services, content, and more. With advancements in AI, recommendation systems have become smarter, more accurate, and efficient than ever before.
|
||||
|
||||
## Understanding Recommendation Systems
|
||||
|
||||
Recommendation systems are algorithms that are designed to predict and suggest items that users are likely to be interested in. Such systems are heavily relied upon by companies like e-commerce platforms, streaming services, and social media platforms to enhance user experience and engagement.
|
||||
|
||||
Two key types of recommendation systems are commonly used: collaborative filtering and content-based filtering. Collaborative filtering determines recommendations based on the preferences of similar users, while content-based filtering uses information about the items themselves to make suggestions.
|
||||
|
||||
## How AI Enhances Recommendation Systems
|
||||
|
||||
AI has greatly enhanced recommendation systems by leveraging advanced algorithms and techniques. Here's how it is revolutionizing the field:
|
||||
|
||||
### 1. Improved Personalization
|
||||
|
||||
AI enables recommendation systems to provide highly personalized suggestions based on individual user behavior, preferences, and history. By analyzing vast amounts of data, AI algorithms can understand intricate patterns and make accurate predictions. Consequently, users receive recommendations tailored specifically to their interests, increasing user satisfaction and engagement.
|
||||
|
||||
### 2. Enhanced Accuracy
|
||||
|
||||
Traditional recommendation systems often suffer from limitations such as "cold start" problems (when a system has minimal user data) and poor generalization. AI algorithms address these shortcomings by combining various data sources, including demographic information, past purchases, browsing patterns, and even social media data. This comprehensive approach results in more accurate and reliable recommendations that are highly aligned with user preferences.
|
||||
|
||||
### 3. Dynamic Learning and Adaptation
|
||||
|
||||
AI-powered recommendation systems possess the ability to dynamically adapt and learn from user feedback. These systems continuously improve by capturing user interactions, analyzing their responses to recommendations, and modifying future suggestions accordingly. As a result, the recommendations become increasingly precise and relevant over time.
|
||||
|
||||
### 4. Handling Complexity
|
||||
|
||||
With the proliferation of information and choices available to users, recommendation systems face the challenge of dealing with vast and diverse datasets. AI techniques like deep learning and natural language processing help tackle these complexities. Deep learning models can extract valuable features from unstructured data, such as reviews or textual descriptions, to make more insightful suggestions.
|
||||
|
||||
### 5. Expanding Beyond Traditional Boundaries
|
||||
|
||||
AI-driven recommendation systems are expanding beyond traditional sectors like e-commerce and media to a wide range of industries. We can now see recommendation systems in sectors such as healthcare, finance, and education. For example, AI-powered healthcare recommendation systems can suggest personalized treatment plans based on patients' medical history and symptoms, leading to improved outcomes and patient care.
|
||||
|
||||
## Conclusion
|
||||
|
||||
AI has revolutionized recommendation systems, making them more accurate, efficient, and personalized. By harnessing the power of advanced algorithms, machine learning, and data analysis, AI enables recommendation systems to provide tailored suggestions that engage users and enhance their overall experience. As AI continues to evolve, we can expect further advancements in recommendation systems, ultimately benefiting both users and businesses across different industries.
|
@ -1,31 +0,0 @@
|
||||
# AI Revolutionizing Retail Inventory Management
|
||||
|
||||

|
||||
|
||||
Artificial Intelligence (AI) technology has expanded its influence across numerous industries, bringing significant advancements and transforming traditional operations. One area where AI is making a significant impact is retail inventory management. With AI-enabled systems, retailers can streamline and optimize their inventory processes, leading to improved customer experience, minimized costs, and increased profitability.
|
||||
|
||||
## Real-time Data Analysis
|
||||
|
||||
AI-powered retail inventory management utilizes machine learning algorithms to analyze vast amounts of historical sales data and real-time customer insights. This allows retailers to gain better visibility into their inventory levels, identifying patterns, trends, and customer preferences. By collecting and processing data from multiple sources, AI systems can accurately predict future demand, enabling retailers to plan their inventory accordingly.
|
||||
|
||||
## Forecasting and Predictive Analytics
|
||||
|
||||
AI-driven inventory management systems excel in forecasting to optimize stock levels. By analyzing historical data, market trends, and external factors, such as holidays or promotions, AI algorithms can accurately predict demand for specific products. This helps retailers avoid stockouts or overstocking, reducing wastage and ensuring timely reordering. Ultimately, these insights enhance inventory turnover rates and ultimately boost revenue.
|
||||
|
||||
## Automation and Efficiency
|
||||
|
||||
Traditionally, retailers would need to manually monitor stock levels, perform inventory counts, and replenish items. With AI, these time-consuming tasks are automated, allowing employees to focus on more value-added activities. AI-powered robots and drones can scan store shelves and warehouses, regularly reporting stock levels and identifying missing or misplaced items. This automation significantly reduces human errors, ensures accurate inventory counts, and enhances the efficiency of stock management processes.
|
||||
|
||||
## Enhanced Supply Chain Management
|
||||
|
||||
AI also plays a crucial role in improving supply chain management within retail organizations. By integrating AI with inventory management systems, retailers can optimize every step of the supply chain, from order processing to product delivery. AI analyzes real-time data from multiple suppliers, helping retailers identify bottlenecks, select the most efficient shipping routes, and monitor delivery timelines. This not only ensures timely delivery but also minimizes fulfillment costs and enhances customer satisfaction.
|
||||
|
||||
## Personalized Customer Experience
|
||||
|
||||
AI-powered inventory management enables retailers to cater to individual customer preferences and provide personalized shopping experiences. By analyzing customer behavior and purchase history, AI algorithms can recommend relevant products to shoppers. This personalized approach not only enhances customer satisfaction but also increases revenue generation. Furthermore, AI chatbots can assist customers with inventory-related queries in real-time, improving their overall shopping experience.
|
||||
|
||||
## Rapid Detection of Fraud and Theft
|
||||
|
||||
AI is instrumental in detecting and preventing inventory-related fraud and theft, which can significantly impact retailers' profitability. Intelligent algorithms monitor patterns, anomalies, and unusual activities within the supply chain or in-store operations. These systems can identify instances of internal theft, shoplifting, or other fraudulent activities promptly. By raising alarms in real-time, AI helps retailers mitigate losses and enhances the overall security of their inventory.
|
||||
|
||||
AI-driven retail inventory management is revolutionizing the industry by optimizing operations, improving efficiency, and enhancing customer experiences. As technology continues to advance, retailers must embrace AI to stay competitive and leverage its power in streamlining their inventory processes. With real-time data analysis, advanced forecasting, efficient automation, optimized supply chain management, and better customer experiences, AI is set to redefine retail inventory management and shape the future of the retail industry.
|
@ -1,39 +0,0 @@
|
||||
# How AI is Revolutionizing Robotics in Manufacturing
|
||||
|
||||
Artificial Intelligence (AI) has been making significant breakthroughs in various industries and has become a game-changer in many ways. One such field that has been greatly impacted by AI is robotics in manufacturing. The combination of AI and robotics has brought forth immense benefits, making manufacturing processes more efficient, precise, and adaptive. Let's explore how AI is revolutionizing robotics in manufacturing.
|
||||
|
||||
## Enhanced automation and accuracy
|
||||
|
||||
One of the key advantages of integrating AI into robotics in manufacturing is enhanced automation. AI-powered robots possess advanced machine vision capabilities that allow them to perceive and understand their environment. With the help of computer vision systems and deep learning algorithms, these robots can identify and classify objects, perform quality inspections, and even make critical decisions.
|
||||
|
||||
This advanced level of automation ensures that manufacturing processes are executed with precision, eliminating errors and reducing the need for human intervention. AI-driven robots can perform complex tasks with high accuracy, leading to improved product quality and minimized waste.
|
||||
|
||||
## Smart and adaptive robots
|
||||
|
||||
AI algorithms enable robots to learn from their interactions with the environment and adapt accordingly. Advanced robotic systems equipped with AI can analyze huge amounts of data generated during the manufacturing process. By leveraging this data, robots can optimize their performance, detect and correct errors, and continuously refine their operations.
|
||||
|
||||
Machine learning algorithms enable robots to identify patterns, make predictions, and optimize their workflows. This adaptability allows manufacturers to respond quickly to changing demands, introduce new product variants, and minimize production downtime. AI-powered robots can quickly optimize their processes based on real-time feedback, ensuring maximum efficiency.
|
||||
|
||||
## Collaborative robots (cobots)
|
||||
|
||||
Collaborative robots, known as cobots, are designed to work alongside humans, enhancing productivity and promoting safer manufacturing environments. AI plays a crucial role in enabling cobots to adapt to human presence and collaborate effectively.
|
||||
|
||||
Using AI, cobots can detect and respond to human actions, ensuring safe operation. Machine learning algorithms enable robots to understand human intentions and perform collaborative tasks accordingly. This collaboration between humans and robots enhances productivity while ensuring worker safety.
|
||||
|
||||
## Predictive maintenance
|
||||
|
||||
AI-driven robots can monitor their own performance and identify potential problems before they impact production. By utilizing machine learning algorithms and advanced analytics, robots can predict maintenance requirements and alert manufacturers before failures occur.
|
||||
|
||||
This proactive approach to maintenance reduces unplanned downtime, optimizing production schedules and minimizing losses. Predictive maintenance powered by AI helps manufacturers save costs associated with reactive maintenance and ensures smooth manufacturing operations.
|
||||
|
||||
## Increased efficiency and productivity
|
||||
|
||||
By integrating AI into robotics, manufacturers can achieve significant improvements in efficiency and productivity. AI-powered robots can work 24/7 without fatigue, maintaining consistent high-quality production rates. They can handle repetitive and dangerous tasks with precision, allowing human workers to focus on more complex and creative assignments.
|
||||
|
||||
Furthermore, AI-driven robots can analyze data from the manufacturing process and identify areas for optimization. They can suggest process changes, automate workflows, and improve overall efficiency. This leads to increased productivity and better utilization of resources.
|
||||
|
||||
## Conclusion
|
||||
|
||||
The integration of AI into robotics has unlocked immense potential in manufacturing. It has empowered robots to perform tasks with enhanced automation, precision, and adaptability. The collaboration between humans and AI-driven robots has increased both productivity and safety in manufacturing environments.
|
||||
|
||||
With further advancements in AI, robotics in manufacturing will continue to evolve, transforming the industry and paving the way for smart factories of the future. As AI continues to revolutionize robotics, manufacturers stand to reap numerous benefits, including improved efficiency, productivity, and overall quality.
|
@ -1,28 +0,0 @@
|
||||
# How AI is Revolutionizing Search Engine Optimization (SEO)
|
||||
|
||||

|
||||
|
||||
Artificial intelligence (AI) has emerged as a game-changer in various industries, and one area it is making a significant impact is in the field of search engine optimization (SEO). AI technologies are transforming the way businesses optimize their websites to improve search engine rankings, increase organic traffic, and enhance user experience. Let's explore how AI is revolutionizing SEO.
|
||||
|
||||
## Enhanced Keyword Research
|
||||
Keywords are the foundation of SEO, and AI-powered tools are making keyword research smarter and more efficient. AI algorithms analyze vast amounts of data to identify user search patterns, intent, and behavior. This enables SEO professionals to discover relevant keywords with high search volume and low competition more accurately. AI tools can suggest long-tail keywords, create semantic clusters, and even predict future keyword trends.
|
||||
|
||||
## Content Generation and Optimization
|
||||
AI is transforming content creation by enabling automated generation and optimization of website pages, blog posts, and articles. Natural Language Processing (NLP) algorithms allow AI systems to analyze and understand human language, resulting in highly relevant and engaging content. AI-powered tools can also ensure proper keyword usage, improve readability, and provide suggestions for optimizing content based on search engine algorithms.
|
||||
|
||||
## Advanced Competitor Analysis
|
||||
Understanding the competition is crucial for SEO success. AI-powered tools can gather and analyze vast amounts of data related to competitor websites, backlink profiles, and content strategies. By examining this information, AI systems can provide valuable insights and recommendations to help businesses stay ahead in the SEO game. These tools can identify gaps in keyword targeting, highlight potential link-building opportunities, and reveal content strategies that perform well.
|
||||
|
||||
## Efficient On-Site Optimization
|
||||
AI is assisting in automating and streamlining on-site optimization processes. This includes optimizing meta tags, headers, image alt tags, and other HTML elements to improve relevance and crawlability. AI algorithms can identify technical issues, such as broken links or slow-loading pages, which negatively impact user experience and search engine rankings. By flagging these issues, AI tools help SEO professionals prioritize improvements and achieve better website performance.
|
||||
|
||||
## Personalized User Experience
|
||||
Providing a personalized user experience is becoming increasingly important for SEO. AI algorithms analyze user behavior, preferences, and historical data to deliver personalized search results. This helps businesses tailor their content, offers, and website design to meet individual user needs. By understanding user intent, AI-powered SEO tools can optimize websites for voice search and mobile devices, contributing to increased user engagement and conversions.
|
||||
|
||||
## Effective Link Building
|
||||
AI-powered tools are revolutionizing the way link building is done. They analyze the relevance, authority, and quality of different websites to identify potential link partners. These tools can assess the credibility of websites and determine the potential value of obtaining backlinks from them. AI algorithms can also identify toxic or spammy links that could harm a website's reputation and provide suggestions for disavowing such links.
|
||||
|
||||
## Predictive Analytics
|
||||
AI analyzes large volumes of data to provide predictive insights for SEO strategies. By examining historical data, search trends, and algorithm updates, AI-powered tools can forecast future SEO performance. These predictive analytics help businesses stay ahead of the competition and make informed decisions regarding their SEO efforts. Whether it's predicting keyword performance, estimating organic traffic growth, or analyzing the impact of algorithm changes, AI can guide SEO professionals towards effective strategies.
|
||||
|
||||
In conclusion, AI is a powerful tool that is revolutionizing the way SEO is conducted. From keyword research and content optimization to competitor analysis and predictive analytics, AI technologies are enabling businesses to achieve higher search engine rankings, increase organic traffic, and enhance user experience. As AI continues to advance, the possibilities for SEO improvement are vast and exciting.
|
@ -1,29 +0,0 @@
|
||||
# How AI is Revolutionizing Seismic Interpretation
|
||||
|
||||
## Introduction
|
||||
|
||||
Seismic interpretation plays a crucial role in the oil and gas industry by providing valuable insights into earth subsurface structures and potential hydrocarbon reservoirs. Historically, this process has been highly manual and time-consuming, relying on the expertise of geoscientists to analyze seismic data in detail. However, with the emergence of Artificial Intelligence (AI) technology, seismic interpretation is undergoing a major revolution. AI is bringing automation, efficiency, and accuracy to this critical domain, transforming the way geoscientists work and ushering in a new era of exploration and production.
|
||||
|
||||
## Improved Data Processing and Analysis
|
||||
|
||||
AI algorithms are designed to process and analyze vast amounts of seismic data quickly and efficiently. By leveraging machine learning techniques, AI models can automatically recognize patterns, features, and anomalies within seismic data that might have been overlooked by human analysts. These algorithms can be trained on massive datasets, enabling them to learn from historical seismic interpretations and predict likely subsurface structures, fault lines, and potential reservoir locations.
|
||||
|
||||
## Enhanced Interpretation Accuracy
|
||||
|
||||
One of the significant advantages of AI in seismic interpretation is its ability to improve accuracy. AI models can analyze a wide range of seismic attributes simultaneously, leading to a multi-dimensional interpretation that captures subtle subsurface details. By reducing human bias and subjective errors, AI systems provide geoscientists with more reliable and consistent interpretations. This not only saves time but also enhances decision-making processes when it comes to well placement, drilling locations, and reservoir mapping.
|
||||
|
||||
## Accelerated Workflow
|
||||
|
||||
Traditional seismic interpretation workflows can be time-consuming, as geoscientists meticulously analyze seismic data and manually interpret subsurface structures. AI technology drastically shortens this timeline by automating several stages of the interpretation process. AI systems can quickly process large seismic datasets, analyze them for potential patterns, and produce initial interpretations. Geoscientists can then validate and refine these interpretations, significantly accelerating the overall workflow.
|
||||
|
||||
## Data-Driven Insights
|
||||
|
||||
AI-powered seismic interpretation enables geoscientists to gain valuable insights from vast amounts of seismic data. By analyzing historical datasets, AI models can identify trends, anomalies, and previously unseen patterns in subsurface structures. This empowers geoscientists with a more comprehensive understanding of the geological makeup of a region. Furthermore, AI can integrate data from multiple sources, such as well logs, production data, and geological surveys, to provide a holistic view of the subsurface environment.
|
||||
|
||||
## Intelligent Risk Assessment
|
||||
|
||||
AI technology supports intelligent risk assessment in seismic interpretation. By analyzing seismic attributes, past drilling results, and production data, AI models can identify high-risk areas or areas with a high potential for hydrocarbon accumulation. Geoscientists can then focus their efforts on these areas, optimizing exploration and determining the most suitable drilling locations. This data-driven risk assessment allows for better resource allocation, reducing costs and increasing the overall success rate of hydrocarbon extraction.
|
||||
|
||||
## Conclusion
|
||||
|
||||
Artificial Intelligence is revolutionizing seismic interpretation by automating data processing, improving interpretation accuracy, accelerating workflows, providing data-driven insights, and enabling intelligent risk assessment. This technology empowers geoscientists to make more informed decisions, optimize exploration efforts, and unlock new hydrocarbon reservoirs effectively. With the continuous advancements in AI, the future of seismic interpretation holds tremendous potential for enhanced productivity, increased discovery rates, and improved efficiency in the oil and gas industry.
|
@ -1,23 +0,0 @@
|
||||
# How AI is Revolutionizing Self-Driving Cars
|
||||
|
||||
|
||||
## Introduction
|
||||
Self-driving cars, once only seen in science fiction movies, have become a reality with the help of Artificial Intelligence (AI). AI is revolutionizing the automotive industry, enabling vehicles to navigate and drive themselves with minimal or no human intervention. With advanced algorithms and intelligent systems, self-driving cars are transforming the way we commute, making transportation safer, more efficient, and convenient.
|
||||
|
||||
## Enhanced Safety
|
||||
One of the primary advantages of self-driving cars powered by AI is their potential to significantly reduce road accidents. Autonomous vehicles rely on a combination of cameras, radar, Lidar sensors, and AI algorithms to perceive and interpret their surroundings, making split-second decisions as human drivers would. This technology minimizes human errors caused by distractions, fatigue, or impaired judgment, leading to safer roads for everyone.
|
||||
|
||||
## Efficient Traffic Management
|
||||
AI-powered self-driving cars have the potential to alleviate traffic congestion. Sophisticated AI algorithms can analyze real-time traffic data from various sources, including other vehicles, traffic lights, and road infrastructure. By optimizing routes, adjusting speeds, and coordinating with traffic systems, self-driving cars can navigate more efficiently, reducing commute times and congestion on roads.
|
||||
|
||||
## Improved Accessibility
|
||||
Self-driving cars have the potential to revolutionize transportation accessibility for individuals who are unable to drive conventional vehicles, such as the elderly, people with disabilities, or those without a driver's license. AI technology enables these individuals to gain independence and mobility, enhancing their quality of life. Furthermore, self-driving taxi services could provide affordable and reliable transportation options for those who cannot afford car ownership.
|
||||
|
||||
## Environmental Benefits
|
||||
With growing concerns about climate change and environmental impact, self-driving cars powered by AI can help reduce carbon emissions. By optimizing routes and driving patterns, self-driving cars can minimize fuel consumption and reduce the overall environmental footprint of transportation. Moreover, the increased adoption of electric self-driving cars would further contribute to cleaner and greener mobility.
|
||||
|
||||
## Addressing Challenges
|
||||
While AI is revolutionizing self-driving cars, there are still challenges to overcome. Developing advanced AI algorithms capable of handling complex and unpredictable scenarios remains a significant hurdle. Ensuring the security and privacy of data collected by self-driving cars is another concern. Furthermore, defining regulatory frameworks and legal standards for autonomous vehicles present ongoing challenges that need to be addressed to ensure a smooth transition to AI-driven transportation.
|
||||
|
||||
## Conclusion
|
||||
Artificial Intelligence is revolutionizing the automotive industry by enabling self-driving cars. Enhanced safety, efficient traffic management, improved accessibility, and environmental benefits are just a few of the advantages that AI brings to autonomous vehicles. Although there are challenges to overcome, the ongoing development and integration of AI into self-driving cars hold immense potential for transforming transportation as we know it. As this technology continues to evolve, we can look forward to a future where self-driving cars become the new norm.
|
@ -1,39 +0,0 @@
|
||||
# How AI is Helping and Revolutionizing Sentiment Analysis
|
||||
|
||||
In the era of big data and social media, businesses are constantly seeking insights into the thoughts, feelings, and opinions of their customers. Sentiment analysis, also known as opinion mining, is a powerful technology that has emerged to address this need. Utilizing artificial intelligence (AI), sentiment analysis has revolutionized the way organizations process and interpret vast amounts of textual data to extract valuable insights.
|
||||
|
||||
## Understanding Sentiment Analysis
|
||||
|
||||
Sentiment analysis is the process of determining the sentiment or emotional tone within a piece of text. It involves analyzing and classifying data into positive, negative, or neutral sentiments, allowing businesses to gauge public opinion towards their products, services, or brand.
|
||||
|
||||
Traditionally, sentiment analysis relied on manual methods, which were not only time-consuming but also prone to human bias. However, with advancements in AI, sentiment analysis has greatly improved in terms of accuracy, scalability, and speed.
|
||||
|
||||
## AI-Driven Sentiment Analysis
|
||||
|
||||
Artificial intelligence algorithms, particularly natural language processing (NLP) models, have played a significant role in revolutionizing sentiment analysis. These algorithms can not only comprehend raw text but also identify and interpret contextual nuances, slang, and even sarcasm.
|
||||
|
||||
By leveraging machine learning and deep learning techniques, AI models are trained on vast datasets, enabling them to learn and recognize patterns and understand sentiment at a granular level. As a result, the accuracy and reliability of sentiment analysis have greatly improved, providing businesses with highly valuable and actionable insights.
|
||||
|
||||
## Applications in Business
|
||||
|
||||
AI-powered sentiment analysis has a wide range of applications across various industries. Some of the significant areas where sentiment analysis is helping and revolutionizing business processes include:
|
||||
|
||||
### 1. Brand Monitoring and Reputation Management
|
||||
|
||||
Sentiment analysis allows businesses to monitor online conversations about their brand in real-time. By analyzing social media posts, customer reviews, and online forums, companies can gain valuable insights into how their brand is perceived by the public. This information can be used to identify potential issues, respond to customer concerns, and make data-driven decisions to improve brand reputation.
|
||||
|
||||
### 2. Customer Feedback and Market Research
|
||||
|
||||
Sentiment analysis enables businesses to analyze customer feedback and reviews more efficiently. By understanding the sentiment expressed in these interactions, companies can identify common pain points, measure customer satisfaction, and gain insights into customer preferences. This information is invaluable when it comes to making informed decisions regarding product development, marketing strategies, and enhancing overall customer experience.
|
||||
|
||||
### 3. Competitor Analysis
|
||||
|
||||
AI-driven sentiment analysis allows businesses to gain a competitive edge by monitoring and analyzing sentiment towards their competitors. By understanding customer opinions about rival products or services, companies can identify gaps in the market, capitalize on strengths, and stay ahead of the competition.
|
||||
|
||||
### 4. Social Media Marketing and Influencer Campaigns
|
||||
|
||||
Sentiment analysis helps businesses assess the effectiveness of social media marketing campaigns. By analyzing the sentiment of social media mentions and engagement, companies can measure the impact of their marketing efforts and make necessary adjustments to optimize results. Additionally, sentiment analysis can assist in identifying relevant influencers and monitoring their influence on brand sentiment.
|
||||
|
||||
## Conclusion
|
||||
|
||||
Artificial intelligence has completely revolutionized sentiment analysis, enabling businesses to extract meaningful insights from vast amounts of textual data. By leveraging AI algorithms, companies can understand public sentiment, improve brand reputation, enhance customer experiences, and gain a competitive advantage. As AI technology continues to advance, sentiment analysis will continue to evolve, providing businesses with even deeper insights into the thoughts and opinions of their customers.
|
@ -1,31 +0,0 @@
|
||||
# How AI is Revolutionizing Simulation and Modeling
|
||||
|
||||
Artificial intelligence (AI) has emerged as a game-changing technology that is transforming various industries. One area where AI is making significant strides is simulation and modeling. With its ability to process vast amounts of data, learn from patterns, and make intelligent decisions, AI is revolutionizing the way simulations and models are developed, improving their accuracy, efficiency, and effectiveness.
|
||||
|
||||
## Enhanced Accuracy and Predictability
|
||||
|
||||
Traditionally, simulations and modeling have relied on algorithms and mathematical equations to replicate real-world scenarios. However, these models often had limitations due to their inability to account for complex variables and unpredictable events. AI changes the game by enabling simulations that learn from data and adjust their algorithms based on patterns and feedback.
|
||||
|
||||
By analyzing historical data and recognizing hidden patterns, AI algorithms can optimize the accuracy of models. This enhanced accuracy facilitates better predictions through simulations, helping researchers and experts make more informed decisions in a variety of fields, such as climate forecasting, financial modeling, and risk analysis.
|
||||
|
||||
## Faster Simulations and Reduced Costs
|
||||
|
||||
AI-powered simulations and modeling systems can significantly speed up the entire process, reducing the time it takes to create complex models. Leveraging advanced machine learning techniques, AI algorithms automate and optimize parts of the simulation process. This automation eliminates the need for manual intervention, reducing errors and saving valuable time.
|
||||
|
||||
Moreover, AI-powered simulations often require fewer computational resources, which results in reduced costs. Traditional simulations often demanded expensive infrastructure and lengthy processing times. AI streamlines these requirements by making simulations more efficient and effective, enabling organizations to accomplish more with fewer resources.
|
||||
|
||||
## Intelligent Decision-Making
|
||||
|
||||
AI's ability to learn from vast datasets and identify patterns enables it to make intelligent decisions within simulations and models. It provides valuable insights into complex scenarios, helps identify optimal solutions, and assists in predicting various outcomes. These AI-enabled decisions empower researchers and decision-makers in fields such as supply chain management, urban planning, and healthcare.
|
||||
|
||||
Furthermore, AI can simulate and model scenarios that are challenging or even dangerous to replicate in real-life. For instance, AI-powered medical simulations can assist in training healthcare professionals for complex surgeries or emergency situations, minimizing risks and improving patient outcomes.
|
||||
|
||||
## Optimizing System Design and Performance
|
||||
|
||||
Simulation and modeling are fundamental in designing and optimizing complex systems. AI can analyze vast amounts of data and simulations, identifying areas of improvement and optimizing system designs. By learning from historical performance data, AI algorithms can recommend modifications, upgrades, or changes that enhance the overall performance of various systems.
|
||||
|
||||
AI's ability to adapt and improve continuously through machine learning allows simulations and models to evolve alongside changing requirements, resulting in systems that are better equipped to handle real-world challenges.
|
||||
|
||||
## Conclusion
|
||||
|
||||
AI is revolutionizing simulation and modeling by enhancing accuracy, predictability, and efficiency. With its ability to process immense amounts of data, identify patterns, and make intelligent decisions, AI is transforming various industries that rely on simulations and models. By reducing costs, saving time, and optimizing system designs, AI empowers researchers and professionals to make more informed decisions and achieve improved outcomes. The future of simulation and modeling is undoubtedly intertwined with the growth and advancements of AI technology.
|
@ -1,29 +0,0 @@
|
||||
# AI Revolutionizing Smart Agriculture
|
||||
|
||||

|
||||
|
||||
The rapid advancement of Artificial Intelligence (AI) has opened up countless opportunities across various industries, and one such sector greatly benefiting from this revolution is agriculture. With the help of AI technologies, the field of smart agriculture is witnessing radical changes, improving efficiency, productivity, and sustainability. Let's explore how AI is transforming the face of agriculture.
|
||||
|
||||
## Enhancing Crop Monitoring and Analysis
|
||||
|
||||
AI-powered drones and satellites equipped with advanced cameras and sensors enable real-time crop monitoring on a large scale. By capturing high-resolution images and collecting data related to crop health, moisture levels, and nutrient deficiencies, AI algorithms can quickly analyze the information to generate valuable insights. This technology aids farmers in detecting early signs of diseases, pests, or crop stress, allowing for precise and timely intervention. By addressing issues promptly, AI helps optimize crop yield and reduce loss.
|
||||
|
||||
## Predictive Analytics for Weather Forecasting
|
||||
|
||||
Accurate weather forecasting is crucial for farmers to make informed decisions regarding irrigation, fertilization, and crop protection. AI algorithms trained on historical weather data can predict weather patterns with greater accuracy, enabling farmers to plan farming activities accordingly. By leveraging these predictive analytics models, farmers can optimize water usage, minimize the effects of adverse weather conditions, and enhance resource management on their farms.
|
||||
|
||||
## Precision Farming and Resource Optimization
|
||||
|
||||
AI-powered smart agriculture systems utilize various sensors like soil moisture sensors, temperature sensors, and humidity sensors to monitor and optimize resource usage. AI algorithms can analyze sensor data along with external data sources like weather forecasting to recommend the optimal amount of water, fertilizer, and pesticide required for specific crops and soil conditions. This approach minimizes resource wastage, reduces production costs, while ensuring the overall health and productivity of the farm.
|
||||
|
||||
## Robotic Farming and Harvesting
|
||||
|
||||
The integration of AI and robotics is transforming traditional farming methods. Automated robots equipped with AI technologies can perform labor-intensive tasks like planting seeds, weeding, and harvesting crops with precision and efficiency. AI algorithms enable robots to identify and differentiate between crops, detect ripeness levels, and accurately harvest produce, saving significant time and labor costs for farmers.
|
||||
|
||||
## Livestock Monitoring and Health Management
|
||||
|
||||
AI is also revolutionizing livestock farming by offering advanced monitoring and health management solutions. Wearable devices equipped with AI-enabled sensors can continuously monitor parameters like heart rate, body temperature, and feed consumption. AI algorithms can interpret the obtained data in real-time, identify anomalies, and alert farmers about the health conditions of individual animals or the entire herd. This proactive approach allows farmers to take immediate action, prevent disease outbreaks, and optimize livestock care.
|
||||
|
||||
## Conclusion
|
||||
|
||||
As the global population continues to grow, the demand for sustainable and efficient agricultural practices becomes more pressing. AI-driven technologies and systems, such as crop monitoring, predictive analytics, precision farming, robotic automation, and livestock management, are transforming the agricultural landscape. By leveraging the power of AI, farmers can make data-driven decisions, increase productivity, and minimize environmental impact. The synergy of AI and smart agriculture holds great potential to feed the world's growing population while ensuring sustainable and efficient food production.
|
@ -1,28 +0,0 @@
|
||||
# How AI is Revolutionizing Smart Grids
|
||||
|
||||
> "The development of artificial intelligence has a profound impact on various industries, and one area where it is proving to be a game-changer is in the transformation of smart grids. AI-powered technologies are enhancing the efficiency, reliability, and sustainability of energy distribution, thereby revolutionizing the way we manage and consume electricity."
|
||||
|
||||
With the rapid increase in energy demand and the growing emphasis on sustainability, smart grids have emerged as a critical component of modern power systems. These digital networks enable two-way communication between power utilities, consumers, and decentralized energy sources, facilitating the better integration and control of electricity generation, distribution, and consumption.
|
||||
|
||||
Artificial intelligence (AI) has become an integral part of enhancing the capabilities of smart grids. By leveraging machine learning algorithms, deep neural networks, and predictive analytics, AI empowers grid operators to make informed and optimized decisions in real-time, leading to significant improvements in energy efficiency, cost savings, and resilience.
|
||||
|
||||
### Demand Response and Energy Management
|
||||
AI algorithms enable smart grids to predict electricity demand accurately. By analyzing historical usage data, weather conditions, and various external factors, AI algorithms can forecast energy demand at different timescales. This prediction helps grid operators optimize supply, match generation capacity with demand, and prevent grid instability or blackouts.
|
||||
|
||||
Moreover, AI applications assist consumers in managing their energy consumption effectively. Smart meters monitor real-time electricity usage and provide detailed information on energy consumption patterns. AI algorithms process this information, identify wasteful energy practices, and suggest energy-saving measures to consumers. Such insights empower individuals to make informed decisions about their electricity usage, resulting in reduced costs and a more sustainable lifestyle.
|
||||
|
||||
### Fault Detection and Self-Healing
|
||||
Smart grids integrated with AI technologies can monitor and analyze vast amounts of data in real-time to identify faults or anomalies in the power distribution network. By leveraging machine learning algorithms, AI can identify potential failures or abnormalities before they escalate into system-wide disruptions. Grid operators can then take proactive measures to isolate the issue and minimize downtime.
|
||||
|
||||
Furthermore, AI enables smart grids to self-heal by automatically reconfiguring the network to restore power after an outage or fault. By quickly identifying alternate routes or methods to reroute electricity, AI reduces downtime and significantly improves grid reliability.
|
||||
|
||||
### Integration of Renewable Energy Sources
|
||||
Renewable energy sources like solar panels and wind turbines are playing an increasingly important role in power generation. However, their intermittent nature poses additional challenges for grid operators. AI-based algorithms can predict and optimize the produced energy from renewable sources by considering various factors such as weather conditions, historical data, and predicted demand. This integration allows grid operators to balance fluctuations and ensure a stable power supply to consumers.
|
||||
|
||||
### Cybersecurity and Grid Operations
|
||||
With the increasing digitization of power systems, the threat of cyber attacks on smart grids becomes more significant. AI plays a vital role in strengthening grid cybersecurity. Machine learning algorithms help detect abnormal data patterns, identify potential security breaches, and alert operators in real-time. AI also assists in developing sophisticated encryption methods to protect sensitive data transmitted within the grid.
|
||||
|
||||
Additionally, AI-based approaches enhance grid operations by automating routine tasks, optimizing maintenance schedules, and streamlining energy distribution processes. This automation allows grid operators to allocate resources efficiently and respond swiftly to grid emergencies, ultimately reducing costs and improving customer satisfaction.
|
||||
|
||||
### Conclusion
|
||||
The integration of AI technologies in smart grids is transforming the electricity industry, unlocking numerous benefits and enabling a more sustainable future. From demand response and fault detection to renewable energy integration and cybersecurity, AI is revolutionizing the way we manage and operate power distribution networks. As ongoing advancements in AI continue to emerge, the full potential of smart grids can be realized, creating a more efficient, reliable, and environmentally friendly electrical infrastructure.
|
@ -1,24 +0,0 @@
|
||||
# How AI is Revolutionizing Smart Home Devices
|
||||
|
||||

|
||||
|
||||
**Introduction**
|
||||
In recent years, the integration of Artificial Intelligence (AI) has brought about a revolution in the realm of smart home devices. AI-powered technologies provide smart home devices with enhanced capabilities, enabling them to understand and adapt to human needs, making our lives more convenient, secure, and efficient. This article explores how AI is transforming smart home devices.
|
||||
|
||||
**Voice Assistants**
|
||||
One of the most notable advancements in smart home devices is the integration of voice assistants powered by AI. Voice-enabled assistants like Amazon Alexa, Google Assistant, and Apple Siri have become integral parts of many households. By processing natural language, these assistants can understand and execute commands, control other smart devices, provide information, and even entertain us. The AI behind these assistants continues to improve, becoming more accurate, efficient, and capable of understanding context, making them more reliable and user-friendly.
|
||||
|
||||
**Smart Home Security**
|
||||
AI is significantly enhancing the security features of smart home devices. AI-powered cameras and doorbell systems are not only capable of detecting motion but can also distinguish between humans, animals, and other objects. With facial recognition technology, they can even identify known individuals and alert homeowners accordingly. These systems can learn and adapt to the homeowner's preferences, distinguishing between normal activities and potential threats, thus reducing false alarms.
|
||||
|
||||
**Energy Management**
|
||||
AI-driven smart home devices are also revolutionizing energy management. By analyzing historical and real-time data, AI algorithms can regulate heating, ventilation, and air conditioning (HVAC) systems, optimizing energy usage according to the homeowner's preferences and schedule. These devices can automatically adjust the temperature, lighting, and other parameters based on occupancy patterns, weather conditions, and energy consumption patterns, resulting in significant energy savings.
|
||||
|
||||
**Smart Appliances and Devices**
|
||||
With AI, smart appliances and devices can learn and predict our behavior to enhance their functionality. For example, AI-enabled refrigerators can monitor our food inventory, suggest recipes based on available ingredients, and even place online grocery orders. AI-driven washing machines can automatically select the appropriate cycle, minimizing water and energy consumption. Similarly, AI-powered thermostats can learn our temperature preferences and adjust accordingly, ensuring optimal comfort.
|
||||
|
||||
**Personalized Interactions**
|
||||
AI-powered smart home devices can deliver personalized experiences to individual users. They can learn user preferences, adapt to their routines, and respond accordingly. For instance, AI-enabled lighting systems can adjust the color, intensity, and timing of lights based on individual preferences or activities. Virtual assistants can deliver personalized news updates, playlists, and even reminders tailored to each household member, enabling a more intuitive and customized smart home experience.
|
||||
|
||||
**Conclusion**
|
||||
The integration of AI into smart home devices is transforming our lives by providing increased convenience, security, energy efficiency, and personalization. With AI-powered voice assistants, security systems, energy management, appliances, and personalized interactions, our homes are becoming smarter and more responsive to our needs. As technology continues to advance, AI is set to revolutionize smart home devices further, making our lives even more comfortable and convenient.
|
@ -1,28 +0,0 @@
|
||||
# How AI is Revolutionizing Social Media Monitoring
|
||||
|
||||

|
||||
|
||||
Social media has become a powerful tool for individuals and businesses to express themselves, share information, and connect with others. With millions of posts being published every minute, it has become impossible for human operators to manually monitor and analyze the vast amount of data generated on these platforms. This is where artificial intelligence (AI) steps in.
|
||||
|
||||
AI technology has revolutionized the way social media monitoring is conducted. It enables companies to efficiently and effectively monitor conversations, gain insights, and make data-driven decisions to improve their campaigns, brand reputation, and customer service. Here's how AI is shaping the world of social media monitoring:
|
||||
|
||||
## Sentiment Analysis
|
||||
AI-powered sentiment analysis tools allow businesses to understand the sentiment behind social media mentions and comments. By analyzing the text, tone, and context, AI algorithms can accurately determine whether a post is positive, negative, or neutral. This provides companies with valuable insights about their customers' opinions, enabling them to promptly address complaints, identify trends, and adjust their strategies.
|
||||
|
||||
## Automating Responses
|
||||
AI chatbots have become an essential component in social media monitoring. They can engage in conversations, answer frequently asked questions, and provide assistance to customers. Advanced natural language processing capabilities allow chatbots to understand and respond to user inquiries in real-time. This way, businesses can ensure a seamless customer experience while saving time and resources.
|
||||
|
||||
## Crisis and Risk Management
|
||||
AI tools can detect potential crises or reputational risks by monitoring social media conversations in real-time. By using machine learning algorithms, these tools can identify patterns and trends that might generate negative sentiment and alert the appropriate stakeholders. This enables companies to respond quickly and effectively, mitigating any potential damage to their brand reputation.
|
||||
|
||||
## Influencer Identification
|
||||
Identifying influencers who can amplify a company's message is vital for social media marketing success. AI algorithms can sift through large amounts of data to identify relevant influencers based on their reach, engagement, and relevance to a particular industry. This eliminates the need for manual research and provides businesses with a curated list of potential influencers to collaborate with.
|
||||
|
||||
## Predictive Analytics
|
||||
AI-powered predictive analytics not only help monitor current social media conversations but also forecast future trends. By analyzing historical data, AI algorithms can identify patterns and predict possible outcomes. This helps businesses make proactive decisions and optimize their marketing strategies, leading to increased customer engagement and ROI.
|
||||
|
||||
## Enhanced Data Analysis
|
||||
Analyzing the massive amounts of data generated on social media platforms manually is nearly impossible. AI algorithms, however, excel in extracting valuable insights from vast datasets. They can identify trends, extract keywords, and discover correlations that might be challenging for humans to spot. This enables businesses to make data-driven decisions, identify new market opportunities, and deliver tailored marketing campaigns.
|
||||
|
||||
## Conclusion
|
||||
Artificial intelligence has revolutionized social media monitoring by providing businesses with advanced tools and capabilities previously unimaginable. From sentiment analysis to predictive analytics, AI algorithms can process and understand vast amounts of social media data, enabling companies to gain valuable insights, enhance customer experience, and make informed decisions. As AI continues to evolve, we can expect even more exciting advancements in social media monitoring, empowering businesses to succeed in the digital age.
|
@ -1,37 +0,0 @@
|
||||
# How AI is Revolutionizing Speech Recognition
|
||||
|
||||

|
||||
|
||||
Artificial Intelligence (AI) has made significant advancements in various fields, and one area that has seen remarkable growth is speech recognition. Through the combination of machine learning algorithms and deep neural networks, AI has revolutionized our ability to understand and interpret human speech.
|
||||
|
||||
## Enhanced Communication
|
||||
|
||||
Speech recognition technology has greatly enhanced communication by allowing machines to transcribe spoken words into written text accurately. This breakthrough has been particularly useful in various industries such as healthcare, customer service, and accessibility.
|
||||
|
||||
In healthcare, AI-powered speech recognition is being utilized to transcribe medical consultations, making it easier for doctors to create accurate patient records. With the help of AI, physicians can now spend more time providing care, rather than being occupied with documentation.
|
||||
|
||||
Customer service has also benefited from AI's speech recognition capabilities. Automated voice systems can now understand and process customer inquiries, reducing the need for human intervention. This not only improves customer satisfaction but also increases the efficiency of call centers.
|
||||
|
||||
Moreover, speech recognition has improved accessibility for individuals with disabilities. AI-powered virtual assistants like Siri, Alexa, and Google Assistant enable people to interact with their devices using voice commands alone. This technology allows visually impaired individuals to access information, control devices, and perform tasks with ease.
|
||||
|
||||
## Natural Language Processing
|
||||
|
||||
AI's speech recognition capabilities are constantly evolving, thanks to natural language processing (NLP) algorithms. NLP enables machines to understand context, infer meaning, and respond appropriately to spoken language.
|
||||
|
||||
With the help of AI, speech recognition systems can now accurately interpret spoken commands and queries, even when they involve complex language structures or regional accents. This breakthrough has revolutionized how we interact with technology and opened up new possibilities for human-machine communication.
|
||||
|
||||
The ability to understand human speech naturally has paved the way for voice-activated assistants and smart speakers. This technology is now integrated into our daily lives, allowing us to perform various tasks like setting reminders, playing music, and controlling our smart homes using voice commands alone.
|
||||
|
||||
## Continuous Improvement
|
||||
|
||||
AI's impact on speech recognition is continuously growing, mainly due to machine learning algorithms. These algorithms enable systems to learn from vast amounts of data, improving accuracy and reducing error rates over time.
|
||||
|
||||
By analyzing enormous datasets of human speech, AI systems can detect patterns, develop language models, and adapt to specific accents and dialects. This continuous learning process allows speech recognition technology to evolve and refine its performance with each interaction.
|
||||
|
||||
As the technology progresses, more industries are expected to adopt AI-powered speech recognition solutions. From transcription services and language learning applications to voice-controlled automation in vehicles, the possibilities are endless.
|
||||
|
||||
## Conclusion
|
||||
|
||||
AI has transformed speech recognition, empowering machines to understand and interpret human speech like never before. With improved accuracy, enhanced communication, and the ability to process natural language, AI-powered speech recognition technology has revolutionized numerous industries.
|
||||
|
||||
As AI continues to advance, we can expect more sophisticated speech recognition systems that will further streamline communication, enhance accessibility, and provide new opportunities across various sectors. The future of speech recognition is bright, thanks to the ongoing advancements in artificial intelligence.
|
@ -1,27 +0,0 @@
|
||||
# How AI is Revolutionizing Supply Chain Optimization
|
||||
|
||||
In today's fast-paced and competitive business environment, supply chain optimization plays a crucial role in ensuring operational efficiency and customer satisfaction. Traditionally, supply chain management involves complex decision-making processes, which can be overwhelming for human operators. However, with the advent of Artificial Intelligence (AI), businesses are able to revolutionize and streamline their supply chain operations like never before.
|
||||
|
||||
AI technologies, such as machine learning, natural language processing, and predictive analytics, have the potential to transform supply chain optimization by automating tasks, providing valuable insights, and enabling more intelligent decision-making. Let's explore how AI is helping to revolutionize supply chain management:
|
||||
|
||||
## Demand Forecasting and Planning
|
||||
|
||||
One of the key challenges in supply chain optimization is accurately forecasting demand and planning inventory levels accordingly. AI-powered algorithms are capable of analyzing vast amounts of historical data and external factors to predict future demand with greater accuracy. By taking into account various variables like customer behavior, market trends, and seasonal patterns, AI algorithms can help businesses optimize inventory levels, reduce costs, and minimize stockouts or overstocks.
|
||||
|
||||
## Intelligent Warehouse Management
|
||||
|
||||
AI-driven technology can enhance warehouse management processes, such as inventory management, order picking, and optimizing storage space. With the help of computer vision and machine learning, AI systems can identify and track inventory items, ensuring accurate stock levels, minimizing errors, and improving order fulfillment speed. Additionally, AI can optimize warehouse layout, product placement, and routing algorithms to reduce operational costs and increase efficiency.
|
||||
|
||||
## Supply Chain Visibility and Transparency
|
||||
|
||||
AI enables real-time tracking and monitoring of supply chain operations, providing enhanced visibility and transparency. By integrating data from various sources, such as sensors, IoT devices, and transportation systems, AI algorithms can identify bottlenecks, inefficiencies, and potential disruptions. This allows supply chain managers to make proactive decisions, improve delivery timelines, and effectively manage risks.
|
||||
|
||||
## Streamlined Logistics and Routing
|
||||
|
||||
Optimizing the movement of goods is a critical aspect of supply chain management. AI-powered algorithms analyze historical and real-time data, including traffic patterns, weather conditions, and customer preferences, to optimize delivery routes. This can result in cost savings, improved delivery times, reduced fuel consumption, and enhanced customer satisfaction. Additionally, AI can help automate last-mile delivery tasks through the use of autonomous vehicles or drones, further streamlining the logistics process.
|
||||
|
||||
## Supplier Relationship Management
|
||||
|
||||
Maintaining strong relationships with suppliers is vital for the success of any supply chain. AI can assist in supplier evaluation and selection by analyzing data like supplier performance, market conditions, and prices. By leveraging AI capabilities, businesses can automate supplier collaboration, monitor supplier quality, and proactively manage risks, ultimately improving the efficiency and reliability of their supply chain.
|
||||
|
||||
In conclusion, AI is revolutionizing supply chain optimization by leveraging advanced technologies to automate tasks, provide valuable insights, and enable more intelligent decision-making. From demand forecasting and intelligent warehouse management to supply chain visibility and streamlined logistics, AI is transforming the way businesses manage their supply chains. Embracing AI in supply chain optimization can lead to significant cost savings, increased operational efficiency, and improved customer satisfaction in today's hyper-competitive business landscape.
|
@ -1,27 +0,0 @@
|
||||
# How AI is Revolutionizing Telemedicine
|
||||
|
||||

|
||||
|
||||
In recent years, the ever-evolving field of artificial intelligence (AI) has found its way into numerous industries, including healthcare. One particular area where AI is making significant strides is telemedicine, revolutionizing the way healthcare is delivered remotely. By harnessing the power of AI, telemedicine has the potential to improve patient care, expand access to medical services, and enhance overall healthcare outcomes.
|
||||
|
||||
## Automated Triage and Diagnosis
|
||||
|
||||
AI-powered algorithms are being developed to assist healthcare providers in the triage process, where patients are sorted based on the severity of their condition. With the help of AI, telemedicine platforms can analyze a patient's symptoms, medical history, and vital signs faster and more accurately than ever before. By quickly identifying urgent cases, healthcare professionals can prioritize their attention, ultimately saving lives. AI-driven diagnostic tools are also being refined to provide accurate assessments remotely, minimizing the need for in-person visits in non-emergency situations.
|
||||
|
||||
## Virtual Assistants and Chatbots
|
||||
|
||||
The integration of AI-based virtual assistants and chatbots in telemedicine is transforming the way patients interact with healthcare providers. These intelligent systems can gather patients' medical histories, symptoms, and details of their concerns through conversational interfaces. By employing advanced natural language processing and machine learning, chatbots can offer initial medical advice, answer general health-related questions, and even schedule appointments. Virtual assistants powered by AI can provide 24/7 support, improving patient experience and easing the strain on healthcare professionals.
|
||||
|
||||
## Remote Monitoring and Predictive Analytics
|
||||
|
||||
AI technology enables continuous remote monitoring of patients, especially those with chronic conditions. Wearable devices and sensors collect real-time data, including heart rate, blood pressure, glucose levels, and activity patterns. AI algorithms analyze these streams of data to detect any abnormal patterns or potential health risks. By proactively identifying deviations from normal health parameters, healthcare providers can intervene early, preventing complications and enabling personalized treatment plans. Moreover, AI-powered predictive analytics can detect trends and patterns in large patient data sets, improving disease management strategies and advancing medical research.
|
||||
|
||||
## Enhanced Accessibility and Reduced Costs
|
||||
|
||||
Telemedicine equipped with AI offers enhanced accessibility to healthcare services, especially for those in remote areas or with limited mobility. AI-powered applications and platforms enable patients to consult with specialists from across the globe, overcoming geographical boundaries. Moreover, by replacing in-person consultations with virtual ones, telemedicine reduces the need for physical infrastructure, such as hospitals and clinics. This cost-saving aspect of telemedicine allows for more affordable healthcare options, benefiting both patients and healthcare systems.
|
||||
|
||||
## Ethical Considerations and Future Implications
|
||||
|
||||
While AI-powered telemedicine offers numerous benefits, it is essential to address ethical concerns. Patient privacy, data security, and the potential for biased algorithms are important aspects that need careful consideration. Transparent regulations and industry standards must be established to ensure the responsible use of AI in telemedicine. Furthermore, continuous advancements in AI technology, coupled with ongoing research, will play a crucial role in refining telemedicine practices and maximizing its potential.
|
||||
|
||||
As AI progresses, the impact on telemedicine will only expand further. From automating diagnosis and triage to virtual assistants and remote monitoring, AI is revolutionizing the way healthcare is delivered remotely. By harnessing the power of AI, telemedicine has the potential to improve patient outcomes, provide accessible healthcare options, and transform the future of medicine.
|
@ -1,27 +0,0 @@
|
||||
# How AI is Revolutionizing Traffic Management
|
||||
|
||||
Artificial Intelligence (AI) has emerged as a transformative force in various aspects of our lives. From healthcare to entertainment, AI is making a significant impact. One area where AI is proving to be revolutionary is traffic management. With the ever-increasing number of vehicles on the road, traditional traffic management systems are struggling to cope. However, AI offers a promising solution to this growing problem.
|
||||
|
||||
## Smart Traffic Control Systems
|
||||
|
||||
AI enables the creation of smart traffic control systems that can monitor and manage traffic flow in real-time. Traditional systems often rely on fixed timers and pre-determined schedules, leading to inefficient traffic management. In contrast, AI-based systems use a combination of sensors, cameras, and data analysis to dynamically respond to changing traffic conditions.
|
||||
|
||||
Using AI algorithms, these systems can optimize traffic signal timings based on real-time traffic flow data. By analyzing data from various sources such as CCTV cameras, GPS data, and mobile apps, AI can determine the most efficient traffic patterns for a given time and location. This dynamic approach ensures that traffic signals are adjusted according to current needs, reducing congestion and improving overall traffic flow.
|
||||
|
||||
## Predictive Traffic Analysis
|
||||
|
||||
AI also plays a vital role in predictive traffic analysis. By analyzing historical traffic data and considering various external factors such as weather, events, and construction work, AI algorithms can predict traffic patterns and congestion hotspots. This information allows traffic management authorities to proactively plan and implement measures to mitigate traffic problems.
|
||||
|
||||
Predictive analysis helps in optimizing traffic flow by suggesting alternate routes to drivers in congested areas. AI-powered navigation apps use real-time data to guide drivers through the least congested routes, ensuring a smoother commute. This not only saves time for individual drivers but also helps in reducing overall traffic congestion.
|
||||
|
||||
## Intelligent Transportation Systems
|
||||
|
||||
AI-driven Intelligent Transportation Systems (ITS) are transforming how traffic management is approached. These systems integrate AI algorithms, data analysis, and communication technologies to improve traffic safety, efficiency, and sustainability.
|
||||
|
||||
For instance, AI can identify traffic rule violations by analyzing data from surveillance cameras. This helps in ensuring compliance with traffic regulations and enhances overall road safety. Additionally, AI can analyze vehicle speed and patterns to identify potential accidents, enabling authorities to take proactive measures to prevent collisions.
|
||||
|
||||
Moreover, AI-powered ITS can integrate with public transportation systems, facilitating efficient and reliable transit services. By optimizing routes and schedules based on real-time data, AI ensures that public transportation is more responsive to the needs of commuters, reducing congestion on the roads.
|
||||
|
||||
## Conclusion
|
||||
|
||||
AI is revolutionizing traffic management by introducing smart and dynamic systems that optimize traffic flow and improve road safety. Through real-time monitoring, predictive analysis, and intelligent transportation systems, AI provides innovative solutions to the challenges faced by traditional traffic management methods. As technology advances, we can expect AI to play an increasingly prominent role in tackling traffic congestion, making our daily commutes smoother and more efficient.
|
@ -1,25 +0,0 @@
|
||||
# How AI is Revolutionizing Virtual Reality (VR)
|
||||
|
||||
Virtual Reality (VR) is a technology that has been steadily evolving and capturing the imagination of people worldwide. From entertainment and gaming to education and healthcare, VR offers users an immersive and interactive experience that transports them to virtual environments. As VR continues to advance, one of the key driving forces behind its growth is the integration of Artificial Intelligence (AI).
|
||||
|
||||
AI is paving the way for new possibilities in VR by enhancing user experience, improving realism, and enabling intelligent and adaptive interactions within virtual worlds. Here are some of the ways AI is revolutionizing the world of VR:
|
||||
|
||||
## Enhanced User Experience
|
||||
AI algorithms have the ability to analyze and interpret data collected from VR users to personalize their experiences. By understanding user preferences, AI algorithms can optimize visual and auditory stimuli, creating tailored and immersive experiences. For example, AI can adapt the virtual environment based on a user's emotions, gaze, or even biofeedback to enhance engagement and overall satisfaction.
|
||||
|
||||
## Real-Time Adaptation
|
||||
Traditionally, VR experiences were static and pre-defined, limiting interactions and reducing realism. However, with AI, VR can dynamically respond and adapt to user behavior in real-time. AI algorithms can process user inputs and adjust the virtual environment accordingly, creating a more engaging and interactive experience. This real-time adaptation allows VR experiences to become more intuitive and responsive, amplifying the feeling of presence and immersion.
|
||||
|
||||
## Natural Language Processing (NLP)
|
||||
Integrating NLP with VR opens up new possibilities for communication and interaction within virtual environments. AI-powered speech recognition and synthesis technologies enable users to interact with virtual worlds through voice commands or conversations. This capability enhances the realism of social VR experiences, making interactions with AI-controlled virtual characters more natural and immersive.
|
||||
|
||||
## Intelligent Avatars and Characters
|
||||
AI plays a crucial role in shaping virtual characters in VR. By employing machine learning algorithms, AI can create intelligent avatars that behave and interact realistically with users. These virtual characters can adapt their behavior based on context, learn from user interactions, and simulate emotions and social cues. AI-driven characters enhance the social aspects of VR experiences, making interactions with virtual beings more dynamic and lifelike.
|
||||
|
||||
## Improved Training and Education
|
||||
AI-powered VR enables immersive training and education environments that simulate real-world scenarios. By combining VR with AI algorithms, users can experience realistic simulations with intelligent feedback. For example, in medical training, AI can recognize and evaluate a trainee's actions, providing immediate feedback and guidance. This combination of VR and AI offers a safe and cost-effective way to practice and refine skills in various fields.
|
||||
|
||||
## Behavioral Analysis and Predictive Modeling
|
||||
AI algorithms can analyze user behavior within VR environments, providing valuable insights for designers and developers. By studying user interactions, AI can identify patterns, preferences, and areas for improvement, helping to optimize VR experiences. Additionally, AI can generate predictive models based on user data, allowing developers to create personalized VR content tailored to individual users.
|
||||
|
||||
In conclusion, the integration of AI into VR is revolutionizing the way we experience virtual environments. By leveraging AI algorithms, VR experiences can become more immersive, adaptive, and interactive. As AI technology continues to advance, we can expect even more exciting developments in the realm of VR, further blurring the lines between the virtual and real world.
|
@ -1,31 +0,0 @@
|
||||
# How AI is Revolutionizing Voice-Activated Assistants
|
||||
|
||||
Artificial Intelligence (AI) has made significant advancements in recent years, revolutionizing various industries. One area where AI has had a profound impact is voice-activated assistants. These intelligent virtual helpers, such as Siri, Alexa, and Google Assistant, have become an integral part of our daily lives, and AI is the key driving force behind their success.
|
||||
|
||||
## Natural Language Processing
|
||||
|
||||
AI-powered voice-activated assistants have evolved thanks to advancements in Natural Language Processing (NLP). NLP enables machines to understand and interpret human language, allowing assistants to process voice commands and respond in a conversational manner. As a result, these assistants have become more accessible and user-friendly, making day-to-day tasks easier and more efficient.
|
||||
|
||||
## Personalized User Experience
|
||||
|
||||
AI-driven voice-activated assistants excel in providing personalized user experiences. By analyzing user preferences, behavior, and historical data, these assistants can adapt to individual needs and preferences. They can learn from user interactions and make suggestions accordingly. Whether it's recommending personalized playlists, recommending nearby restaurants, or suggesting relevant news articles, AI helps these assistants understand user preferences and provide tailored responses.
|
||||
|
||||
## Integration with Smart Home Devices
|
||||
|
||||
Voice-activated assistants have seamlessly integrated with a range of smart home devices, thanks to AI capabilities. Users can control IoT devices like thermostats, lighting systems, and security cameras simply by using voice commands. AI ensures that these assistants can understand the user's intent, accurately interpret their commands, and execute them flawlessly. This integration has transformed the way we interact with our homes, offering convenience, energy efficiency, and enhanced security.
|
||||
|
||||
## Natural and Intuitive Conversations
|
||||
|
||||
AI has played a pivotal role in improving the conversational abilities of voice-activated assistants. Earlier versions of these assistants often struggled with understanding complex queries or context-dependent follow-up questions. However, AI advancements have made it possible for virtual assistants to engage in more natural and intuitive conversations. They can now handle ambiguous queries, understand context, and provide accurate responses, making the interaction with users feel more human-like.
|
||||
|
||||
## Hands-free Accessibility
|
||||
|
||||
Voice-activated assistants have significantly enhanced accessibility for individuals with physical disabilities or limitations. AI enables these virtual assistants to understand and respond to voice commands, empowering people to perform tasks without needing to physically operate devices. From making phone calls to sending messages and controlling smart devices, AI-powered assistants have become indispensable tools, ensuring equal access to information and services for everyone.
|
||||
|
||||
## Continual Improvement and Learning
|
||||
|
||||
One of the key strengths of AI is its ability to continually learn and improve over time. Voice-activated assistants utilize machine learning algorithms to analyze vast amounts of data, allowing them to enhance their accuracy and performance continually. They can adapt to new accents, dialects, and even languages, ensuring that their communication capabilities evolve alongside user needs. With each interaction, these assistants become smarter and more capable, providing users with an ever-improving experience.
|
||||
|
||||
## Conclusion
|
||||
|
||||
AI has revolutionized voice-activated assistants, transforming them from simple voice-activated command systems to sophisticated, intelligent helpers. From the way we interact with our homes to personalized recommendations and hands-free accessibility, AI has made these assistants an integral part of our daily lives. As technology continues to advance, we can expect AI-powered voice-activated assistants to become even more intuitive, efficient, and seamlessly integrated into our various devices and environments.
|
@ -1,31 +0,0 @@
|
||||
# AI Revolutionizing Waste Management
|
||||
|
||||
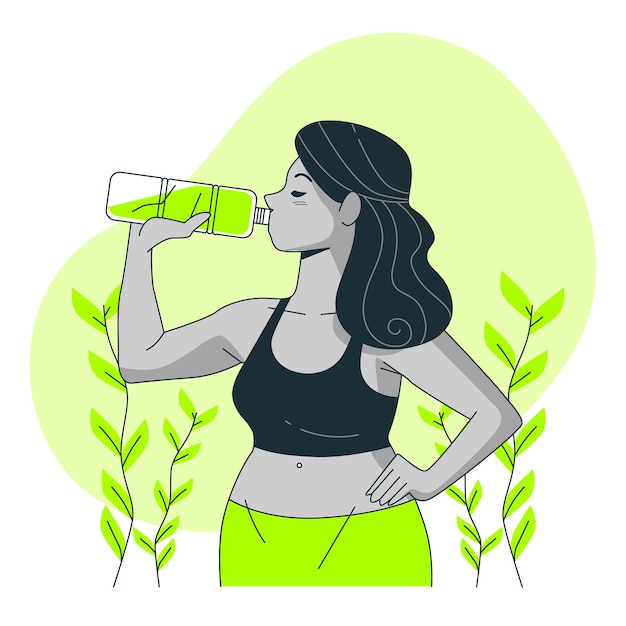
|
||||
|
||||
Waste management is an ever-growing concern, with cities and nations struggling to handle the increasing amounts of waste generated daily. However, emerging technologies like artificial intelligence (AI) are making significant strides in revolutionizing waste management practices. AI is reshaping how waste is identified, sorted, and managed, offering tremendous benefits for both the environment and human lives.
|
||||
|
||||
## Efficient Waste Sorting
|
||||
|
||||
One of the primary challenges in waste management is the proper sorting and disposal of different types of waste. AI-powered systems are transforming this process, making it faster and more accurate. Computer vision technology, a branch of AI, enables waste sorting robots to analyze and categorize waste materials quickly. These robots use optical sensors and cameras to identify and sort recyclable materials such as plastics, paper, and metals from non-recyclables.
|
||||
|
||||
By automating waste sorting, AI not only increases efficiency and precision but also reduces the burden on human workers who would typically be involved in manual sorting. This technology ensures that recyclable materials are redirected to recycling facilities, significantly reducing the amount of waste that ends up in landfills or incinerators.
|
||||
|
||||
## Prediction and Optimization
|
||||
|
||||
Another significant impact of AI in waste management lies in its ability to predict waste generation patterns. By analyzing historical data and various factors like population density, weather conditions, and social behaviors, AI algorithms can estimate future waste production accurately. This predictive capability enables waste management authorities to optimize their collection and disposal strategies, ensuring resources are utilized efficiently.
|
||||
|
||||
AI algorithms can determine the optimal collection routes, schedules, and the number of waste collection vehicles needed based on real-time data. By reducing unnecessary pickups and optimizing routes, AI helps minimize fuel consumption, lowers carbon emissions, and reduces traffic congestion in cities.
|
||||
|
||||
## Smarter Recycling
|
||||
|
||||
Recycling plays a crucial role in waste management. AI and machine learning algorithms are revolutionizing the recycling industry, making it more efficient and effective. These techniques enable waste management facilities to identify and separate different types of recyclable materials better. For instance, AI-powered sensors can rapidly identify and sort various plastic types, ensuring they can be recycled efficiently.
|
||||
|
||||
Moreover, AI algorithms can assist in developing more sustainable and eco-friendly packaging materials. By analyzing data on material properties, manufacturing techniques, and environmental impact, AI can help scientists and manufacturers create packaging that is easier to recycle and has a reduced environmental footprint.
|
||||
|
||||
## Waste-to-Energy Conversion
|
||||
|
||||
AI is also contributing to waste-to-energy (WtE) conversion systems, which convert non-recyclable waste into usable energy. By using AI algorithms to optimize WtE processes, it becomes possible to extract more energy efficiently, thereby reducing reliance on traditional fossil fuels. AI can help predict optimal energy generation levels, reduce emissions, and minimize residual waste produced during the process.
|
||||
|
||||
## Conclusion
|
||||
|
||||
Artificial intelligence is undeniably revolutionizing waste management across the globe. Whether it is efficient waste sorting, predictive optimization, smarter recycling, or waste-to-energy conversion, AI technologies are proving to be powerful tools. By combining AI's capabilities with human efforts, we can pave the way for a sustainable and cleaner future. The continued development and integration of AI in waste management will undoubtedly lead to a more efficient and environmentally friendly approach to handling waste.
|
@ -1,37 +0,0 @@
|
||||
# How Artificial Intelligence is Revolutionizing Weather Forecasting
|
||||
|
||||

|
||||
|
||||
Weather forecasting has always been a complex task, with meteorologists analyzing vast amounts of data and using advanced models to predict future weather conditions. However, with the recent advancement in artificial intelligence (AI), weather forecasting has taken a giant leap forward. AI, with its ability to analyze data quickly and make accurate predictions, is transforming the way we understand and forecast the weather.
|
||||
|
||||
## Predictive Analysis with AI
|
||||
|
||||
AI-powered weather forecasting relies on the analysis of massive amounts of data collected from various sources, such as weather stations, satellites, airplanes, and even social media. Machine learning algorithms are employed to process and understand this data, allowing meteorologists to make more accurate predictions.
|
||||
|
||||
One of the key advantages of AI in weather forecasting is its ability to analyze historical weather patterns and compare them to real-time data. By identifying similarities and patterns, AI algorithms can predict future weather conditions with a higher degree of accuracy. This predictive analysis can be particularly useful in identifying potentially dangerous weather events, such as hurricanes, tornadoes, or heavy storms, thereby enabling better preparedness and response efforts.
|
||||
|
||||
## Improving Model Accuracy
|
||||
|
||||
Traditional weather forecasting models rely on numerous equations and assumptions that have been developed over the years. While these models have proven to be reasonably accurate, they still face challenges when it comes to capturing the complexity of weather systems. AI can help improve model accuracy by integrating data-driven approaches.
|
||||
|
||||
By feeding vast amounts of weather data into AI models, they can learn to identify patterns and relationships that are difficult for traditional models to capture. AI algorithms can detect subtle interactions between various atmospheric variables, leading to more precise predictions. This fine-grained analysis enables better identification and tracking of weather patterns, resulting in improved forecast accuracy.
|
||||
|
||||
## Faster and More Timely Predictions
|
||||
|
||||
In addition to accuracy, AI can significantly improve the speed and timeliness of weather predictions. Traditional forecasting models are computationally intensive and can take hours or even days to generate forecasts. AI-powered systems, on the other hand, can process vast amounts of data and generate near-real-time predictions, allowing meteorologists to respond quickly to rapidly changing weather conditions.
|
||||
|
||||
Enhancing the speed of weather predictions is crucial for various sectors, including aviation, agriculture, and disaster management. With AI, airports can plan flight schedules better, farmers can make informed decisions about planting and harvesting, and emergency responders can mobilize resources more effectively.
|
||||
|
||||
## Personalized and Hyperlocal Forecasts
|
||||
|
||||
AI-powered weather forecasting systems have the potential to provide personalized and hyperlocal forecasts. By analyzing individual user data, such as location and preferences, AI algorithms can tailor weather forecasts according to specific needs. This level of customization allows individuals to plan their activities better, whether it's a morning jog, a picnic, or a construction project.
|
||||
|
||||
Moreover, AI can offer hyperlocal forecasts by analyzing the minute variations in weather patterns across different regions of a city or even within a neighborhood. This level of granularity ensures that people receive accurate and localized weather information for their immediate surroundings, enabling them to make informed decisions based on real-time conditions.
|
||||
|
||||
## Challenges and Future Prospects
|
||||
|
||||
While AI has transformed weather forecasting, there are still challenges to overcome. Ensuring the reliability and accuracy of AI models is paramount, as incorrect predictions can have significant consequences. The integration of AI with human expertise is essential to validate and interpret the results generated by AI algorithms.
|
||||
|
||||
In the future, AI can further enhance weather forecasting by incorporating data from additional sources, such as smart sensors, internet of things (IoT) devices, and even drones. Advanced predictive modeling techniques can be utilized to identify the impact of climate change on long-term weather patterns.
|
||||
|
||||
Overall, AI has revolutionized weather forecasting by providing faster, more accurate, and personalized predictions. As technology continues to advance, the potential for AI to improve our understanding of weather patterns and their implications for our daily lives becomes even more exciting.
|
@ -1,21 +0,0 @@
|
||||
Decision Trees
|
||||
Support Vector Machines (SVM)
|
||||
Neural Networks
|
||||
Naïve Bayes
|
||||
k-Nearest Neighbors (k-NN)
|
||||
Gradient Boosting Machines (GBM)
|
||||
Random Forests
|
||||
DBSCAN
|
||||
Gaussian Mixture Models (GMM)
|
||||
Principal Component Analysis (PCA)
|
||||
Independent Component Analysis (ICA)
|
||||
t-SNE
|
||||
Latent Dirichlet Allocation (LDA)
|
||||
Association Rules (Apriori, FP-Growth)
|
||||
Policy Gradients
|
||||
Actor-Critic Methods
|
||||
Proximal Policy Optimization (PPO)
|
||||
Monte Carlo Tree Search (MCTS)
|
||||
SARSA
|
||||
Temporal Difference Learning (TD Learning)
|
||||
Trust Region Policy Optimization (TRPO)
|
Loading…
Reference in New Issue
Block a user